Scalable Semi-Supervised Learning through Combined Anchor-based Graph and Flexible Manifold Embedding.
2023 International Conference on Computer and Applications (ICCA)(2023)
摘要
This paper focuses on graph-based semi-supervised learning, specifically for large-scale graphs used in inductive multi-class classification. The proposed method aims to overcome limitations in current scalable graph-based semi-supervised learning techniques. The key innovation is integrating the anchor graph calculation into the learning model, rather than treating it as a separate, offline step. This approach involves several essential tasks, including simultaneously estimating unlabeled samples, mapping the feature space to the label space, creating an affinity matrix for the anchor graph, and using labels and features associated with anchor points to construct the graph. The experimental results, conducted with large datasets, demonstrate a positive trend, showing higher accuracy and greater stability compared to existing scalable graph-based semi-supervised learning methods.
更多查看译文
关键词
Graph-based semi-supervised learning,large databases,anchor-to-anchor graph,label propagation,unified framework
AI 理解论文
溯源树
样例
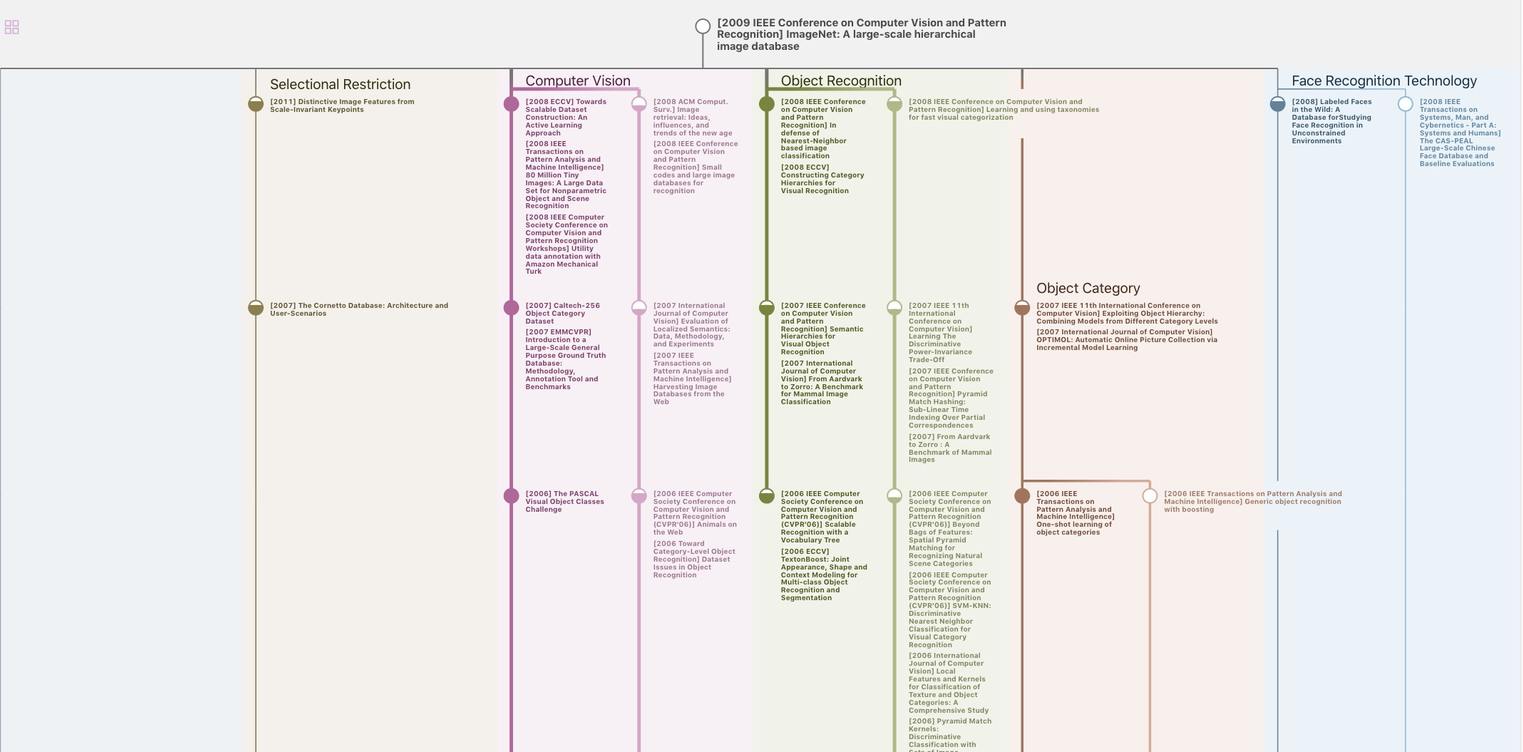
生成溯源树,研究论文发展脉络
Chat Paper
正在生成论文摘要