Data-driven autoencoder neural network for onboard BMS Lithium-ion battery degradation prediction
Journal of Energy Storage(2024)
摘要
An autoencoder based neural network architecture, CD-Net, is proposed to predict Lithium-ion battery capacity degradation as a function of operation time as part of a battery management system. CD-Net's generalization performance on various LIB cell chemistry is tested. The incorporation of cell chemistry in CD-Net leads to an improvement in the overall battery capacity prediction accuracy of >2 % for LiNiMnCoO2 cells, >5 % for LiNiCoAlO2 cells, and >12 % for LiFePO4 cells when compared to the similar ML models that do not incorporate cell chemistry information. A comparison of onboard battery health prediction using CD-Net against support vector regression, Bayesian regression, and Gaussian process regression-based approaches shows that CD-Net has higher computational efficiency with <2 % of relative remaining useful life (RUL) prediction error in a no-cell chemistry information setting. In summary, our work presents a chemistry-independent neural network model tailored specifically for onboard BMS applications, showcasing notable predictive capabilities in the context of Lithium-ion battery health assessment.
更多查看译文
关键词
BMS,BR,EOL,GPR,CD-Net,LFP,LIB,MAE,ML,MSE,NCA,NMC,RUL,SNL,SoC,SoH,SVR
AI 理解论文
溯源树
样例
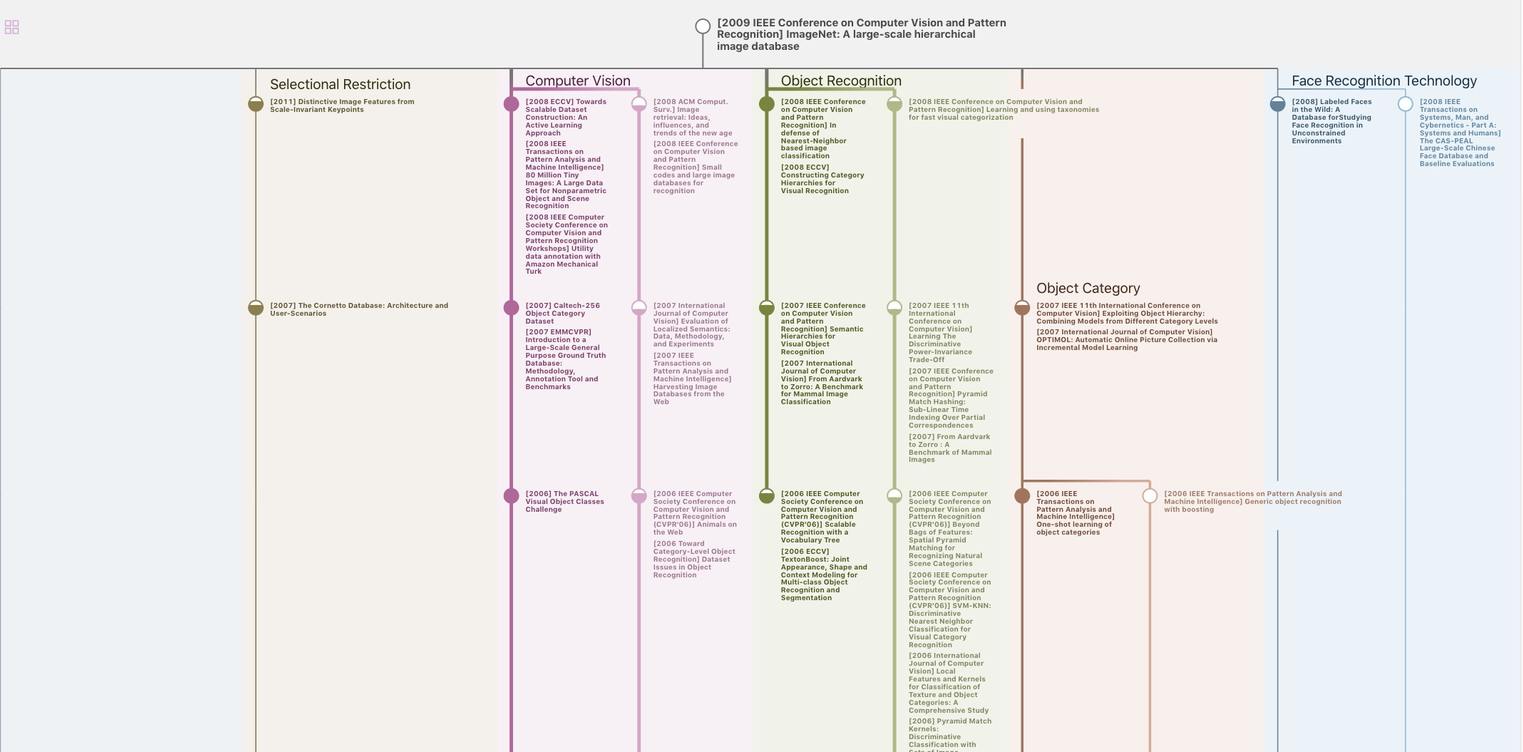
生成溯源树,研究论文发展脉络
Chat Paper
正在生成论文摘要