Instance-Aware Monocular 3D Semantic Scene Completion
IEEE TRANSACTIONS ON INTELLIGENT TRANSPORTATION SYSTEMS(2024)
摘要
We study outdoor 3D scene understanding, a challenging task demanding the intelligent system to infer both geometry and semantics from a single-view image - a critical skill for autonomous vehicles to navigate in the real 3D world. Towards this end, we present an instance-aware monocular semantic scene completion framework. To the best of our knowledge, this is the first endeavor specifically targeting the challenge of instance perception in the camera-based semantic scene completion task. Our method consists of two stages. In stage I, we design a region-based VQ-VAE network, providing an effective solution for 3D occupancy prediction. In stage II, we first introduce an instance-aware attention module, explicitly incorporating instance-level cues captured from mask images to enhance the instance features in RGB images. Then we leverage the deformable cross-attention to aggregate image features corresponding to each voxel query and utilize the deformable self-attention to refine query proposals. We combine these key ingredients and evaluate our method on two challenging datasets, namely SemanticKITTI and SSCBench-KITTI-360. The results unequivocally demonstrate the superiority of our proposed method over the state-of-the-art VoxFormer-S. Specifically, our method surpasses VoxFormer-S by 0.22 IoU and 0.72 mIoU on the validation set and achieves an impressive improvement of 3.04 IoU and 1.06 mIoU on the SSCBench-KITTI-360 validation set. Meanwhile, our approach ensures accurate perception of critical instances, thereby exhibiting its exceptional performance and potential for practical deployment.
更多查看译文
关键词
3D scene understanding,semantic scene completion,3D vision
AI 理解论文
溯源树
样例
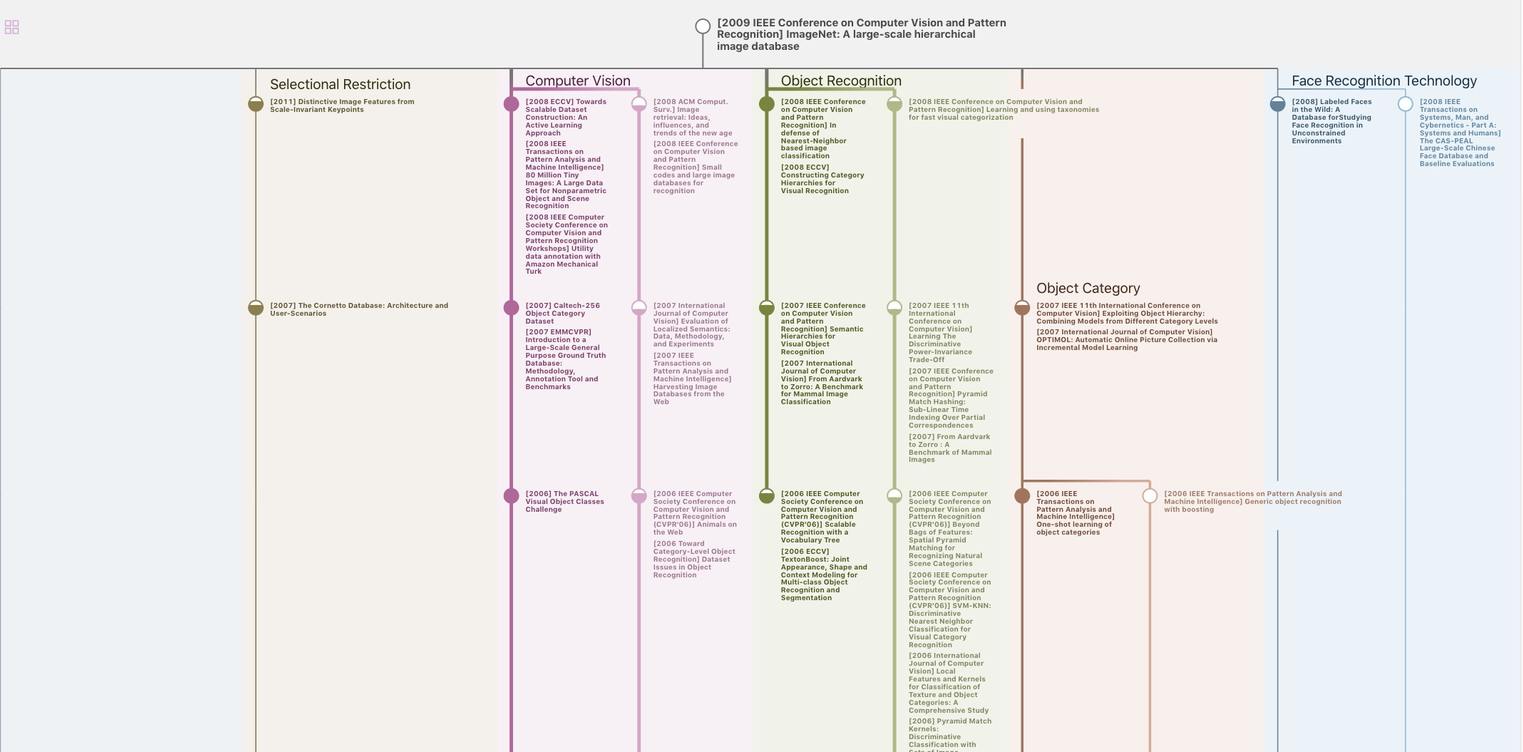
生成溯源树,研究论文发展脉络
Chat Paper
正在生成论文摘要