Dual Weighted-Class Adversarial Network for Rotary Machine Fault Diagnosis Using Multisource Domain with Class-Inconsistent Data
IEEE-ASME TRANSACTIONS ON MECHATRONICS(2024)
摘要
Most existing transfer fault diagnosis methods have gained noteworthy domain adaptability and generalization capability for rotating machinery under the scenario where source and target have identical label space. Nevertheless, inconsistent fault modes that occur under different operating conditions in an actual industrial environment can make it challenging to construct a transfer fault diagnosis model across domains since domain shift and category inconsistencies. To address this issue, a dual weighted-class adversarial network (DWCAN) is proposed for rotary machine fault diagnosis using multisource domain with class-inconsistent data. First, the model consists of a common feature extractor, weighted intraclass and interclass adversarial discriminator, and classifier. Then, based on the intraclass and interclass adversarial strategy, the DWCAN method learns domain invariant fault representations across domains by narrowing domain and class inconsistencies and achieves intraclass compactness and interclass separability from the aspect of local class alignment. Lastly, two experimental datasets are adopted to demonstrate the promising performance of DWCAN compared with other methods.
更多查看译文
关键词
Class inconsistent,dual weighted-class adversarial network (DWCAN),fault diagnosis,multisource domain,rotary machine
AI 理解论文
溯源树
样例
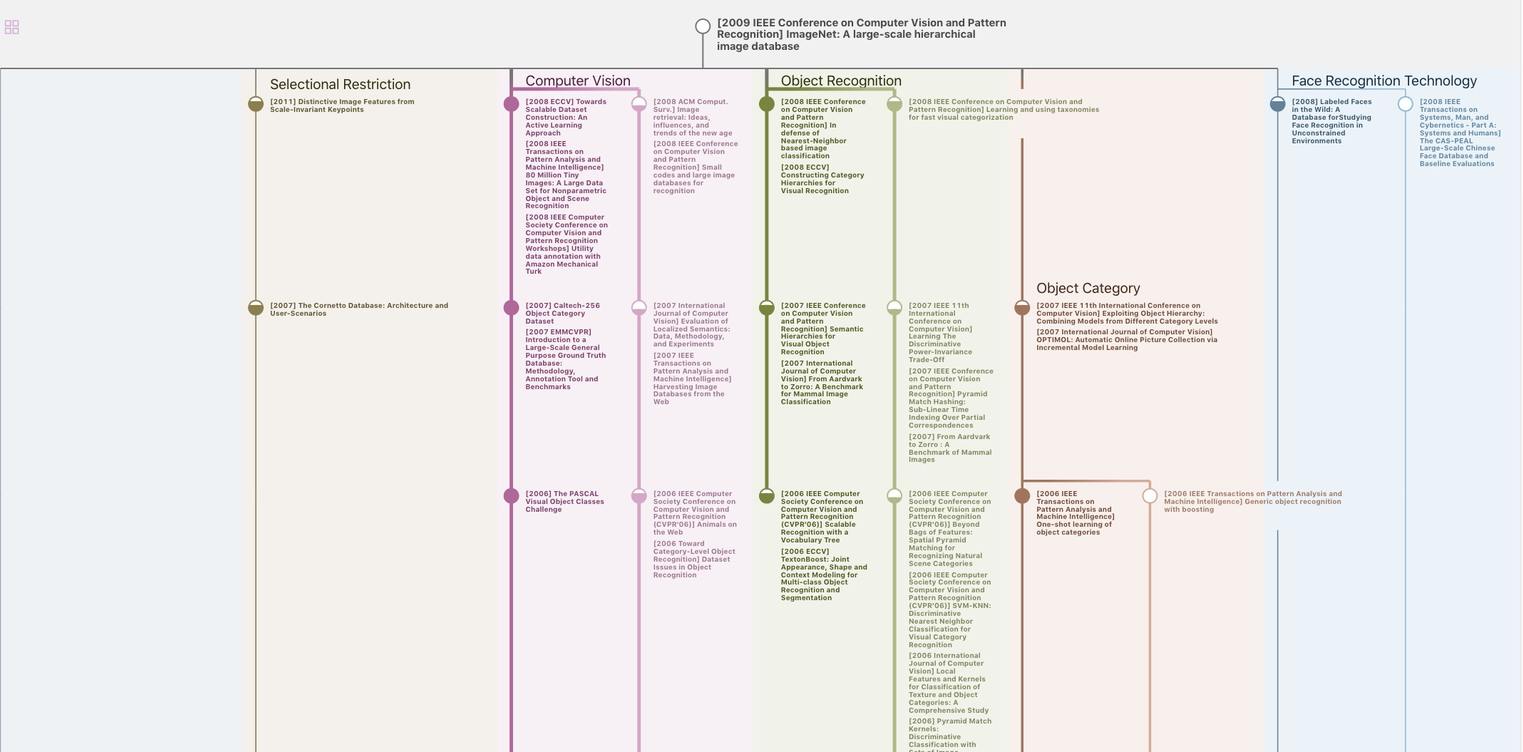
生成溯源树,研究论文发展脉络
Chat Paper
正在生成论文摘要