Multi-Step Regression Network With Attention Fusion for Airport Delay Prediction
IEEE TRANSACTIONS ON INTELLIGENT TRANSPORTATION SYSTEMS(2024)
摘要
As part of airport behavior decisions, the accurate prediction of airport delay is highly significant in optimizing flight takeoff and landing sequences. However, the combination of various influencing factors affects airport delay prediction strongly, which would bring severe challenges in prediction. This paper introduces the sequence-to-sequence network and proposes a multi-step regression prediction method for the airport delay (DA-BILSTM) to accurately predict the airport delay. Rather than only considering a single kind of airport delay influencing factors, we design an attention fusion network for learning the sequence and condition correlation features adaptively. Moreover, the Bayesian optimization algorithm is introduced to optimize DA-BILSTM's hyperparameters. The method is applied individually to two datasets for predicting the airport's delays. The experiment results show that the prediction performance of DA-BILSTM is better than many state-of-the-art methods including the autoregressive integrated moving average model (ARIMA), long short-term memory (LSTM), gated recurrent unit(GRU), CNN-BILSTM, and TS-LSTM. When using DA-BILSTM in the two datasets, the average MAE of airport delay prediction in the next 5 hours is about 10 minutes, and the average RMSE is 20 minutes.
更多查看译文
关键词
Airports,Delays,Atmospheric modeling,Correlation,Predictive models,Meteorology,Indexes,Airport delay prediction,attention fusion network,bidirectional long short-term memory network,sequence to sequence
AI 理解论文
溯源树
样例
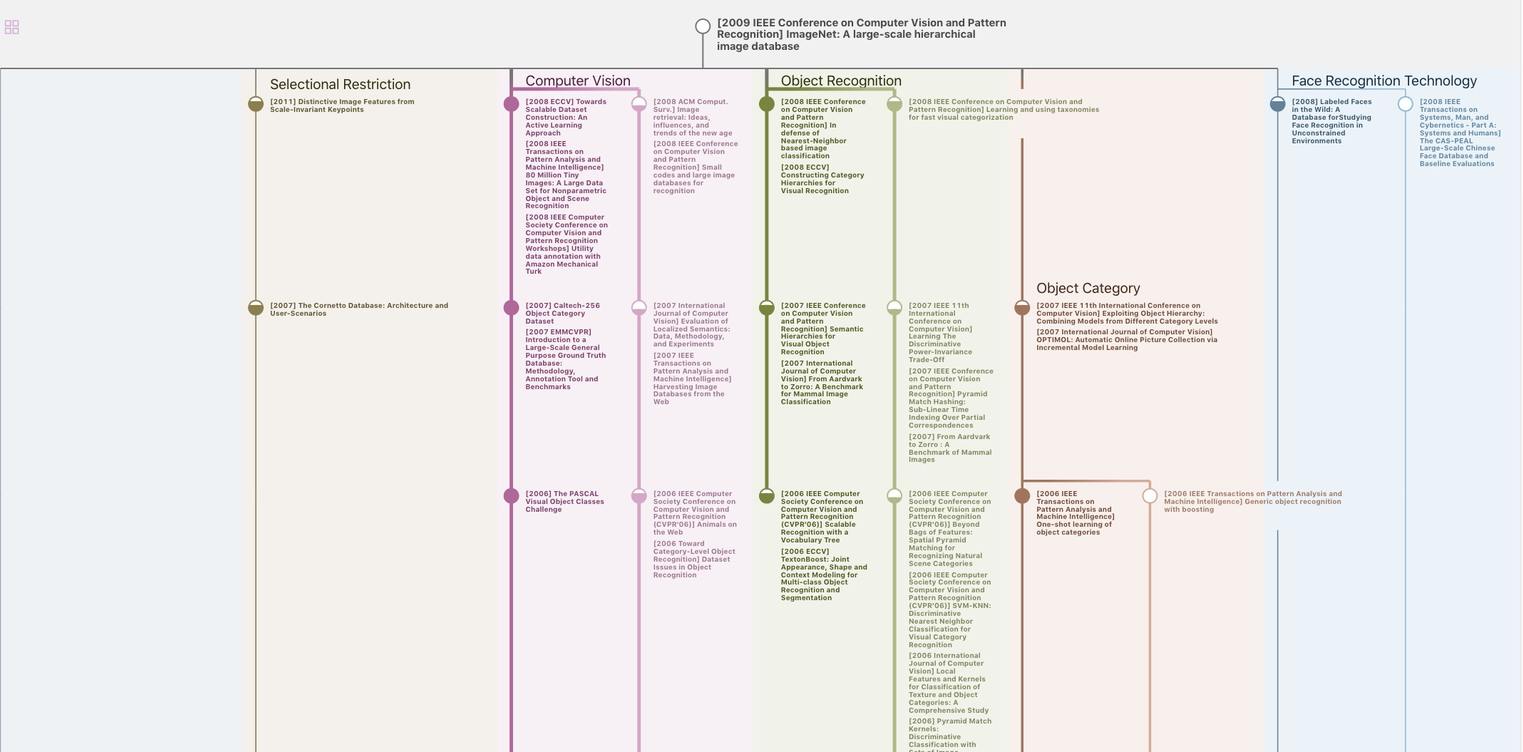
生成溯源树,研究论文发展脉络
Chat Paper
正在生成论文摘要