FPGA-Accelerated Sim-to-Real Control Policy Learning for Robotic Arms.
IEEE Trans. Circuits Syst. II Express Briefs(2024)
摘要
Sim-to-real robot learning has been used in various applications, but its implementation in software may not provide the best performance. This tutorial describes how hardware acceleration based on Field-Programmable Gate Array (FPGA) technology for deep reinforcement learning can improve sim-to-real robot control policy learning. A novel architecture for the Deep Deterministic Policy Gradient (DDPG) algorithm is developed for a full-stack sim-to-real development platform to learn control policies for robotic arms. The capability of our development platform is illustrated by transferring learned policies encoded as fixed-point numbers from our implementation to a miniature robotic arm.
更多查看译文
关键词
FPGA,acceleration,robotic arms,sim-to-real,robot learning,reinforcement learning
AI 理解论文
溯源树
样例
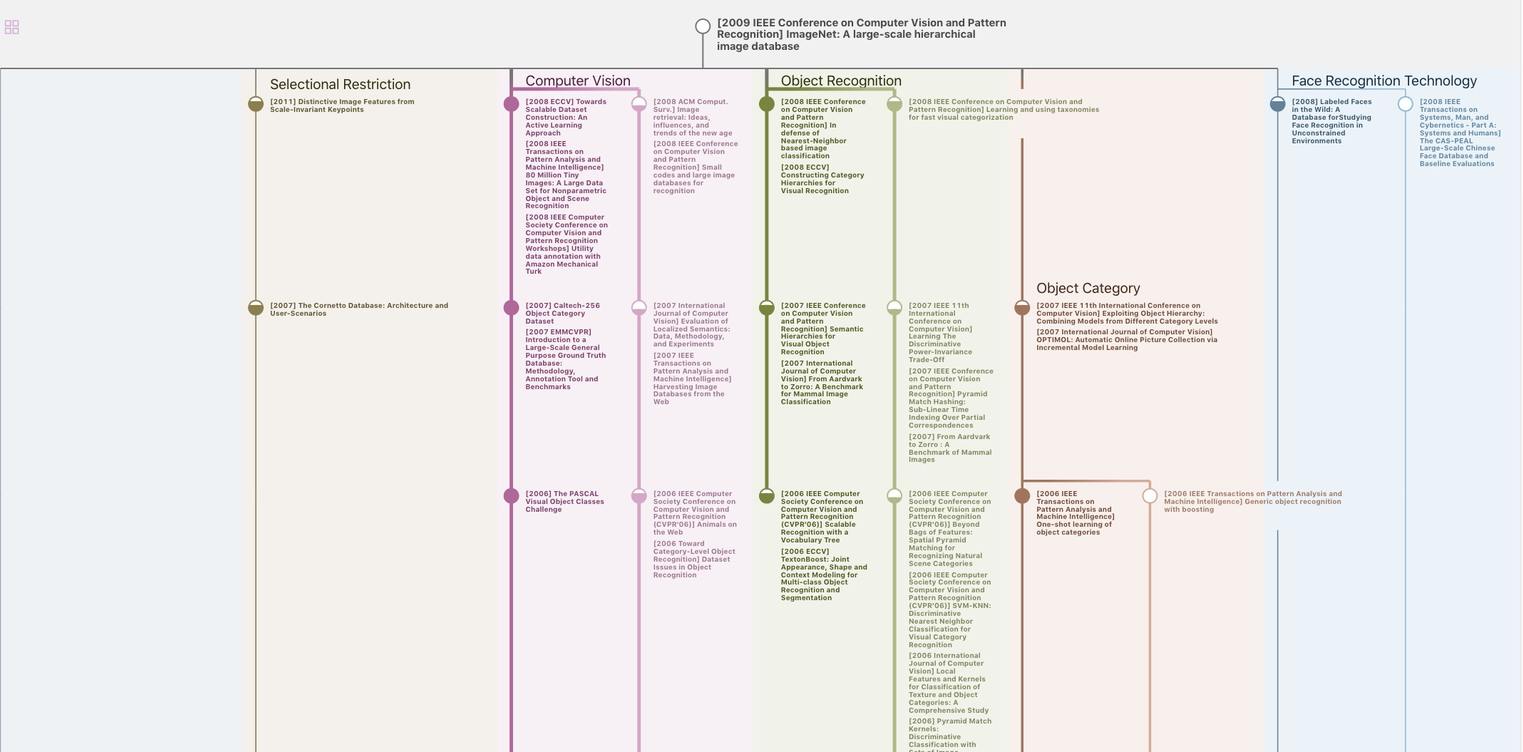
生成溯源树,研究论文发展脉络
Chat Paper
正在生成论文摘要