Kinematic and Dynamic Manipulability Optimizations of Redundant Manipulators Based on RNN Model
IEEE TRANSACTIONS ON INDUSTRIAL INFORMATICS(2023)
摘要
Aiming at the trajectory planning problem considering kinematic and dynamic manipulability optimizations, this article proposes an acceleration level manipulability maximization (ALMM) scheme to solve the problem in acceleration level for the first time. The manipulability index with nonlinear characteristics is reconstructed in acceleration level by a novel multilevel simultaneous processing strategy. The minimum joint velocity index is introduced to ensure the convergence of the system and maintain the velocity at a low level. Subsequently, the ALMM scheme, which includes pose maintenance, manipulability optimization, joint velocity minimization, and joint physical limit avoidance, is constructed and further formulated as a unified quadratic program. Then, a recurrent neural network with rigorously provable convergence is designed for the ALMM method. Simulations and physical experiments illustrate that the ALMM scheme can accomplish the manipulability optimization task excellently. Comparisons further verify the superiority of this scheme.
更多查看译文
关键词
Kinematic control,manipulability optimization,recurrent neural network (RNN),redundancy resolution
AI 理解论文
溯源树
样例
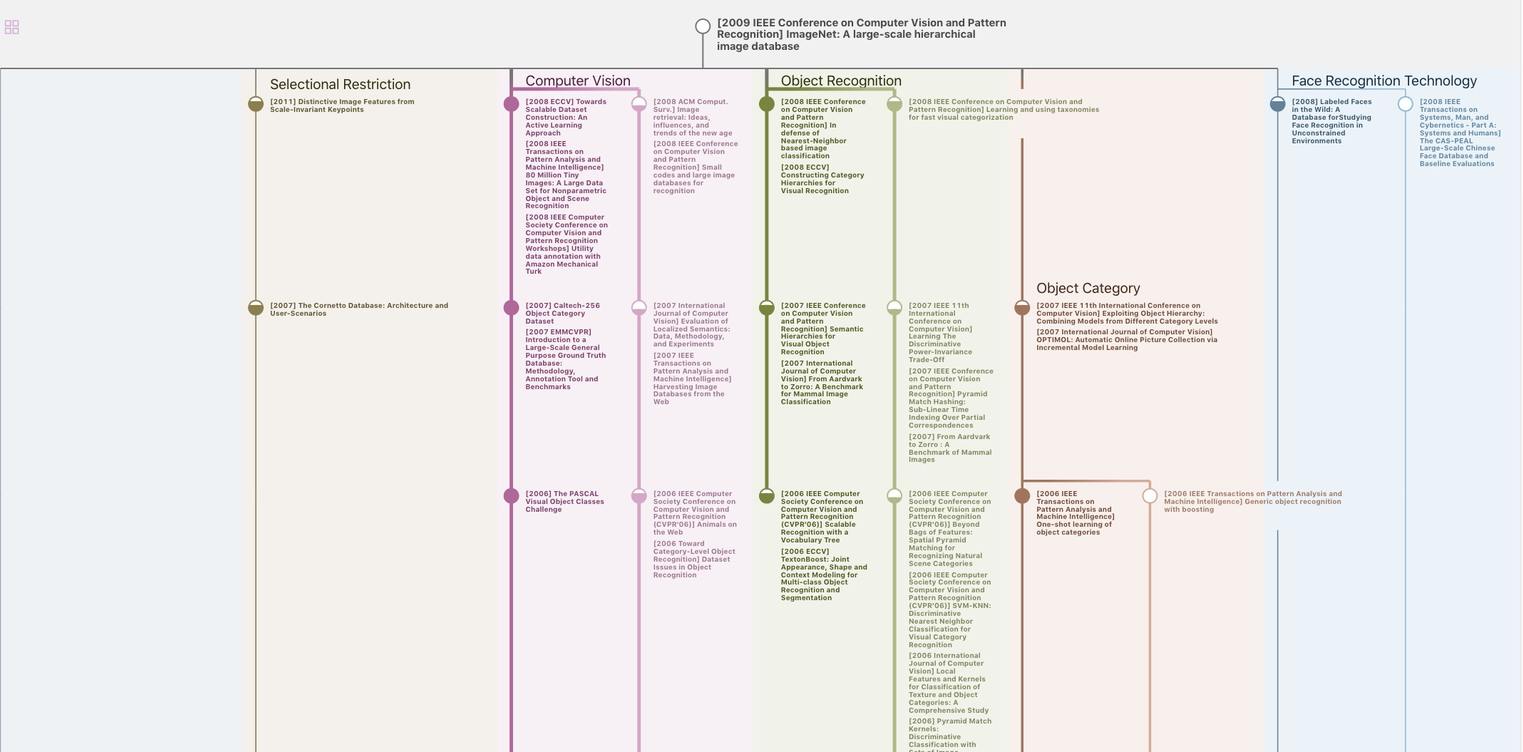
生成溯源树,研究论文发展脉络
Chat Paper
正在生成论文摘要