Toggle Rate Aware Quantization Model Based on Digital Floating-Point Computing-in-Memory Architecture
IEEE Transactions on Circuits and Systems II: Express Briefs(2024)
摘要
Computing-in-memory (CIM) has been proven to achieve high energy efficiency and significant acceleration effects on neural networks with high computational parallelism. Based on typical integer CIMs, some floating-point CIMs (FP-CIM) are proposed recently to execute more accuracy-demanding tasks such as training and high-precision inference. However, prior research has not adequately explored the relationship between circuit design within the FP-CIM architecture and hardware/software metrics. Furthermore, in digital circuits, the data toggle rate significantly affect hardware performance. In this brief, a toggle rate-aware quantization model is proposed to define and explore the design space of FP-CIM. Based on the experimental results, some key considerations on FP-CIM design are derived. With the toggle rate reduction scheme, toggle rate can be reduced by 28%, resulting in a remarkable 1.18x improvement in energy efficiency with only a 0.35% accuracy loss. To validate our model, a 28nm digital FP-CIM test chip is fabricated which achieves energy efficiency of 32.28 TFLOPS/W and inference accuracy of 76.14% on DenseNet161 and ImageNet dataset.
更多查看译文
关键词
Quantization,Toggle rate,Floating-point,Computing-in-memory
AI 理解论文
溯源树
样例
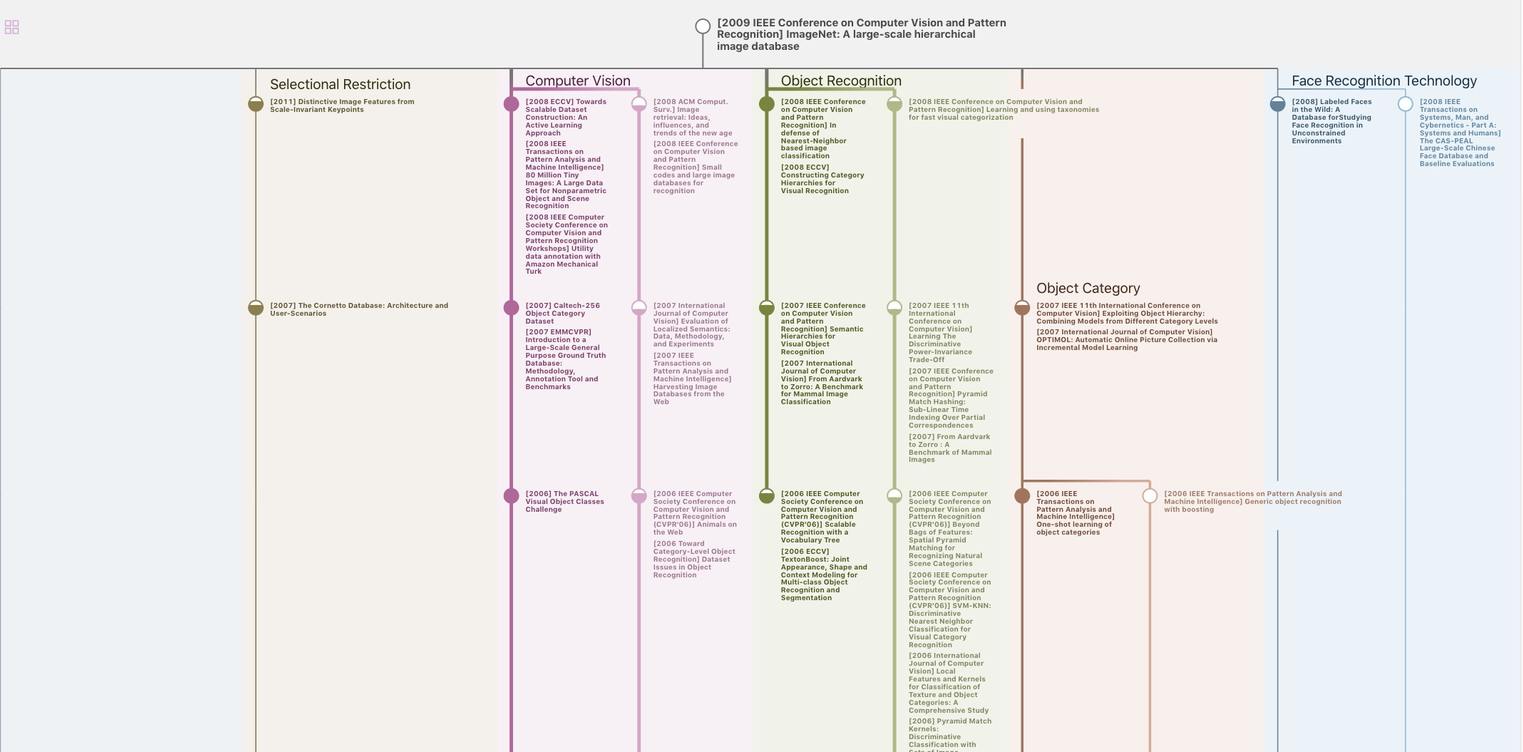
生成溯源树,研究论文发展脉络
Chat Paper
正在生成论文摘要