Graph Structure Change-Based Anomaly Detection in Multivariate Time Series of Industrial Processes
IEEE TRANSACTIONS ON INDUSTRIAL INFORMATICS(2024)
摘要
Multivariate time series anomaly detection plays an important role for the safe operation of industrial devices and systems. At present, many effective methods have the major limitation that the changes in information propagation between variables are not considered when anomalies occur. Therefore, this article proposes a novel graph structure change-based anomaly detection on multivariate time series (GSC-MAD). First, a stable graph structure under normal conditions is obtained and a single-step prediction for all variables is achieved from a high-dimensional time-series embedding representation learned from the normal data. Then, anomaly detection is achieved by combining the variable behavior deviation reflected by prediction errors and the information propagation deviation between variables reflected by GSC. Extensive experiments on five real-world benchmarks are conducted to demonstrate the effectiveness of the proposed method and compared with current state-of-the-art (SOTA) baselines, a relative improvement of 6.64% on the average F1 is achieved. Moreover, an actual chemical industrial case is provided to verify the effect of the GSC-MAD and a relative improvement of 4.03% is achieved on the F1 metric compared with SOTA baselines. Comparison experiment results show that the proposed method achieves the SOTA results in terms of current baselines. Further experiment analysis shows the good interpretability of the proposed method for detected anomalies.
更多查看译文
关键词
Anomaly detection,Time series analysis,Measurement,Feature extraction,Deep learning,Valves,Monitoring,graph neural network (GNN),industrial systems,interpretability analysis,multivariate time series
AI 理解论文
溯源树
样例
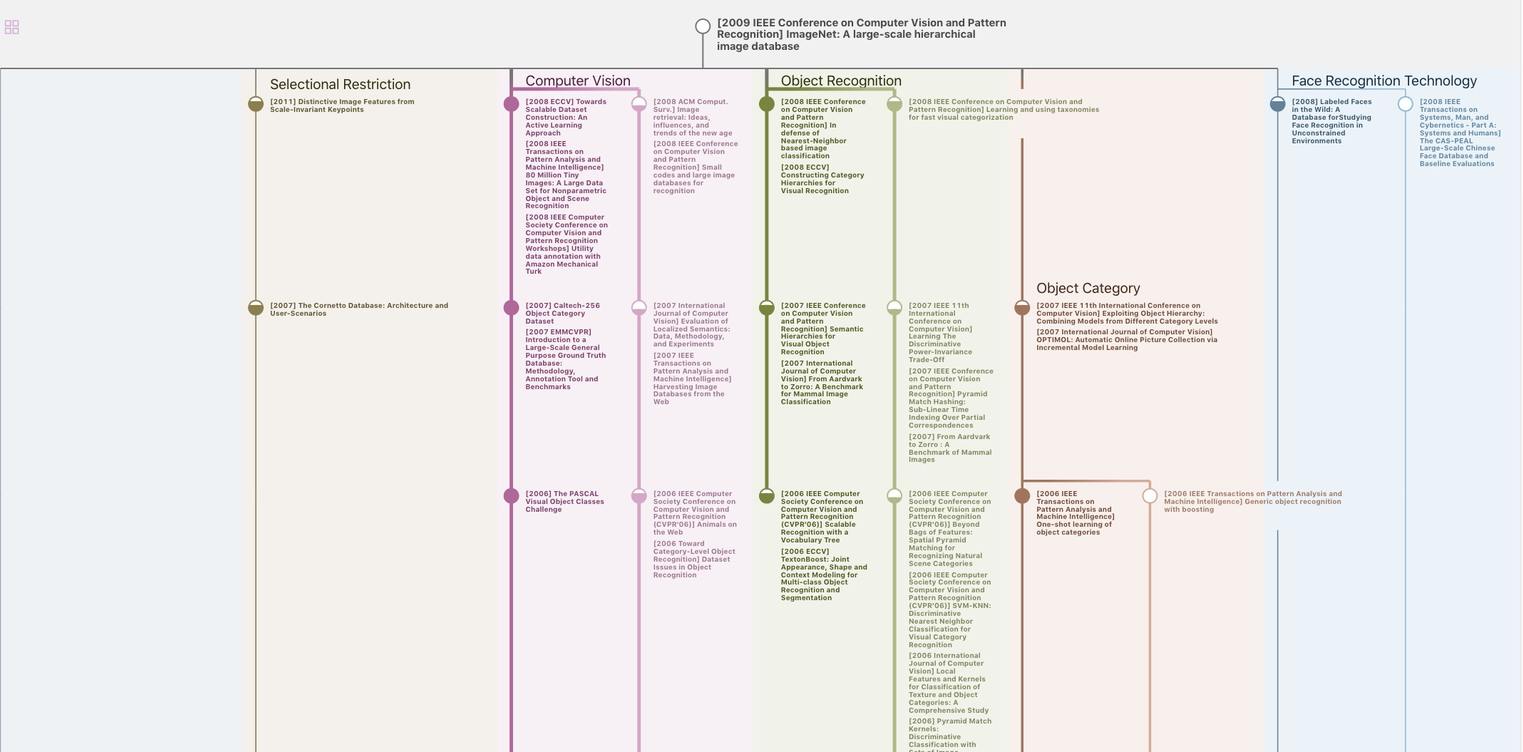
生成溯源树,研究论文发展脉络
Chat Paper
正在生成论文摘要