BSS-TFNet: Attention-Enhanced Background Signal Suppression Network for Time-Frequency Spectrum in Magnetic Particle Imaging
IEEE TRANSACTIONS ON EMERGING TOPICS IN COMPUTATIONAL INTELLIGENCE(2024)
摘要
Magnetic particle imaging (MPI) is a rapidly developing medical imaging modality, which uses the nonlinear response of superparamagnetic iron oxide nanoparticles to the applied magnetic field to image their spatial distribution. Background signal is the main source of artifacts in MPI, which mainly includes harmonic interference and Gaussian noise. For different sources of noise, the existing methods directly process the time domain signal to achieve signal enhancement or construct system function by frequency domain signal to obtain high-quality reconstructed images. However, due to the randomness and variety of the background signal, the existing methods fail to eliminate all kinds of noise at the same time, especially when the noise is nonlinear. In this work, we proposed a deep learning method adopting self-attention mechanism, which can effectively suppress different levels of harmonic interference and Gaussian noise simultaneously. Our method deals with the two-dimensional time-frequency spectrum acquired by short-time Fourier transform from the temporal signal, learning global features and local features between time and frequency domain through the network, to achieve the purpose of reducing background noise. The performance of our method is analyzed via simulation experiments and imaging experiments performed with an in-house MPI scanner, which shows that our method can effectively suppress background signals and obtain high-quality MPI images.
更多查看译文
关键词
Magnetic particle imaging,deep learning,self-attention mechanism,time-frequency spectrum,background signal
AI 理解论文
溯源树
样例
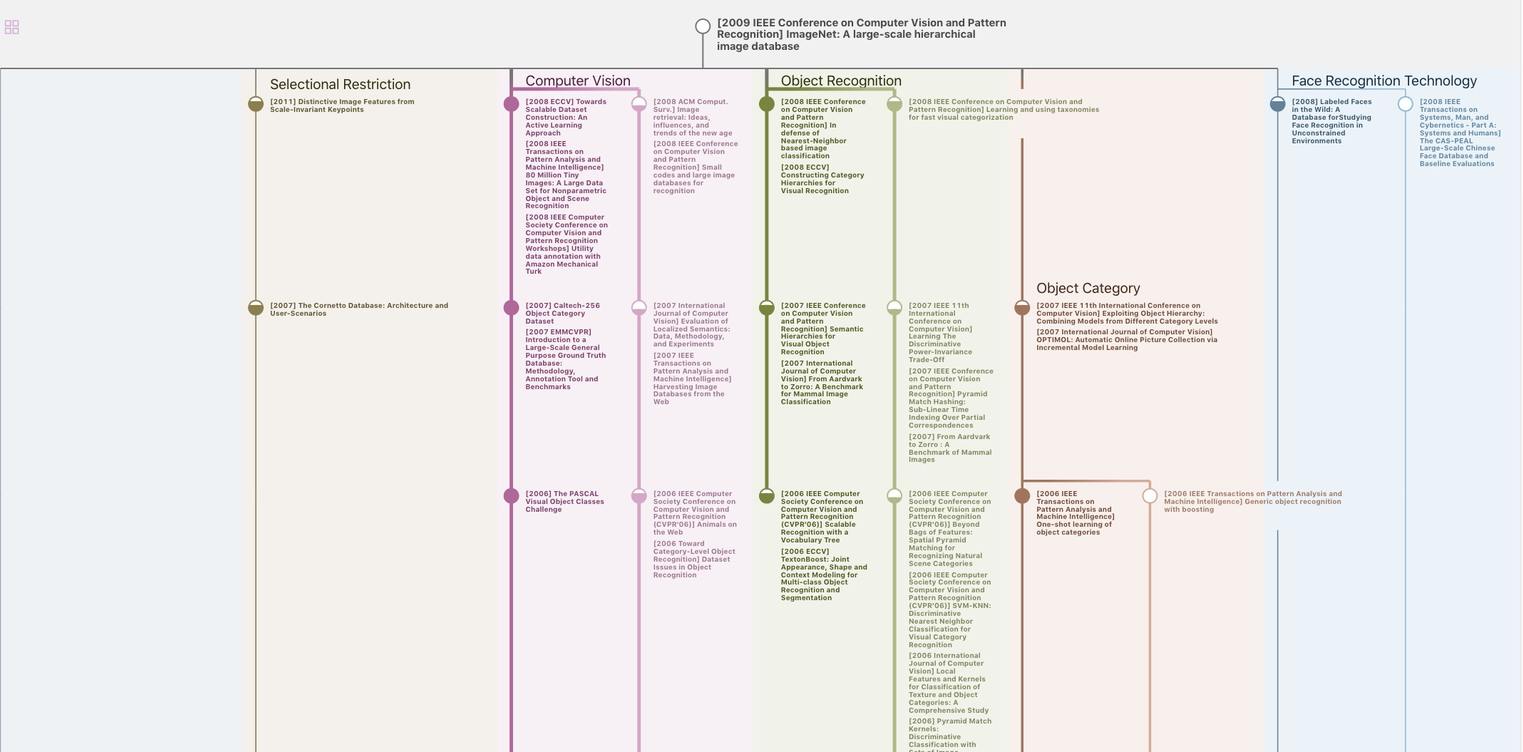
生成溯源树,研究论文发展脉络
Chat Paper
正在生成论文摘要