Image Classification on Hypersphere Loss
IEEE TRANSACTIONS ON INDUSTRIAL INFORMATICS(2024)
摘要
The effectiveness of an image classification system depends on the following two key components: 1) the feature learning module and 2) the classification module. A well-designed loss function can not only enhance the classification ability of the latter but also improve the feature extraction capabilities of the former. This article devises a novel hypersphere loss function, which enhances the intraclass compactness and interclass separability of feature vectors given by the feature learning module. Furthermore, a new generalized class center is introduced into the loss function to handle the inevitable variability in samples (such as illumination, background, blurriness, low resolution, etc.) within the same class. Then, an alternative learning strategy is employed to optimize trainable parameters and class centers. Specifically, we first fix the trainable parameters of the deep learning model and calculate class centers using the exponentially weighted moving average method. Subsequently, we fix the generalized class centers and update the model's trainable parameters using mini-batch stochastic gradient descent. The proposed algorithm is evaluated on a range of typical tasks, including standard image classification, face verification, object detection, and retail product checkout. The results demonstrate that our proposed algorithm outperforms several state-of-the-art approaches.
更多查看译文
关键词
Constrained optimization,hypersphere,image classification,metric learning
AI 理解论文
溯源树
样例
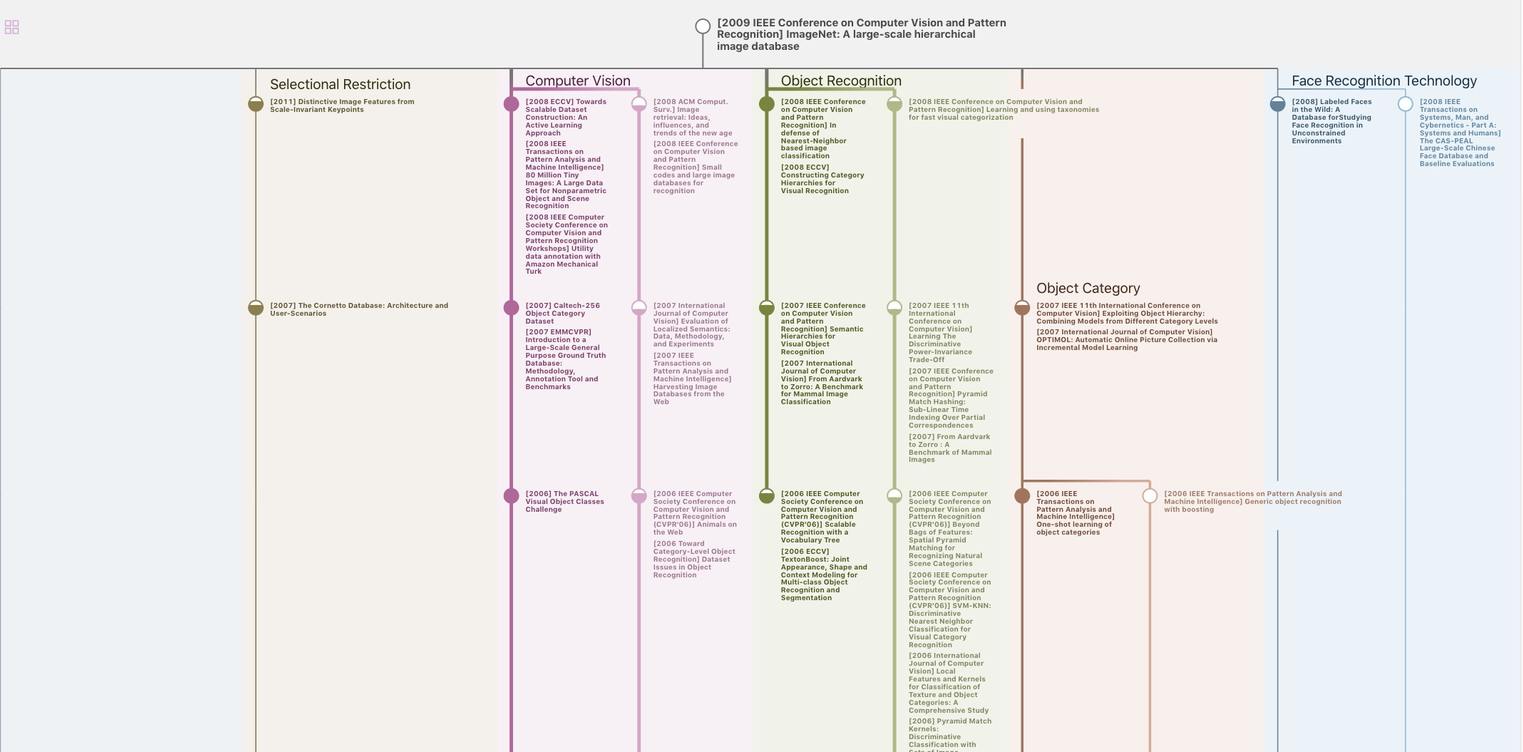
生成溯源树,研究论文发展脉络
Chat Paper
正在生成论文摘要