Multi-granularity-Aware Network for SAR Ship Detection in Complex Backgrounds
IEEE Geoscience and Remote Sensing Letters(2024)
摘要
Synthetic aperture radar (SAR) is a vital tool for ship detection, as it acquires high-resolution remote sensing images when optical images cannot penetrate. However, two primary challenges confronting SAR ship detection are complex backgrounds with islands, clutter, and land, as well as diverse scales of ship targets, particularly small ones, leading to numerous missed detections and false alarms. To overcome these challenges, we propose a multi-granularity-aware network (MGA-Net). Specifically, we design a multi-granularity hybrid feature fusion module (MGHF2M) to extract more representative local detail and global semantic information, enhancing the model’s capability to represent ship features to adapt to complex backgrounds. In addition, we design a multi-granularity feature synergy enhancement module (MGFSEM), which uses depthwise separable convolutions with different kernel sizes to extract features at different granularities and retain the original features, significantly improving the model’s representation of ship features at different scales. Experimental results show that our MGA-Net achieves the highest mAP and F1-score, surpassing eight advanced methods on three public datasets.
更多查看译文
关键词
Ship detection,multi-granularity,complex backgrounds,synthetic aperture radar (SAR)
AI 理解论文
溯源树
样例
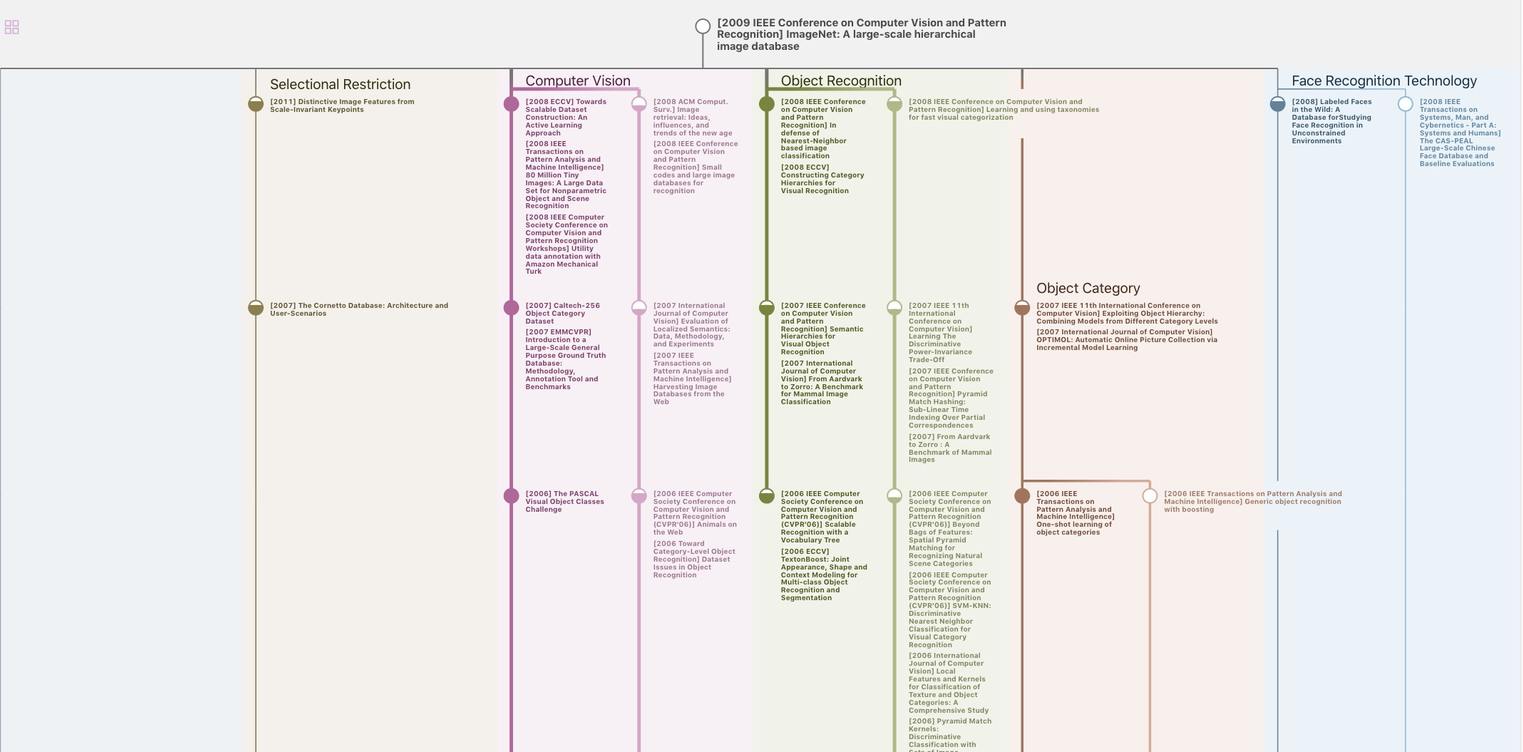
生成溯源树,研究论文发展脉络
Chat Paper
正在生成论文摘要