Bayesian Distributed Target Detection for Mismatched Signals in Sample-Starved Environment
IEEE GEOSCIENCE AND REMOTE SENSING LETTERS(2024)
摘要
In the case of distributed target detection in unknown Gaussian noise, training data are often limited, and signal mismatch is a common issue. To tackle these problems, we utilize the Bayesian theory by taking the noise covariance matrix as an inverse Wishart distribution. Our approach involves incorporating a fictitious determinist jamming signal in the signal-absence hypothesis to create a selective detector. Although this detector provides enhanced capability to reject mismatched signals, it comes at the cost of lower detection performance in the absence of signal mismatch. We propose a flexible Bayesian detector to tackle this limitation, wherein a customizable parameter can regulate the performance of mismatched signals. The tunable Bayesian detector is particularly robust to signal mismatch. In addition, it can achieve a higher probability of detection (PD) compared with existing methods when the tunable parameter is appropriately adjusted for matched signals. All the proposed Bayesian detectors can work with limited training data or even with no training data. The effectiveness of the proposed detectors is demonstrated through both simulated and actual data.
更多查看译文
关键词
Bayes methods,Detectors,Signal to noise ratio,Geoscience and remote sensing,Radar,Training data,Radar detection,Adaptive detection,Bayesian theory,signal mismatch
AI 理解论文
溯源树
样例
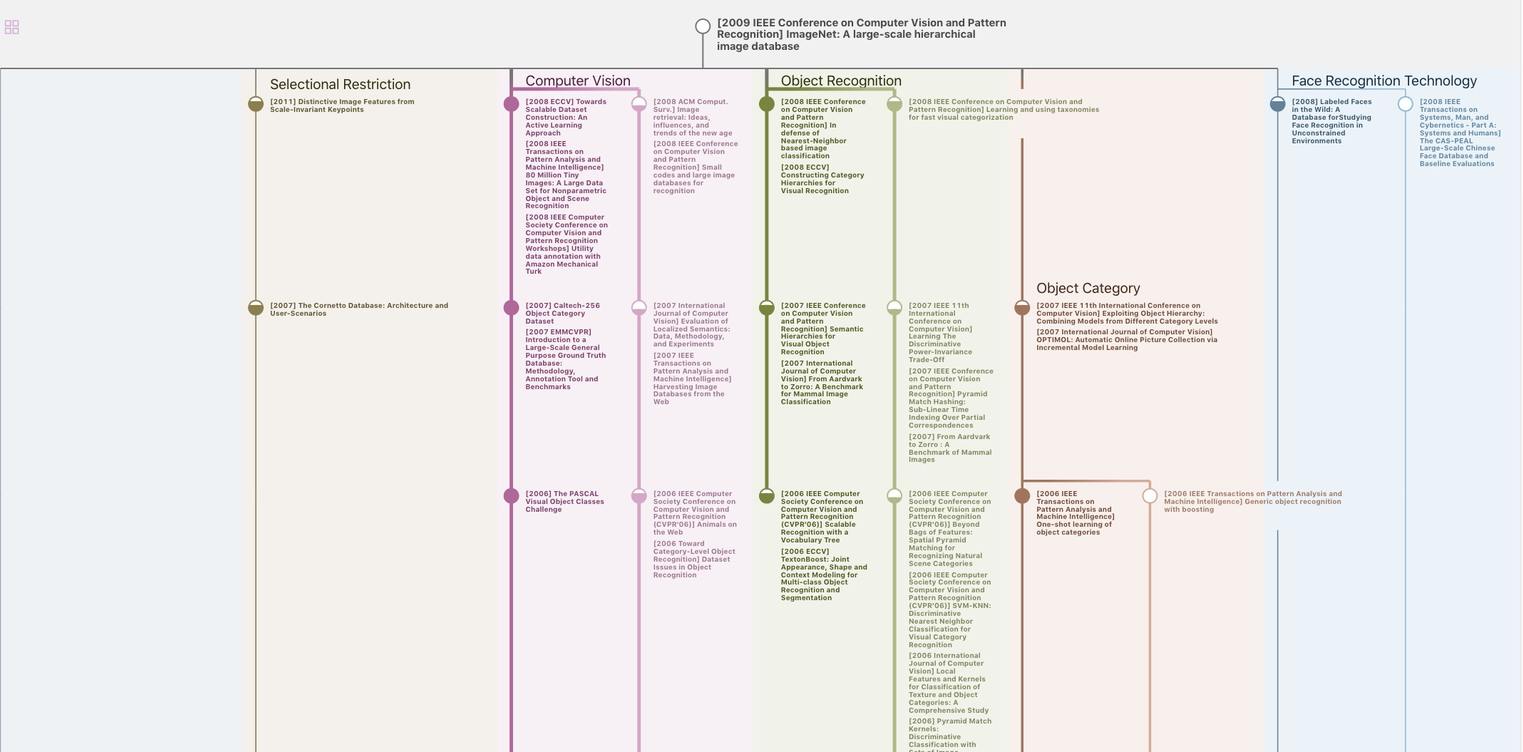
生成溯源树,研究论文发展脉络
Chat Paper
正在生成论文摘要