Prediction of Low-Frequency Seismic Data Without Relying on Wavelet Using Deep Learning
IEEE GEOSCIENCE AND REMOTE SENSING LETTERS(2024)
摘要
When using neural networks to predict low-frequency data of land seismic exploration, the biggest challenge is the difficulty in obtaining the wavelets of real seismic data. Training data that replicate the waveform structure of real seismic data are not easy to be generated. When a network trained with seismic records generated with other wavelets is directly applied to real data, it often can only approximate the prediction or, in some cases, cannot predict low-frequency data of land seismic data at all. Therefore, we propose a new approach that utilizes cross-convolutions to approximately unify the wavelets of real and simulated training seismic data. This allows the trained network to be used for predicting low-frequency data. Furthermore, the wavelets of the predicted low-frequency data are also known, which is more advantageous for subsequent full-waveform inversion (FWI). We employ two different wavelets to simulate real-world application scenarios. A simple layered model is utilized to validate the feasibility of the proposed method, and its generalization is tested on more complex Marmousi and overthrust models. For field data, better results can also be obtained using our methods.
更多查看译文
关键词
Training,Data models,Numerical models,Neural networks,Synthetic data,Band-pass filters,Testing,Deep learning,independent of wavelet,low-frequency signal prediction,time-series neural networks
AI 理解论文
溯源树
样例
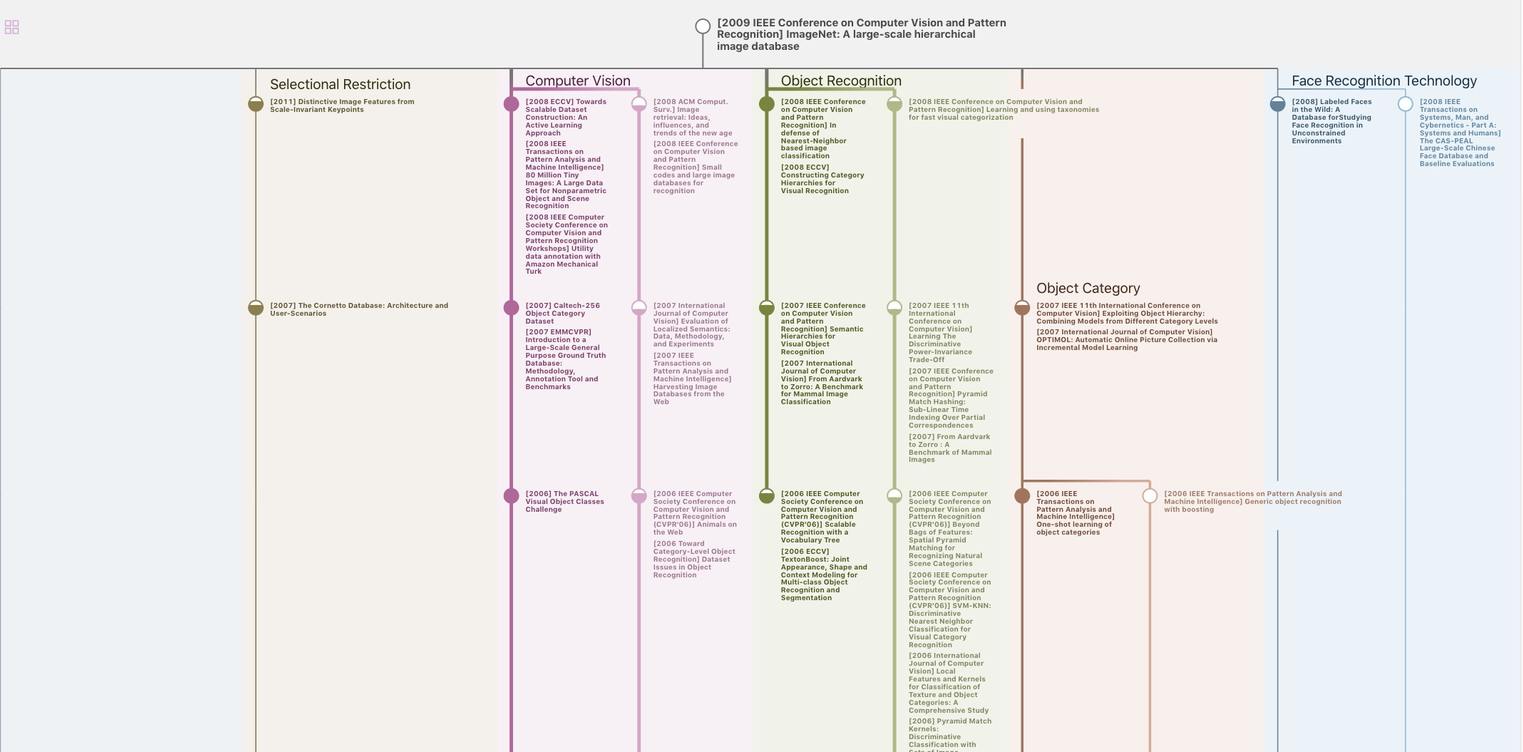
生成溯源树,研究论文发展脉络
Chat Paper
正在生成论文摘要