A FeFET-Based ADC Offset Robust Compute-In-Memory Architecture for Streaming Keyword Spotting (KWS).
IEEE Trans. Emerg. Top. Comput.(2024)
摘要
Keyword spotting (KWS) on edge devices requires low power consumption and real-time response. In this work, a ferroelectric field-effect transistor (FeFET)-based compute-in-memory (CIM) architecture is proposed for streaming KWS processing. Compared with the conventional sequential processing scheme, the inference latency is reduced by 7.7×∼17.6× without energy efficiency loss. To make the KWS models robust to hardware non-idealities such as analog-to-digital converter (ADC) offset, an offset-aware training scheme is proposed. It consists of ADC offset noise injection and frame-wise normalization. This scheme effectively improves the mean accuracy and chip yield by 1.5%∼5.2%, and 5%∼39%, for TC-ResNet and DS-TC-ResNet (with MatchboxNet configuration), respectively. The proposed CIM architecture is implemented with ferroelectric field-effect transistor technology, with simulated low energy consumption of 1.65μJ/decision for 12-word keyword spotting using TC-ResNet8.
更多查看译文
关键词
Compute-in-memory (CIM),ferroelectric field-effect transistor (FeFET),keyword spotting (KWS),streaming processing
AI 理解论文
溯源树
样例
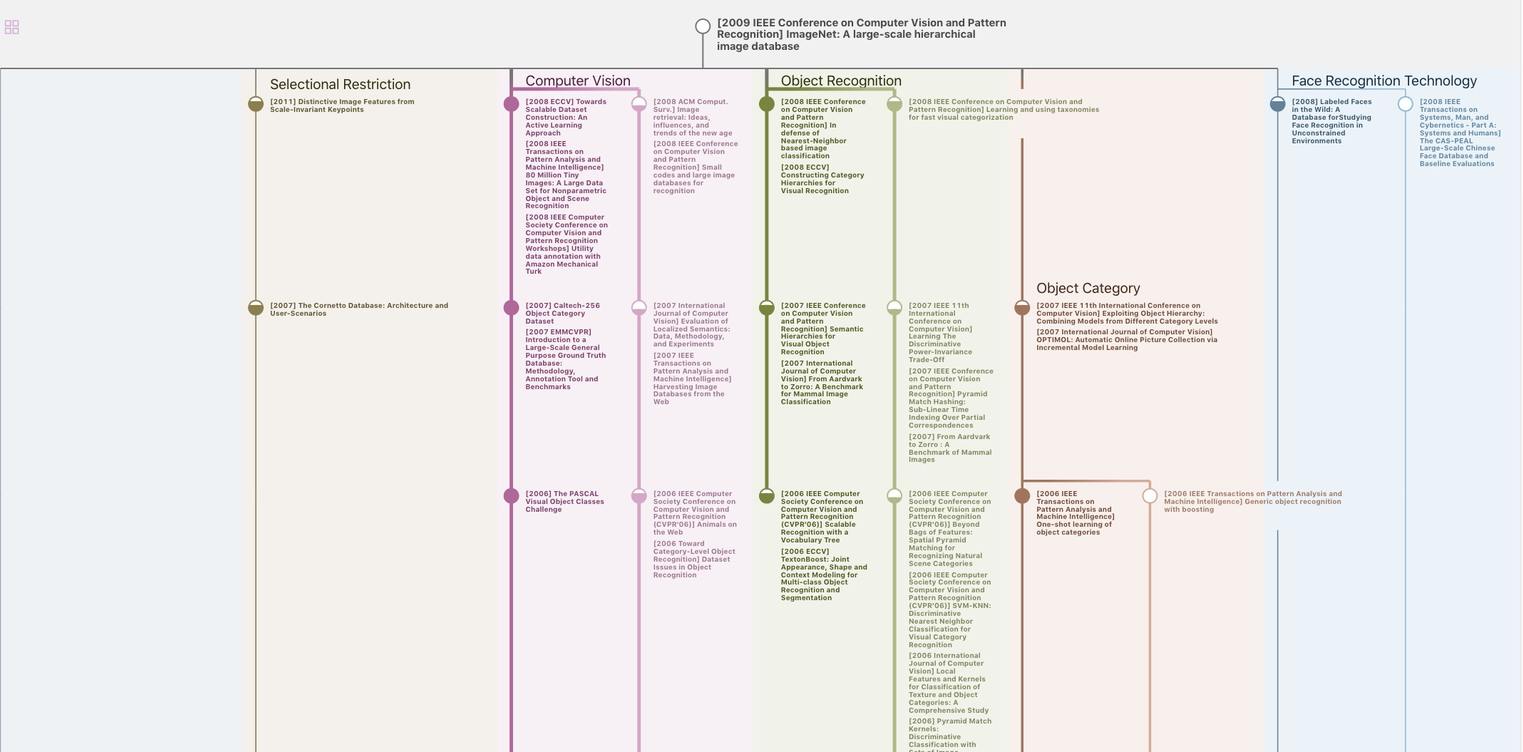
生成溯源树,研究论文发展脉络
Chat Paper
正在生成论文摘要