Energy-efficient Ferroelectric-FET-based Agent with Memory Trace for Enhanced Reinforcement Learning
IEEE Electron Device Letters(2023)
摘要
In this work, for the first time, we algorithmically merge the memory trace effect into activation function in neural network for reinforcement learning, and a novel ferroelectric FET (FeFET) based agent with both activation function and plastic weight is proposed and experimentally demonstrated. By exploiting the physics of short-time scale dynamics of depolarization process induced by depolarization field, the activation function with memory trace effect can be emulated in one FeFET at ultra-low operating voltage of 0.5V. In addition, the plastic weight with multilevel states can be realized by the long-time scale retention of spontaneous polarization in FeFET simultaneously. Moreover, based on the proposed FeFET-based agent, reinforcement learning is demonstrated with convergence rate boost and high energy efficiency. This work provides a promising highly-integrated agent solution for RL system.
更多查看译文
关键词
Ferroelectric FET (FeFET),reinforcement learning,memory trace,short-term and long-term memory
AI 理解论文
溯源树
样例
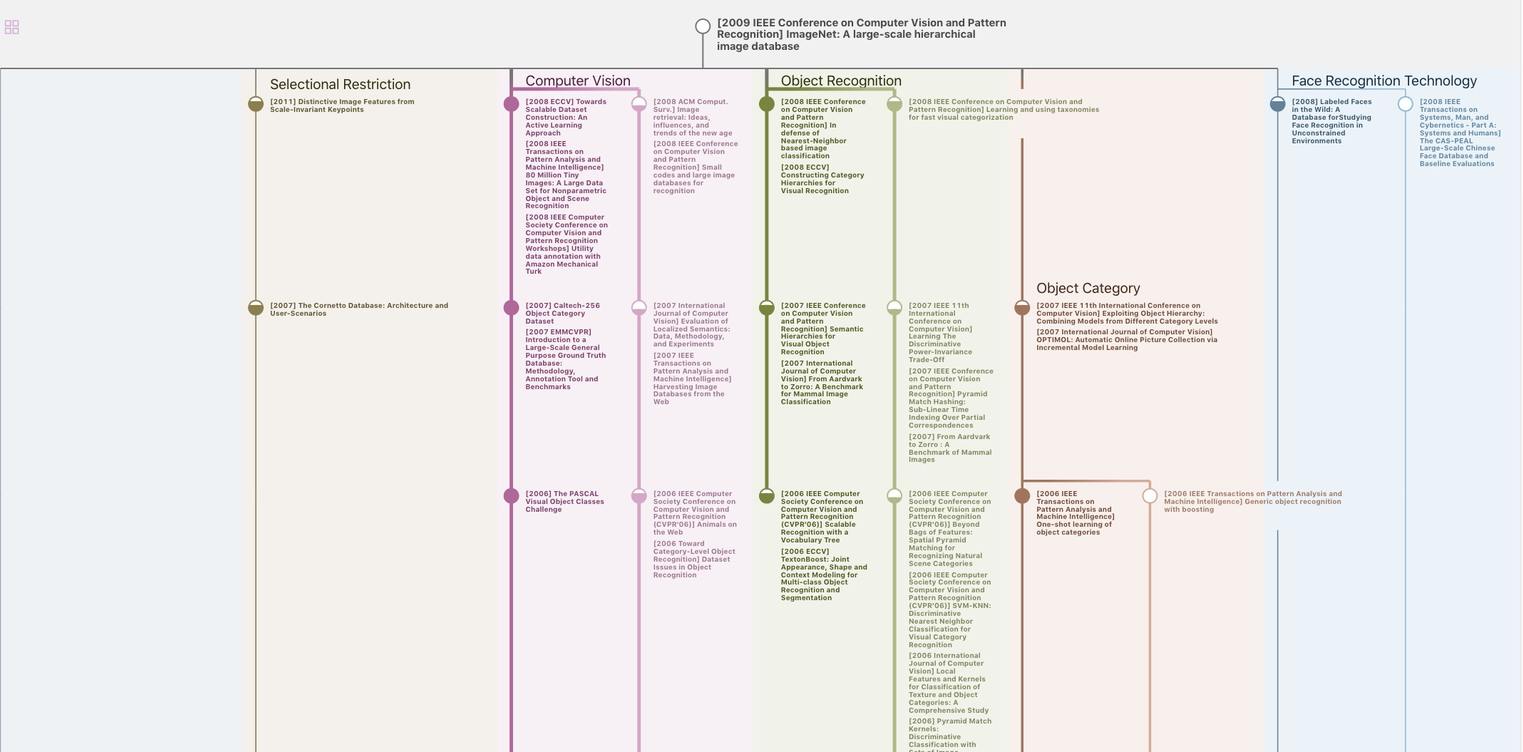
生成溯源树,研究论文发展脉络
Chat Paper
正在生成论文摘要