Dynamic Memory-Based Curiosity: A Bootstrap Approach for Exploration in Reinforcement Learning
IEEE TRANSACTIONS ON EMERGING TOPICS IN COMPUTATIONAL INTELLIGENCE(2023)
摘要
The sparsity of extrinsic rewards presents a significant challenge for deep reinforcement learning (DRL). As an alternative, researchers have focused on intrinsic rewards to improve exploration efficiency. One of the most representative approaches is utilizing curiosity. However, the challenge of designing effective intrinsic rewards remains, as artificial curiosity differs significantly from human curiosity. In this article, we introduce a novel curiosity approach for DRL, named DyMeCu, which stands for Dynamic Memory-based Curiosity. Inspired by human curiosity and information theory, DyMeCu constructs a dynamic memory using the online learner following the bootstrap paradigm. Additionally, we design a two-learner architecture inspired by ensemble techniques to access curiosity better. The information gap between the two learners serves as the intrinsic reward for agents, and the state information is consistently consolidated into the dynamic memory. Compared with previous curiosity methods, DyMeCu can better mimic human curiosity by using a dynamic memory that can be dynamically grown based on a bootstrap paradigm with two learners. Large-scale empirical experiments on multiple benchmarks, including DeepMind Control Suite and Atari Suite, demonstrate that DyMeCu outperforms competitive curiosity-based methods with or without extrinsic rewards. In the Atari Suite, DyMeCu achieves a mean human-normalized score of 5.076 on a subset of 26 Atari games, achieving a 77.4% relative improvement over the best other baselines. In the DeepMind Control Suite, DyMeCu presents new state-of-the-art results across 11 tasks of all 12 when compared to curiosity-based methods and other pre-training strategies.
更多查看译文
关键词
Deep reinforcement learning,curiosity,exploration,intrinsic rewards
AI 理解论文
溯源树
样例
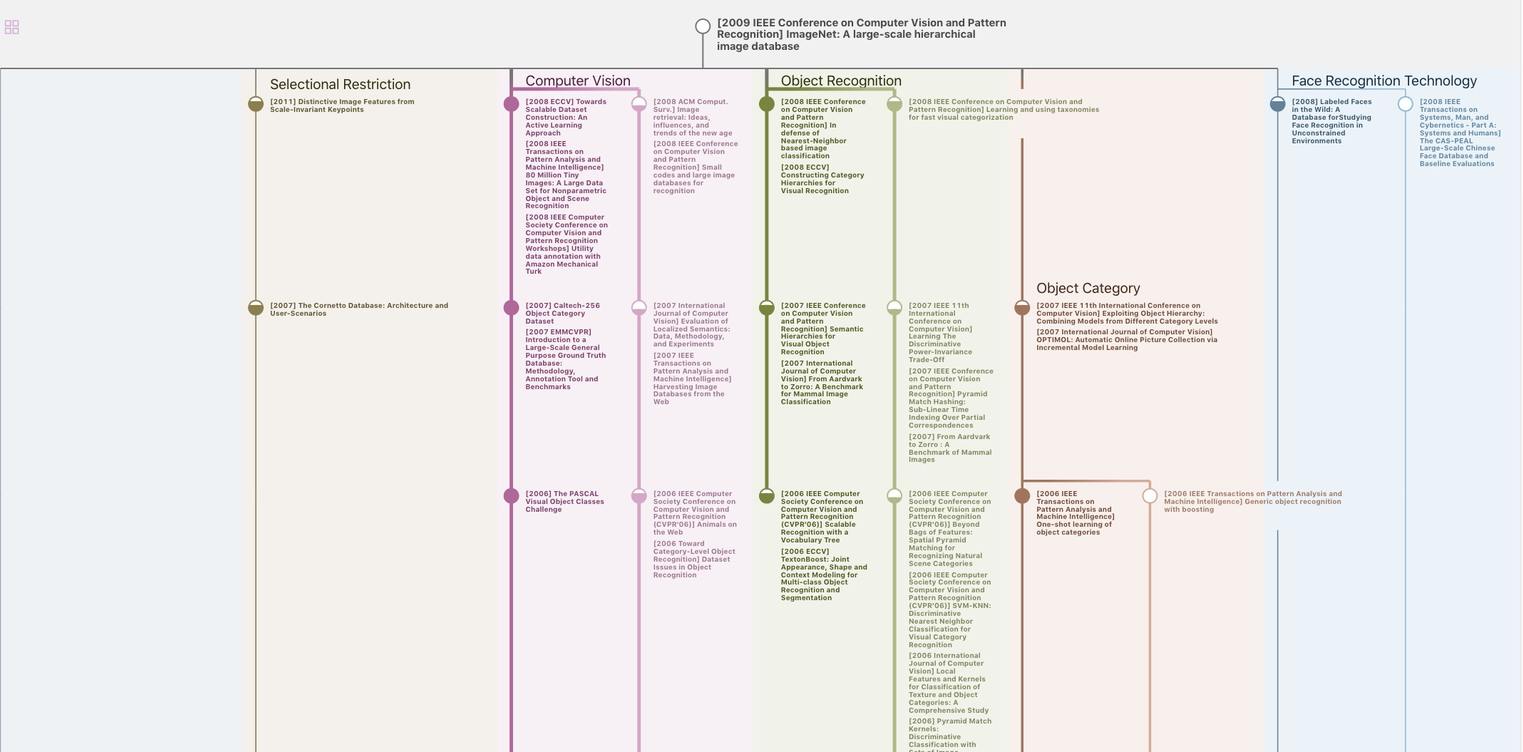
生成溯源树,研究论文发展脉络
Chat Paper
正在生成论文摘要