Verifiable Privacy-Preserving Image Retrieval in Multi-Owner Multi-User Settings
IEEE TRANSACTIONS ON EMERGING TOPICS IN COMPUTATIONAL INTELLIGENCE(2024)
摘要
Recently, the Convolutional Neural Network (CNN) based Content-Based Image Retrieval (CBIR) has substantially improved the search accuracy of encrypted images. Further, the increasing trends in outsourcing the CNN-based CBIR service to the cloud relieve the users from severe computation and storage requirements. However, all of the existing CNN-based CBIR schemes lack the support for Multi-owner multi-user settings and thus significantly limit the flexibility and scalability of cloud computing. To fill this gap, we propose a Verifiable Privacy-preserving Image Retrieval scheme in the Multi-owner multi-user setting (VPIRM). VPIRM utilizes a two-phase transfer learning technique. In the first phase, convolution base transfer takes the pre-trained CNN model for feature extraction, which addresses the issue of scarce training data at the image owner (IO) side. In the second phase, novel secure transfer enables the image user (IU) to construct a query feature vector over the same feature space on which the model is trained. Meanwhile, our scheme simultaneously supports fine-grained access control, dynamic updates, and results correctness and completeness on a malicious cloud server. Finally, a thorough security analysis shows that the scheme achieves various privacy requirements under the known-ciphertext and known-background threat model.
更多查看译文
关键词
Feature extraction,Indexes,Convolutional neural networks,Visualization,Encryption,Access control,Image retrieval,Attribute-based access control,content-based image retrieval,privacy-preserving,secure transfer learning
AI 理解论文
溯源树
样例
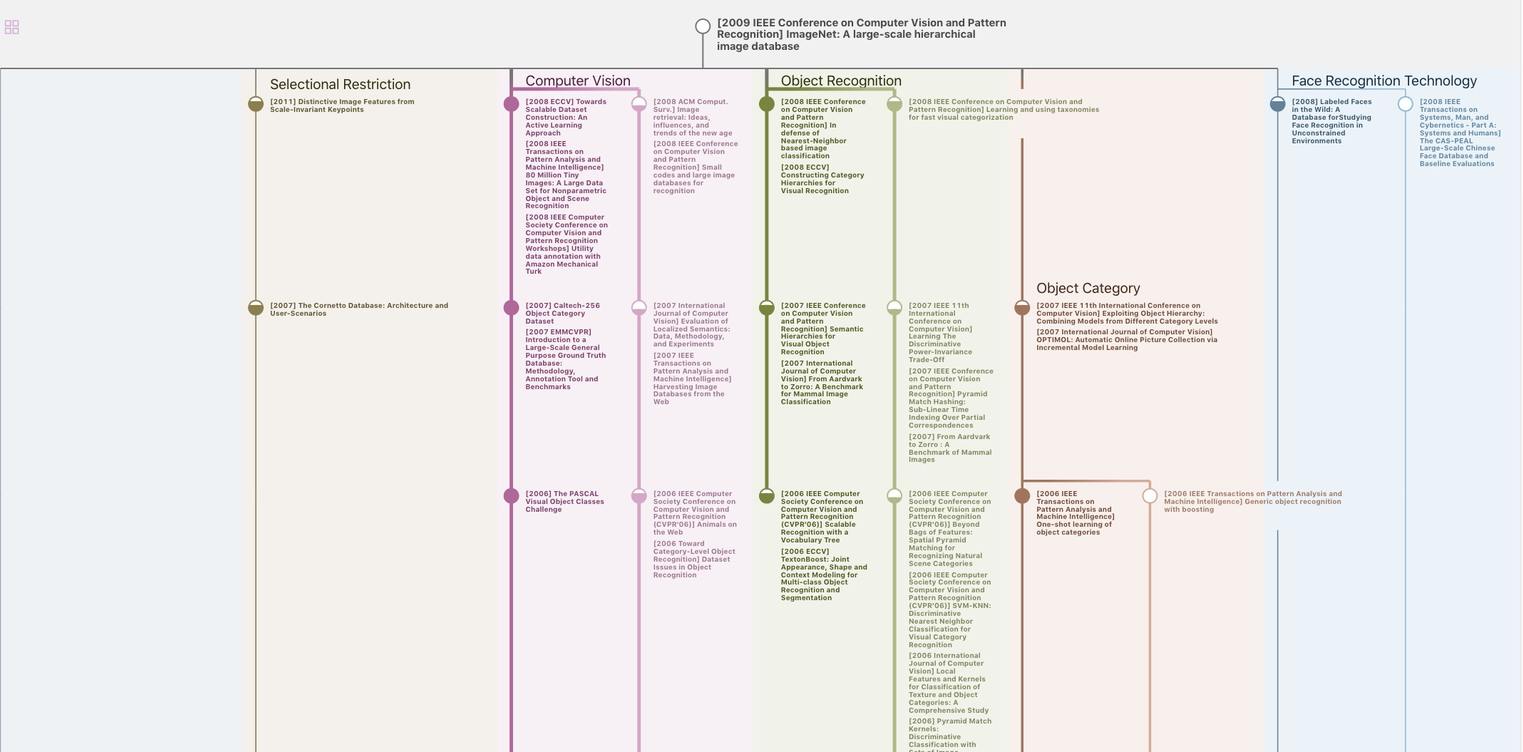
生成溯源树,研究论文发展脉络
Chat Paper
正在生成论文摘要