Feature-Enhanced Convolutional Attention for Unstable Rock Detection in Aerial Images
IEEE GEOSCIENCE AND REMOTE SENSING LETTERS(2024)
摘要
This study introduces the feature-enhanced convolutional attention integration (FEC-AI) module, an innovative convolutional neural network (CNN) algorithm specifically tai-lored for the meticulous detection of small, challenging objects in high-resolution remote-sensing imagery, emphasizing unstable rock formation monitoring. FEC-AI significantly advances CNN-based feature extraction and representation through acombination of advanced techniques. These include the cross-layer attention module (CLAM), which enriches feature maps with multiscale contextual details; the offset-aware adjustment module (OAM) for precise spatial refinement of feature localization; and the contextual feature aggregation (CFA) process, which synergizes these refined features for enhanced detection efficacy. Concurrently, we introduce the RSUR-2D dataset, a comprehensive compilation of 1557 rigorously annotated images depicting karst landscapes around Beijing, expressly designed to challenge and advance remote-sensing algorithms in geological hazard analysis. Through extensive testing on the RSUR-2D and established COCO datasets, the FEC-AI module demonstrated out standing performance in small object detection, achieving amean average precision at mAP(50)of 66.0% and mAP(s)of 21.1%on the RSUR-2D dataset. The RSUR-2D dataset, a valuable resource for the research community, is publicly accessible athttps://github.com/chenmu1204/czx.
更多查看译文
关键词
Feature extraction,Rocks,Hazards,Cross layer design,Training,Convolutional neural networks,Spatial resolution,Aerial,feature enhancement,geological hazard detection,small object detection
AI 理解论文
溯源树
样例
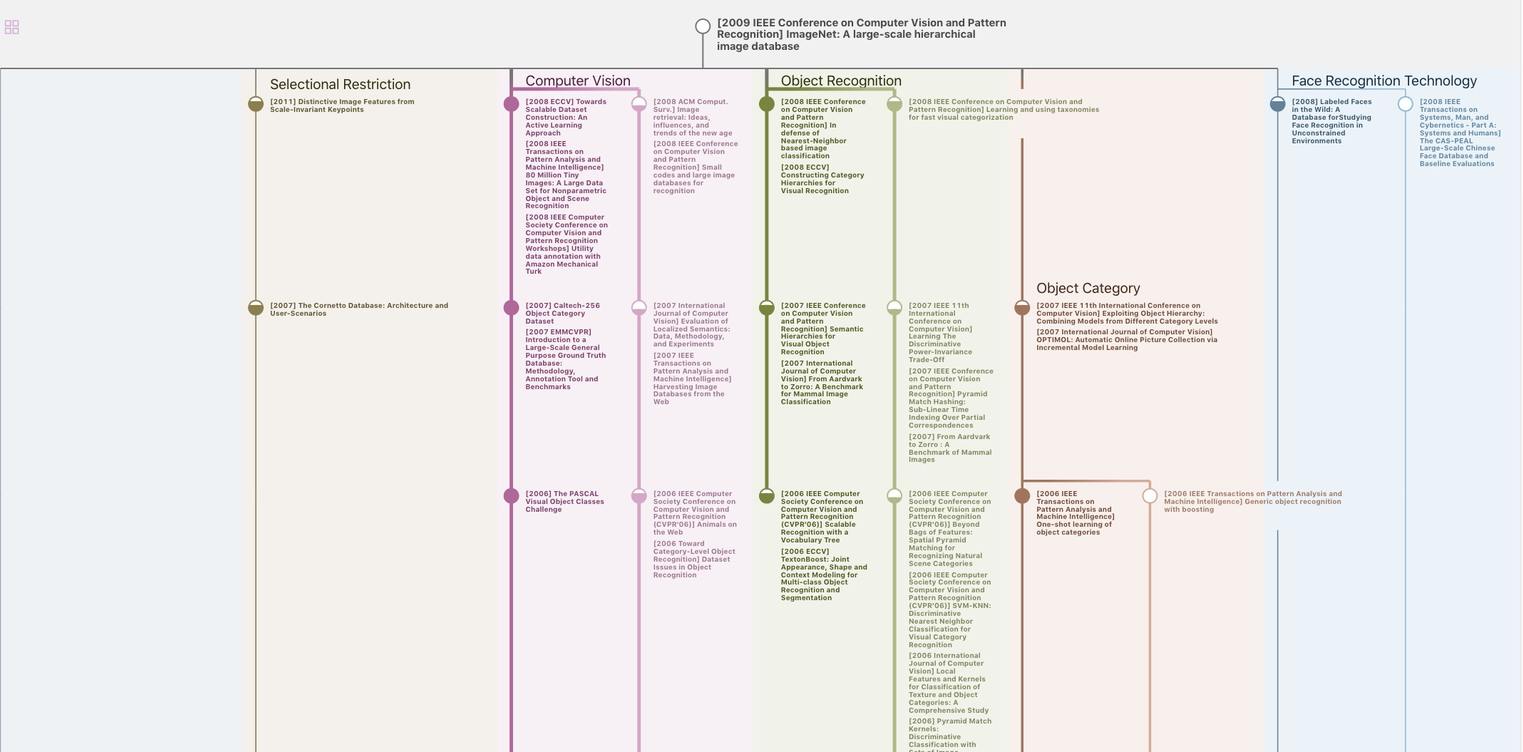
生成溯源树,研究论文发展脉络
Chat Paper
正在生成论文摘要