Evaluation of Frameworks That Combine Evolution and Learning to Design Robots in Complex Morphological Spaces
IEEE Transactions on Evolutionary Computation(2023)
摘要
Jointly optimising both the body and brain of a robot is known to be a challenging task, especially when attempting to evolve designs in simulation that will subsequently be built in the real world. To address this, it is increasingly common to combine evolution with a learning algorithm that can either improve the inherited controllers of new offspring to fine tune them to the new body design or learn them from scratch. In this paper an approach is proposed in which a robot is specified indirectly by two compositional pattern producing networks (CPPN) encoded in a single genome, one which encodes the brain and the other the body. The body part of the genome is evolved using an evolutionary algorithm (EA), with an individual learning algorithm (also an EA) applied to the inherited controller to improve it. The goal of this paper is to determine how to utilise the results of learning process most effectively to improve task performance of the robot. Specifically, three variants are investigated: (1) evolution of the body+controller only; (2) a learning algorithm is applied to the inherited controller with the learned fitness assigned to the genome; (3) learning is applied and the genome is updated with the learned controller, as well as being assigned the learned fitness. Experiments are performed in three different scenarios chosen to favour different bodies and locomotion patterns. It is shown that better performance can be obtained using learning but only if the learned controller is inherited by the offspring.
更多查看译文
关键词
Morphological Evolution,Evolution and Learning,Embodied Intelligence
AI 理解论文
溯源树
样例
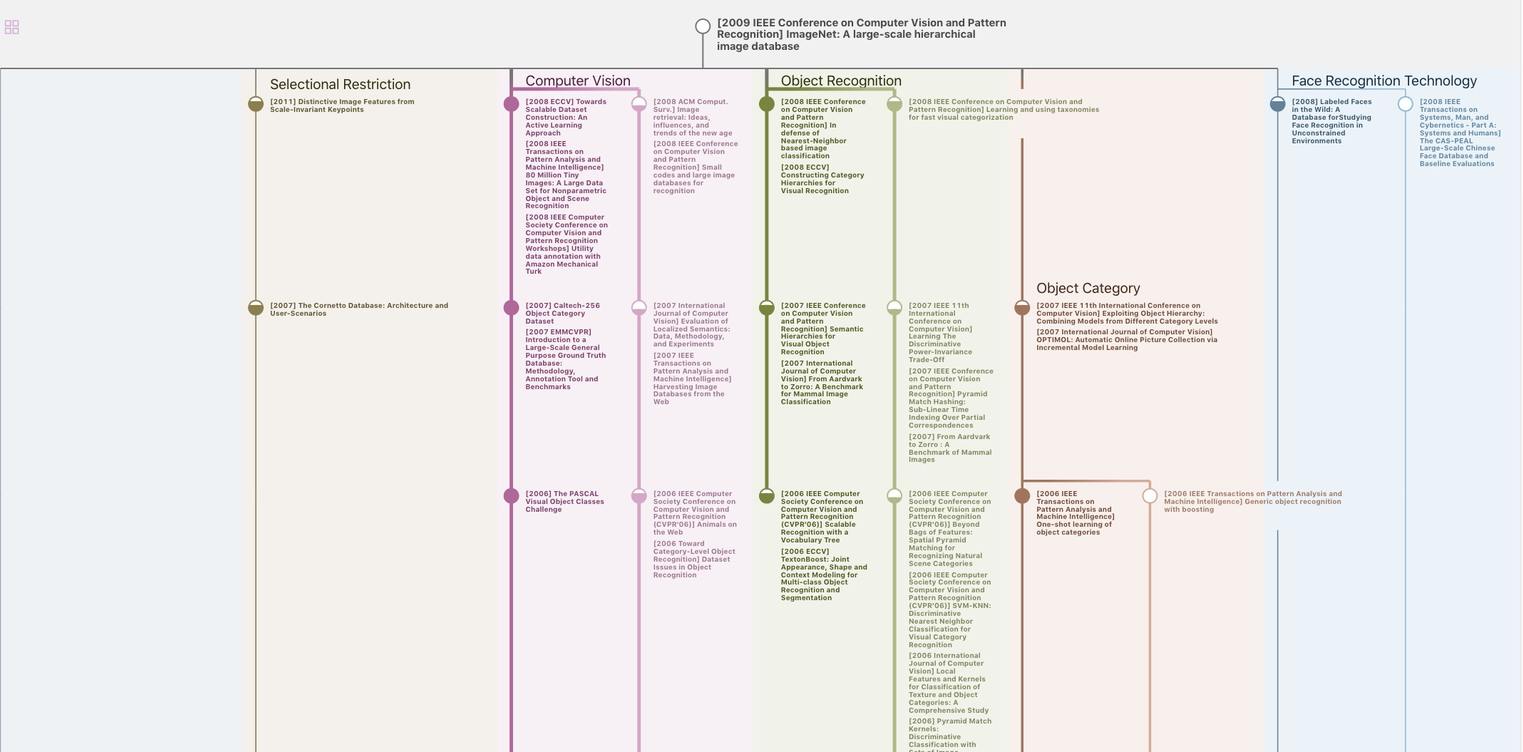
生成溯源树,研究论文发展脉络
Chat Paper
正在生成论文摘要