Controlling a House’s Air-Conditioning Using Nonlinear Model Predictive Control
IEEE Embedded Systems Letters(2024)
摘要
As per literature, there is a potential for energy savings of (5 to 20) % using building embedded control systems. This work aims to control a house air-conditioning system using Model Predictive Control (MPC) with a Neural State Space Prediction (NSSP) Model to maintain interior temperature set-point and reduce energy consumption. Here we present a high-fidelity model that is validated by an experimental prototype of a house air-conditioning system that is controlled using a non-linear MPC. The house air-conditioning system models the air-conditioner and the thermal dynamics of the house. The outdoor temperature is modeled by simulated signals and real measurements. The controller problem is to maintain a house temperature within 20 to 22 ∘C and to minimize energy costs. Compared with the generic nonlinear MPC controller, multistage nonlinear MPC provides a more flexible and efficient way to implement MPC with staged costs and constraints.
更多查看译文
关键词
Model Predictive Control,Non-linear,Neural State-Space Prediction,air conditioner
AI 理解论文
溯源树
样例
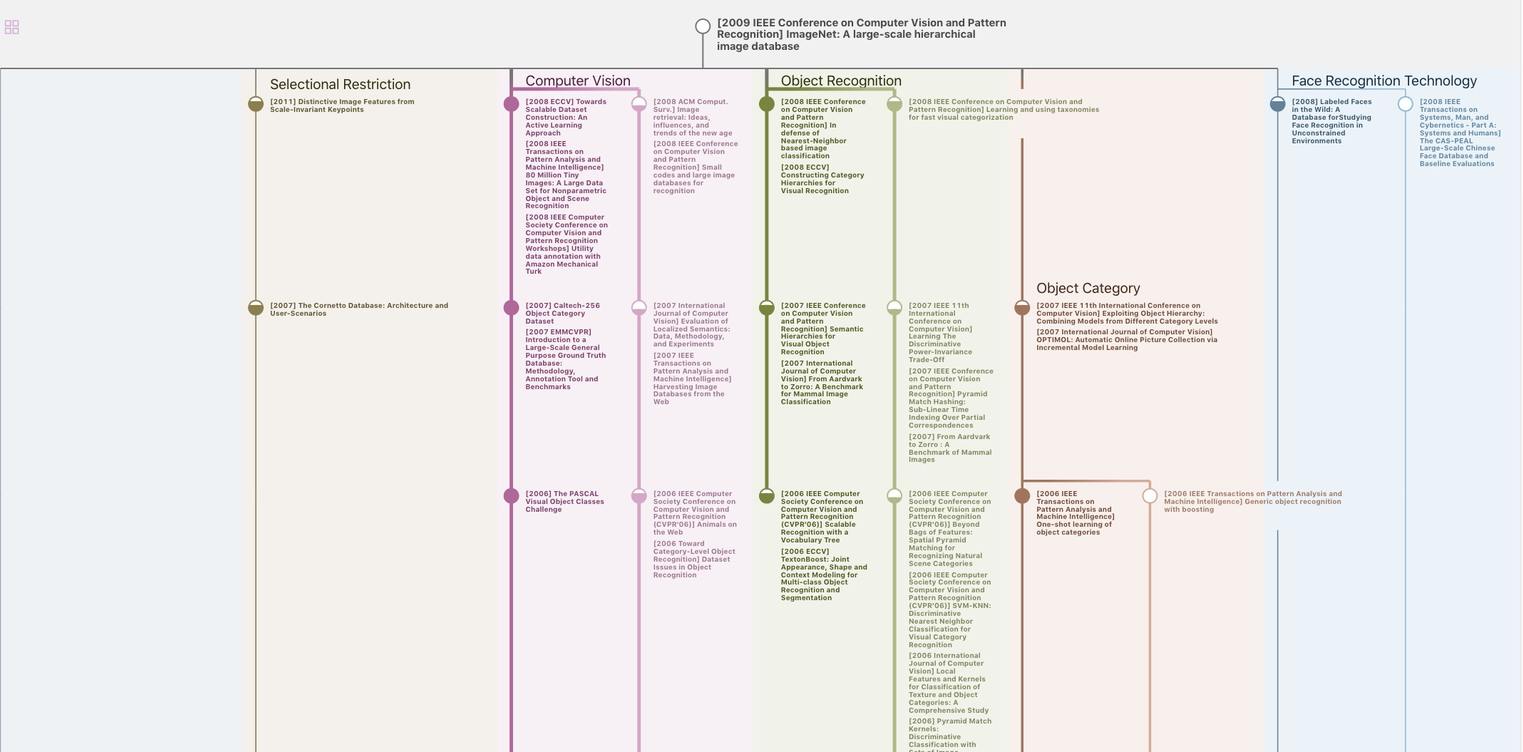
生成溯源树,研究论文发展脉络
Chat Paper
正在生成论文摘要