Locally Private Set-valued Data Analyses: Distribution and Heavy Hitters Estimation
IEEE Transactions on Mobile Computing(2023)
摘要
In many mobile applications, user-generated data are presented as set-valued data. To tackle potential privacy threats in analyzing these valuable data, local differential privacy has been attracting substantial attention. However, existing approaches only provide sub-optimal utility and are expensive in computation and communication for set-valued data distribution estimation and heavy-hitter identification. In this paper, we propose a utility-optimal and efficient set-valued data publication method (i.e.,
Wheel mechanism
). On the user side, the computational complexity is only
$O(\min \lbrace m\log m, m e^\epsilon \rbrace )$
and communication costs are
$O(\epsilon +\log m)$
bits, where
$m$
is the number of items,
$d$
is the domain size and
$\epsilon$
is the privacy budget, while existing approaches usually depend on
$O(d)$
or
$O(\log d)$
(
$d \gg m$
). Our theoretical analyses reveal the estimation errors have been reduced from the previously known
$O(\frac{m^{2} d}{n\epsilon ^{2}})$
to the optimal rate
$O(\frac{m d}{n\epsilon ^{2}})$
. Additionally, for heavy-hitter identification, we present a variant of the Wheel mechanism as an efficient frequency oracle, entailing only
$O(\sqrt{n})$
computational complexity. This heavy-hitter protocol achieves an identification bar of
$\tilde{O}(\frac{1}{\epsilon }\sqrt{\frac{m}{n} \log d})$
, reducing by a factor of
$\sqrt{m}$
relative to existing protocols. Extensive experiments demonstrate our methods are 3-100x faster than existing approaches and have optimized statistical efficiency.
更多查看译文
关键词
local differential privacy,frequency estimation,heavy-hitter identification,distributed data aggregation
AI 理解论文
溯源树
样例
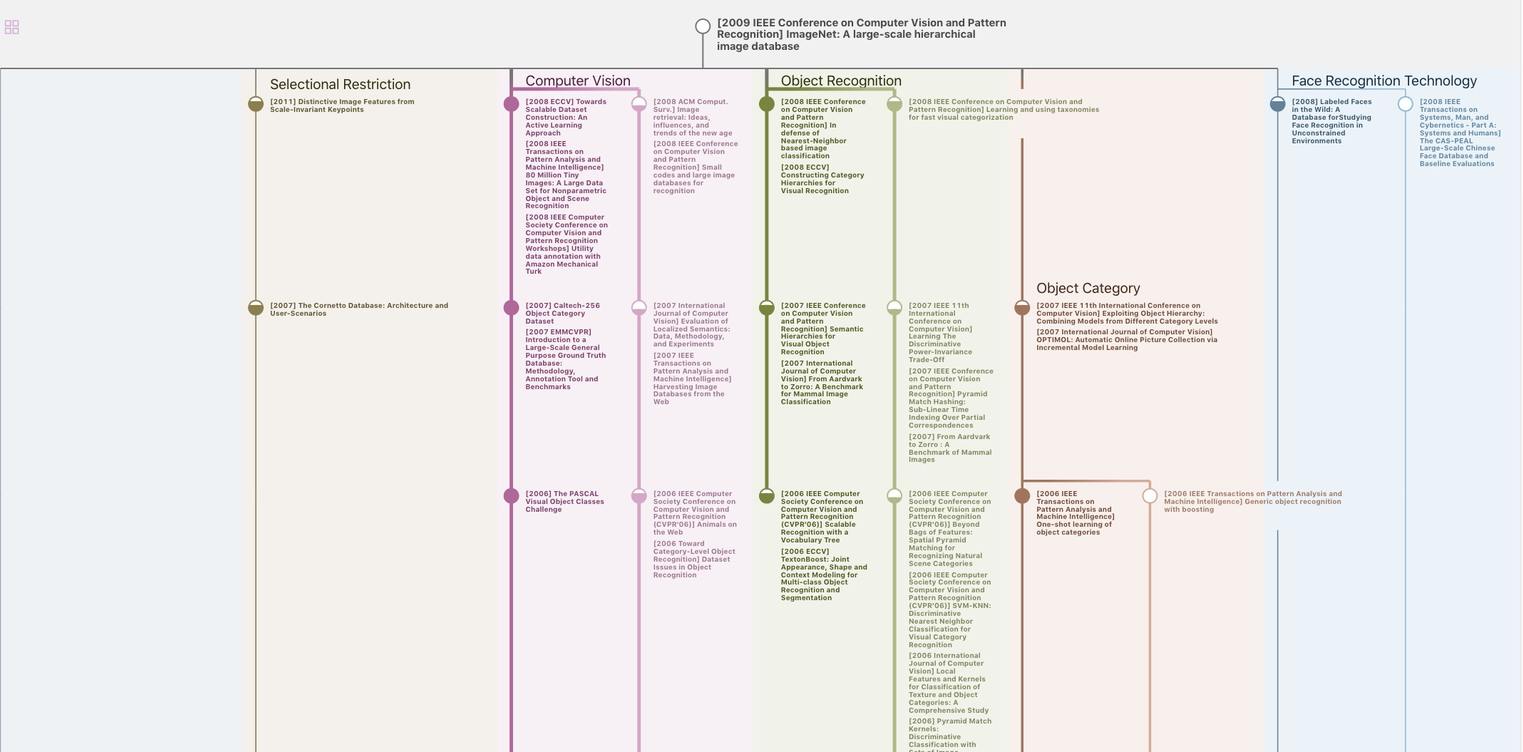
生成溯源树,研究论文发展脉络
Chat Paper
正在生成论文摘要