Deep Reinforcement Learning-Based Contract Incentive Mechanism for Joint Sensing and Computation in Mobile Crowdsourcing Networks
IEEE Internet of Things Journal(2023)
摘要
Mobile crowdsourcing network is a promising paradigm to leverage mobile users (MUs) to perform large-scale sensing task. Due to limited sensing-computation resource and data security risk, it is necessary to design an efficient incentive mechanism to motivate MUs to complete crowdsourcing task. In this paper, a deep reinforcement learning (DRL)-based contract incentive mechanism is proposed by jointly considering participation contribution, sensing task, and computation resource of MUs. Specifically, considering the heterogeneous willingness of the MUs, we formulate a three-dimensional sensing-computation-reward contract incentive mechanism to obtain the maximum utility of mobile crowdsourcing platform. Moreover, based on the individual rationality and incentive compatibility constraints, we derive the optimal contract under the partial information asymmetry scenario. In the case of the complete information asymmetry scenario, we formulate the contract incentive issue as an Markov decision process. Considering the infinite and continuous action and state spaces, we develop the deep deterministic method to obtain the efficient sensing task, computation resource, and incentive reward policy. Finally, we conduct numerical simulation to demonstrate the feasibility of our DRL-based contract crowdsourcing incentive mechanism.
更多查看译文
关键词
Mobile crowdsouring networks,incentive mechanism,joint sensing and computation,contract design,deep reinforcement learning
AI 理解论文
溯源树
样例
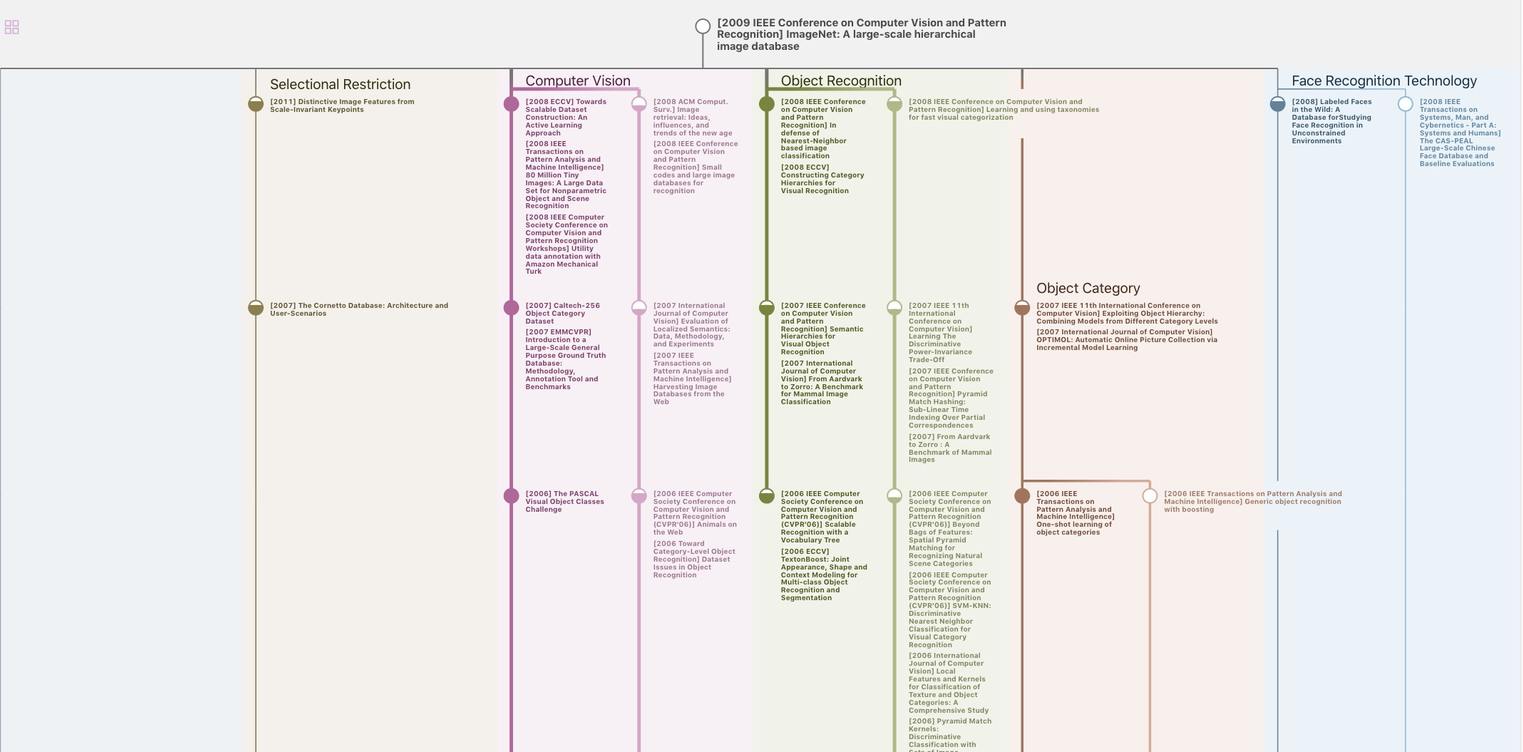
生成溯源树,研究论文发展脉络
Chat Paper
正在生成论文摘要