Towards Efficient Fire Detection in IoT Environment: A Modified Attention Network and Large-Scale Dataset
IEEE Internet of Things Journal(2023)
摘要
Advancements in deep learning and the Internet of Things (IoT) enable early fire detection through vision-based systems, reducing ecological, social, and economic damage. These systems necessitate lightweight, cost-effective convolutional neural networks (CNNs) for real-time operation. Effective deployment on AI-assisted edge devices is crucial for optimal performance. To mitigate this problem, we present the optimized fire attention network (OFAN) for effective and efficient fire detection. In the re-engineered attention block, we swapped the convolution layers by dilated variants and integrated additional dense layers to capture global context and refine more weight optimization. We calibrate the OFAN for real-time processing using a lightweight and efficient feature extractor backbone model. Additionally, a challenging fire dataset is a critical contribution that contains extremely diverse, blazing, and non-fire, captured in lighting and foggy environments. It advances traditional fire detection samples by considering low-light and foggy conditions. A comprehensive experiment is conducted over three widely used fire detection datasets, and our proposed OFAN outperforms state-of-the-art. The proposed OFAN achieved 96.23%, 96.54% and 94.63% accuracies over BoWFire, FD and the newly proposed DiverseFire dataset, respectively. Our research sets a standard for fire detection over edge devices, offering improved accuracy and better frames per second (FPS) performance.
更多查看译文
关键词
Attention Mechanism,Convolutional Neural Network,Deep Learning,Disaster Management,Fire Classification,Fire Detection,Fire Localization,IoT,Surveillance System
AI 理解论文
溯源树
样例
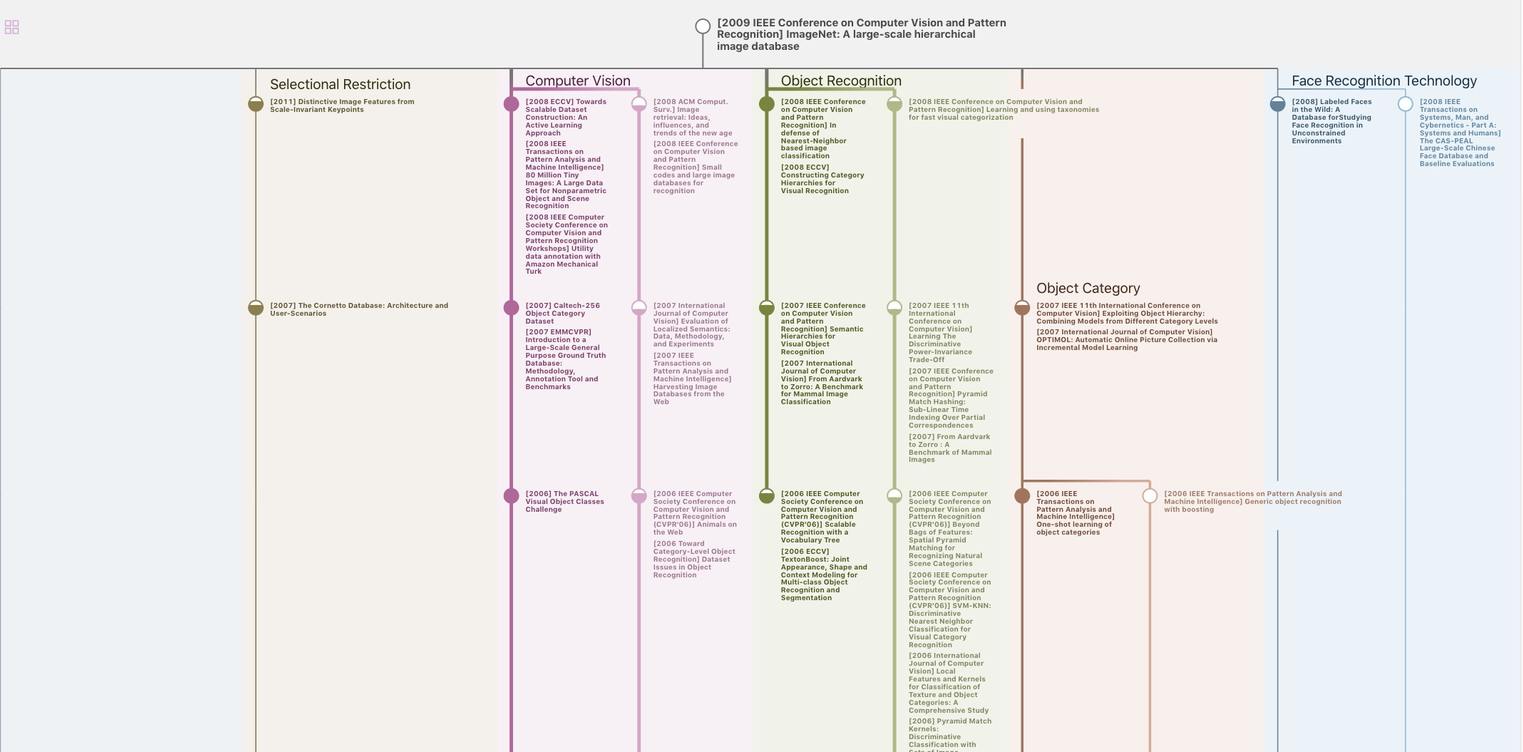
生成溯源树,研究论文发展脉络
Chat Paper
正在生成论文摘要