MASSnet: Deep-Learning-Based Multiple-Antenna Spectrum Sensing for Cognitive-Radio-Enabled Internet of Things.
IEEE Internet Things J.(2024)
摘要
Cognitive radio-based Internet of Things (CR-IoTs) provide an efficient spectrum management for IoT networks with massive wireless access and data transmission needs. As one of the key technologies of CR-IoT, spectrum sensing is of great research significance. Motivated by the recent boom on applications of deep learning in wireless communications networks and IoT, several spectrum sensing methods based on deep learning have emerged. However these algorithms train the sensing models with the extracted features of received signals and require a retraining of sensing models when the number of sensing antennas changes. Thus, we develop multiple-antenna spectrum sensing methods based on convolutional neural networks (MASSnet) using the in-phase (I) and quadrature (Q) components of the signals as the input. The three schemes of MASSnet also provide the flexibility to choose between retraining the sensing models or using the obtained models for different sensing antenna configurations. Experiment results demonstrate the superior performance of the proposed methods over existing deep learning-based spectrum sensing methods in terms of probability of detection especially in very low signal-to-noise ratio (SNR) condition. Furthermore, the proposed methods have good generalization ability to new noise distribution, new fading channel, different frequency offsets, and detecting signals with a new modulation even without retraining.
更多查看译文
关键词
Cognitive radio (CR),Internet of Things (IoT),multiple-antenna,spectrum sensing,deep learning
AI 理解论文
溯源树
样例
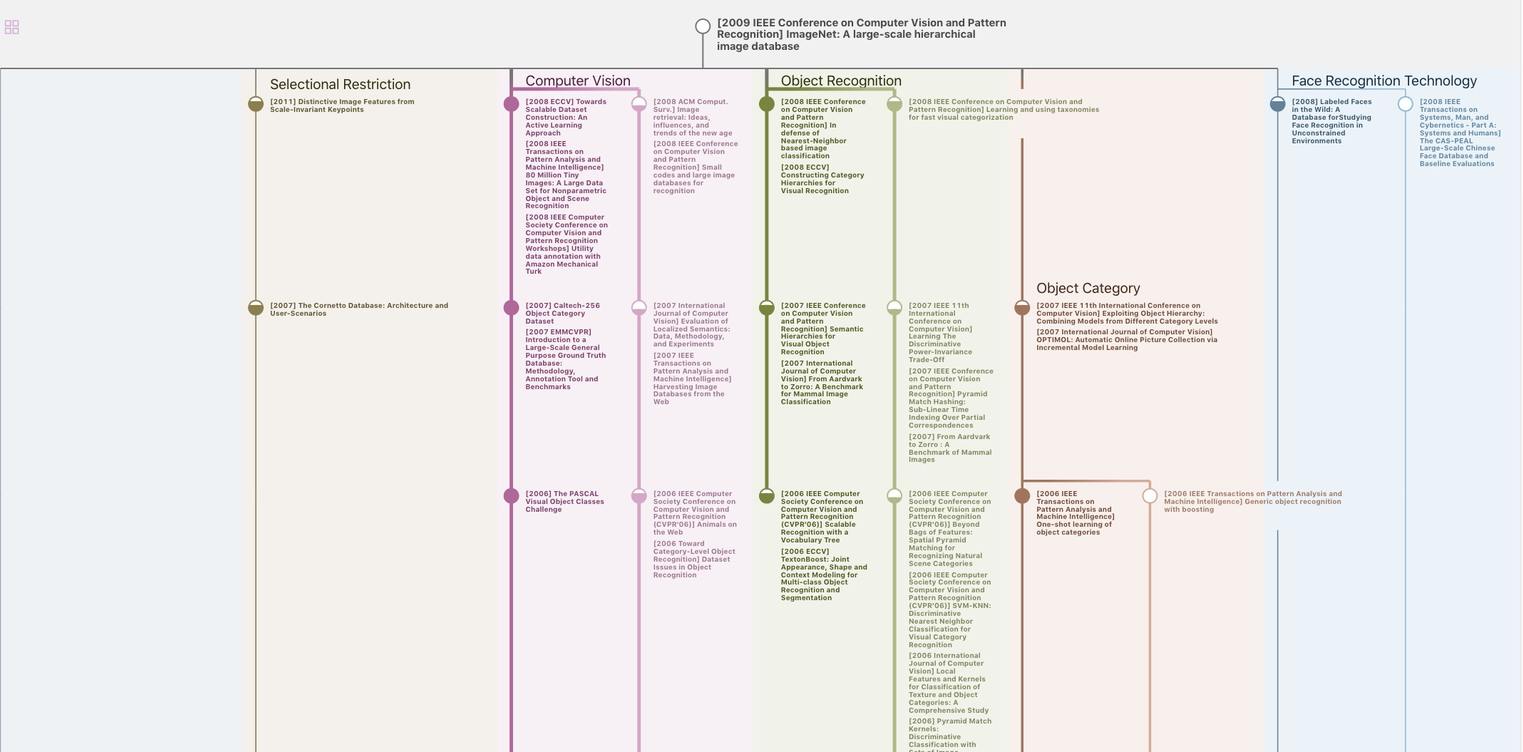
生成溯源树,研究论文发展脉络
Chat Paper
正在生成论文摘要