Graph Autoencoder Anomaly Detection for E-commerce Application by Contextual Integrating Contrast With Reconstruction and Complementarity
IEEE Transactions on Consumer Electronics(2024)
摘要
Considering the personalized characteristics and behavior patterns of users, quickly and accurately identifying abnormal users is crucial in the realm of E-commerce applications. However, existing methods fall short in integrating local and global structural information. Meanwhile, contextual information can serve effectively as a supervisory signal for anomaly detection. This paper proposes a Graph Autoencoder Anomaly Detection model, GAAD, that leverages context-integrated contrast and reconstruction complementarity. This model utilizes local information of target nodes and adopts a contrastive learning module based on graph neural networks to capture anomalies in the structure space; simultaneously, a context subgraph of the global information of the target node is constructed and anomalies in the attribute space are captured through the autoencoder-based reconstruction module. The GAAD model integrates two complementary modules, contrast and reconstruction, to enhance the accuracy of anomaly node detection. The experimental results showcase the superior performance of the proposed model over existing baseline methods across all seven benchmark datasets, thereby highlighting its efficiency in anomaly detection within E-commerce applications. Our code is available at https://github.com/jinjiewu2000/GAAD.
更多查看译文
关键词
autoencoder,graph neural network,contextual integrating,anomaly detection
AI 理解论文
溯源树
样例
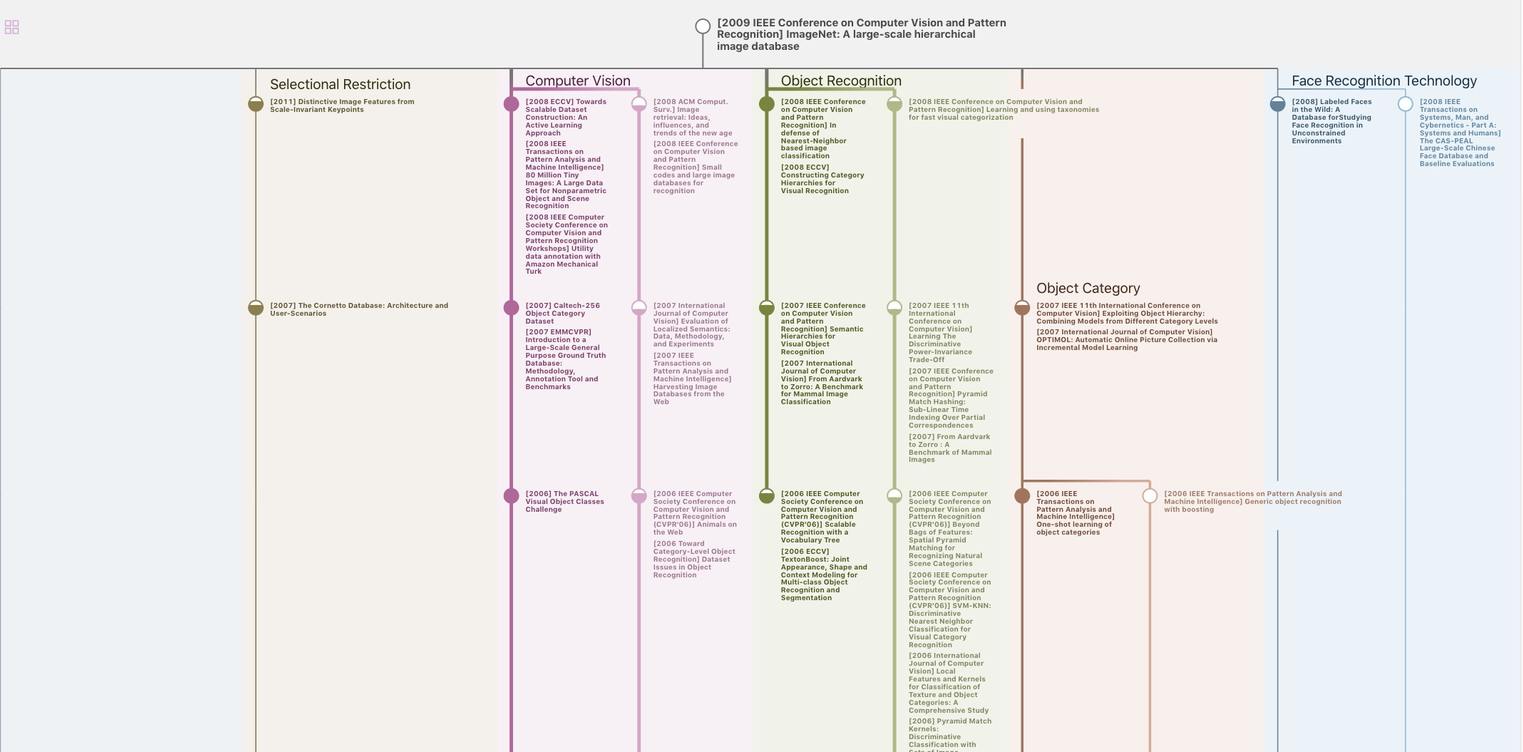
生成溯源树,研究论文发展脉络
Chat Paper
正在生成论文摘要