Decentralized State-Dependent Markov Chain Synthesis with an Application to Swarm Guidance
IEEE Transactions on Automatic Control(2024)
摘要
This paper introduces a decentralized state-dependent Markov chain synthesis (DSMC) algorithm for finite-state Markov chains. We present a state-dependent consensus protocol that achieves exponential convergence under mild technical conditions, without relying on any connectivity assumptions regarding the dynamic network topology. Utilizing the proposed consensus protocol, we develop the DSMC algorithm, updating the Markov matrix based on the current state while ensuring the convergence conditions of the consensus protocol. This result establishes the desired steady-state distribution for the resulting Markov chain, ensuring exponential convergence from all initial distributions while adhering to transition constraints and minimizing state transitions. The DSMC's performance is demonstrated through a probabilistic swarm guidance example, which interprets the spatial distribution of a swarm comprising a large number of mobile agents as a probability distribution and utilizes the Markov chain to compute transition probabilities between states. Simulation results demonstrate faster convergence for the DSMC based algorithm when compared to the previous Markov chain based swarm guidance algorithms.
更多查看译文
关键词
Markov Chains,Consensus Protocol,Decentralized Control,Probabilistic Swarm Guidance
AI 理解论文
溯源树
样例
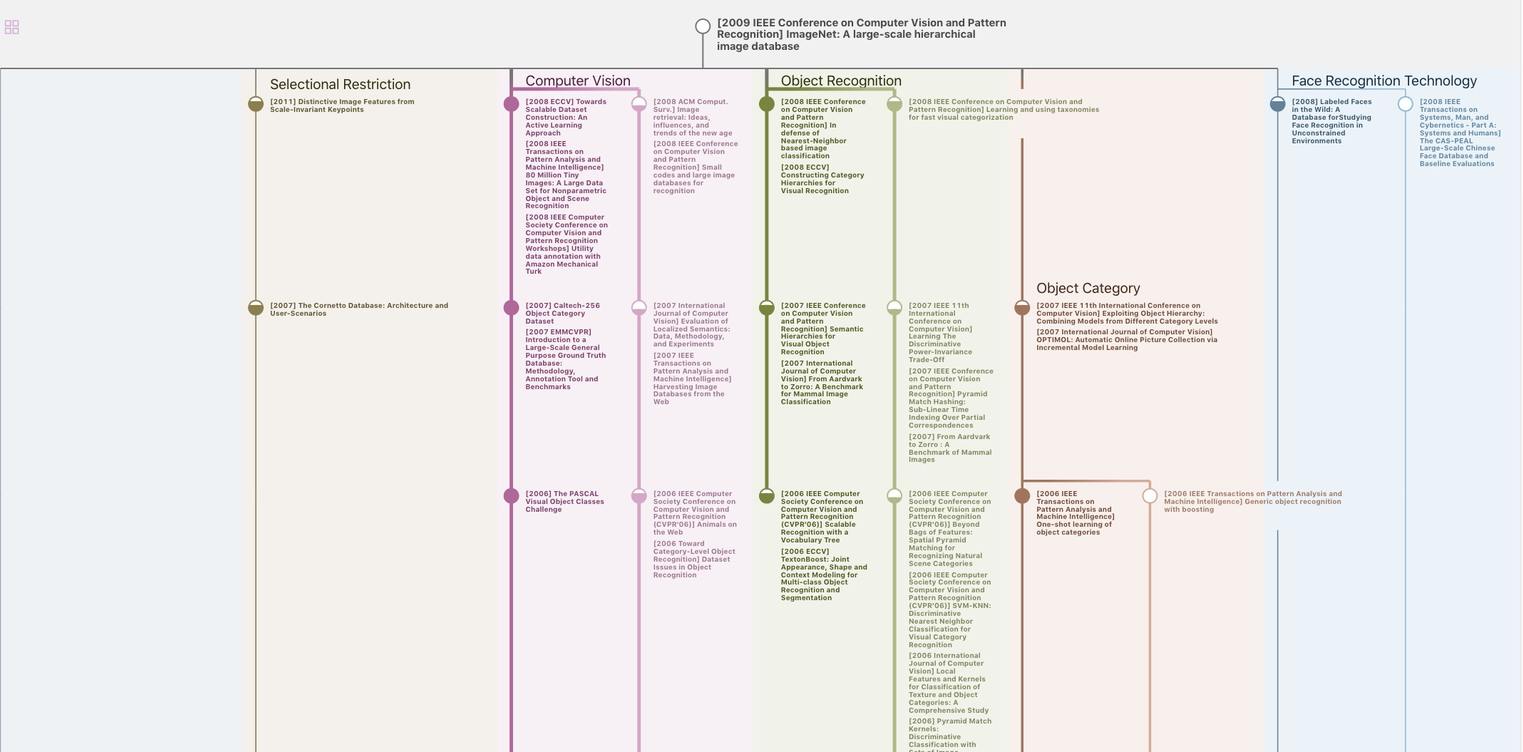
生成溯源树,研究论文发展脉络
Chat Paper
正在生成论文摘要