Robust Weighted Low-Rank Tensor Approximation for Multiview Clustering With Mixed Noise
IEEE TRANSACTIONS ON COMPUTATIONAL SOCIAL SYSTEMS(2023)
摘要
Multiview clustering performs grouping a set of objects by utilizing complementary information from multiple views. Unfortunately, the clustering performance degenerates dramatically if the views are corrupted by noise. To overcome this limitation, we propose a robust multiview clustering approach based on weighted low-rank tensor approximation and noise separation. The proposed model improves the performance through a low-rank approximation function and weighted singular values. The weighted low-rank tensor approximation method considers both prior knowledge and the physical meanings associated with different singular values, leading to superior performance in capturing high-order correlations. Additionally, to eliminate mixed noise, a novel l(Cauchy,1) norm is developed to handle outliers, and the l(1) and Frobenius norms are used to handle random corruptions and slight perturbations, respectively. A high-efficiency optimization algorithm based on the alternating direction method of multipliers (ADMM) is designed to address the challenging proposed model. Experimental results on nine real-world datasets show that the proposed approach outperforms eight state-of-the-art multiview methods. Furthermore, experiments on various kinds of noise demonstrate the superior robustness of the proposed approach. Especially, in the mixed noise condition, the proposed approach is significantly superior to other methods.
更多查看译文
关键词
Low-rank tensor,noise handling,robust multiview clustering,weighted singular values
AI 理解论文
溯源树
样例
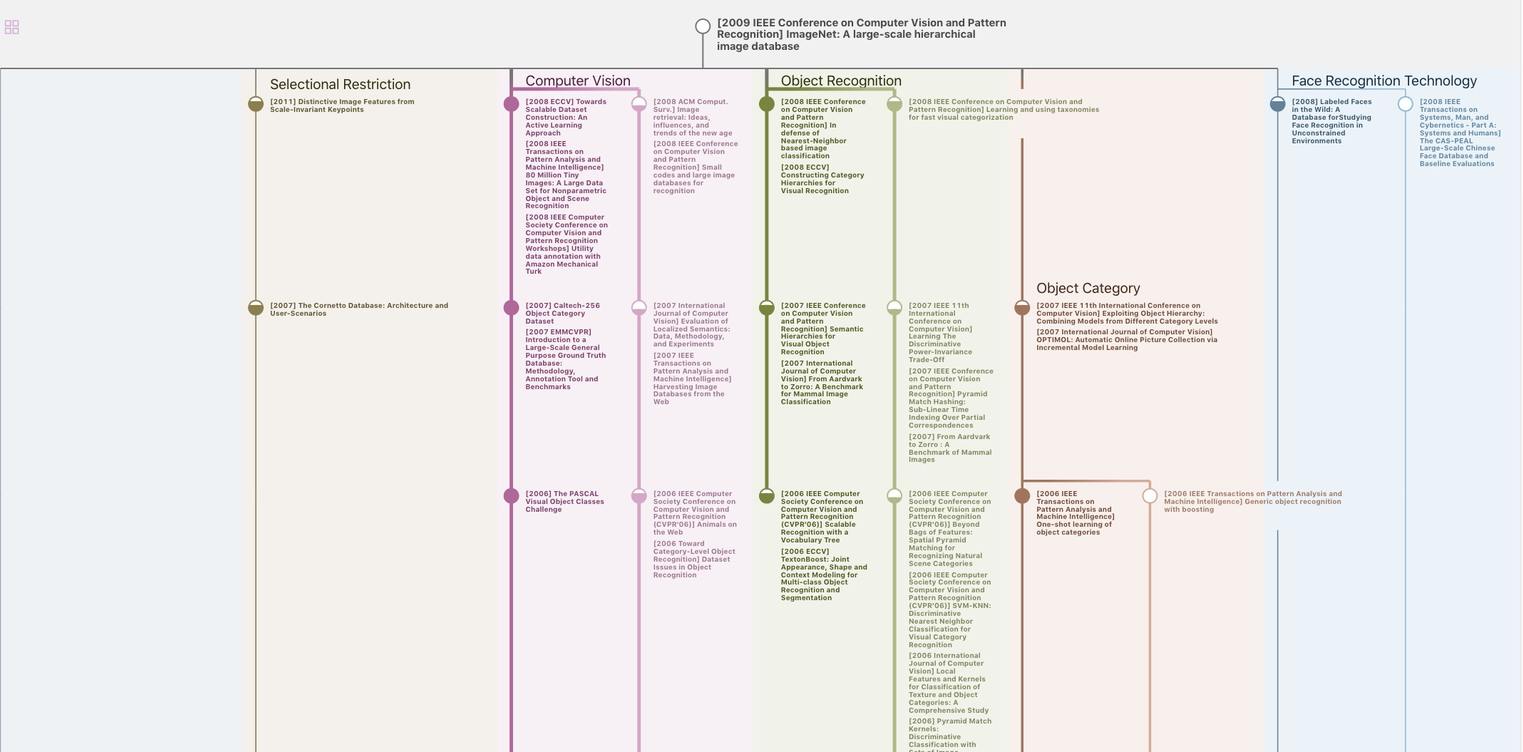
生成溯源树,研究论文发展脉络
Chat Paper
正在生成论文摘要