MSDGSD: A Scalable Graph Descriptor for Processing Large Graphs
IEEE TRANSACTIONS ON COMPUTATIONAL SOCIAL SYSTEMS(2024)
摘要
Graph representation methods have recently become the de facto standard for downstream machine learning tasks on graph-structured data and have found numerous applications, e.g., drug discovery & development, recommendation, and forecasting. However, the existing methods are specially designed to work in a centralized environment, which limits their applicability to small or medium-sized graphs. In this work, we present a graph embedding method that extracts graph representations in a distributed environment with independent and parallel machines. The proposed method is built-upon the existing approach, distributed graph statistical distance (DGSD), to enhance the scalability on large graphs. The key innovation of our work lies in the proposition of a batching mechanism for client-server message passing, which reduces communication overhead during the computation of the distance matrix. In addition, we present a sampling approach for computing pairwise distances between the nodes to compute the desired graph embedding. Moreover, we systematically explore six distinct variations of a distributed graph embeddings and subsequently subject them to comprehensive evaluation. Our extensive evaluations on over 20 graph datasets and ten baseline methods demonstrate improved running time and comparative classification accuracy compared to state-of-the-art embedding techniques.
更多查看译文
关键词
Distributed computing,graph classification,graph embedding,parallel processing
AI 理解论文
溯源树
样例
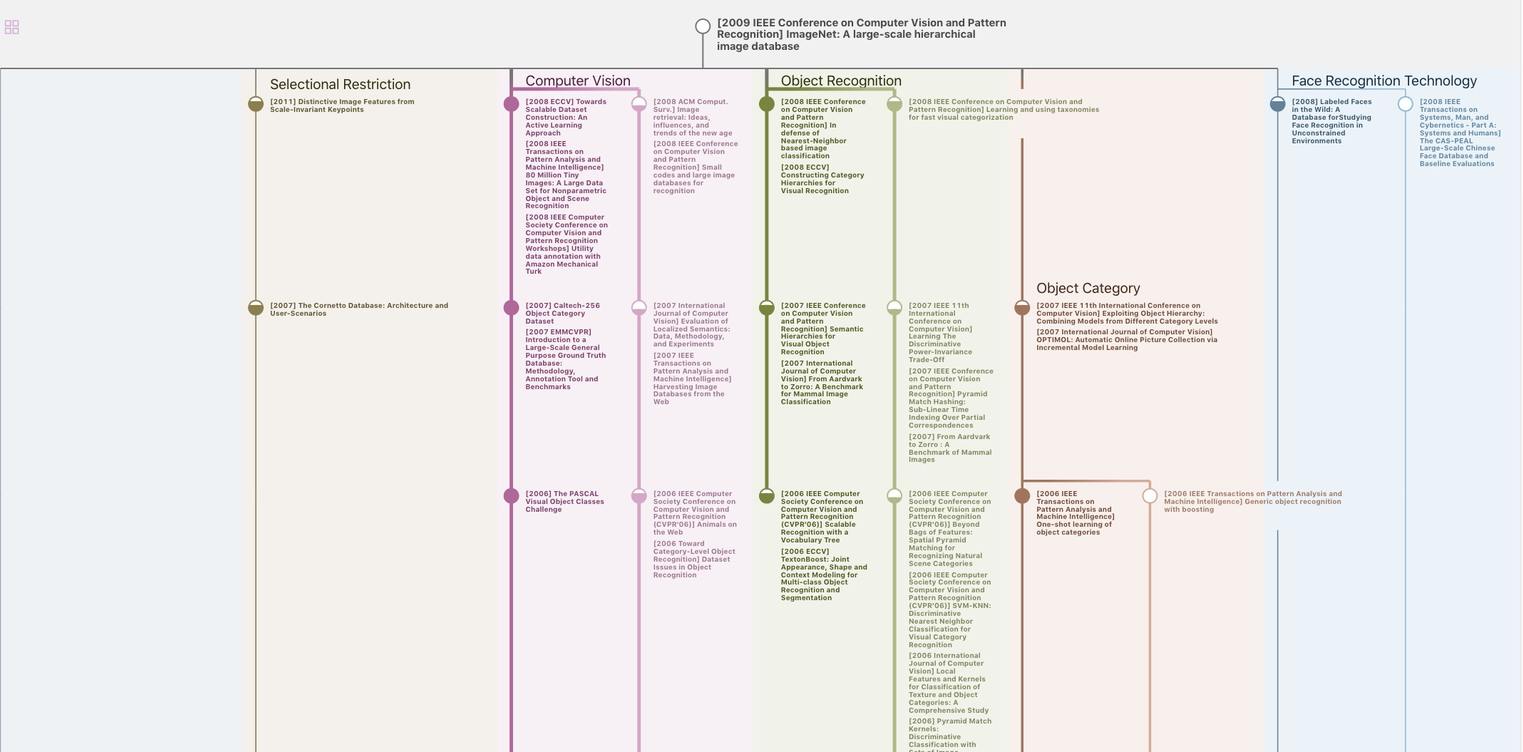
生成溯源树,研究论文发展脉络
Chat Paper
正在生成论文摘要