Health Assessment of Solid Insulation of Mineral Oil-filled Converter Transformer
IEEE Transactions on Dielectrics and Electrical Insulation(2023)
摘要
The furan prediction in the transformer oil can provide information about the oil degradation rate and overall health of the oil-filled transformer. For this study, the furan content in mineral oil of a 300 MVA converter transformer installed at ±500 kV HVDC inverter station is taken. Six features are taken as input to the machine learning model to predict the furan content for predictive maintenance of the converter transformer, i.e., carbon monoxide, carbon-di-oxide, acidity, breakdown voltage, total combustible gases, and water content. A hybrid ML model is developed consisting of a convolutional neural network-long short-term memory network (CNN-LSTM) and extreme gradient boosting (XGBoost). Then, the mean absolute percentage error with recursive weighting (MAPE-RW) technique is employed to combine the predictions of two models to achieve better outcomes than a single model. For this purpose, a total of 350 data are collected from the local utilities for training and testing. Moreover, a total of 41 numbers of data are collected from the converter transformer for validation of the proposed model. The performance of the proposed algorithm is evaluated by mean absolute error, root means squared error, and coefficient of determination. The hybrid model is also compared with other models to show its efficacy and robustness. Depending upon the furan prediction, converter transformer insulation health is classified into three classes for preventive maintenance.
更多查看译文
关键词
Oil insulation,machine learning,power transformers,regression analysis
AI 理解论文
溯源树
样例
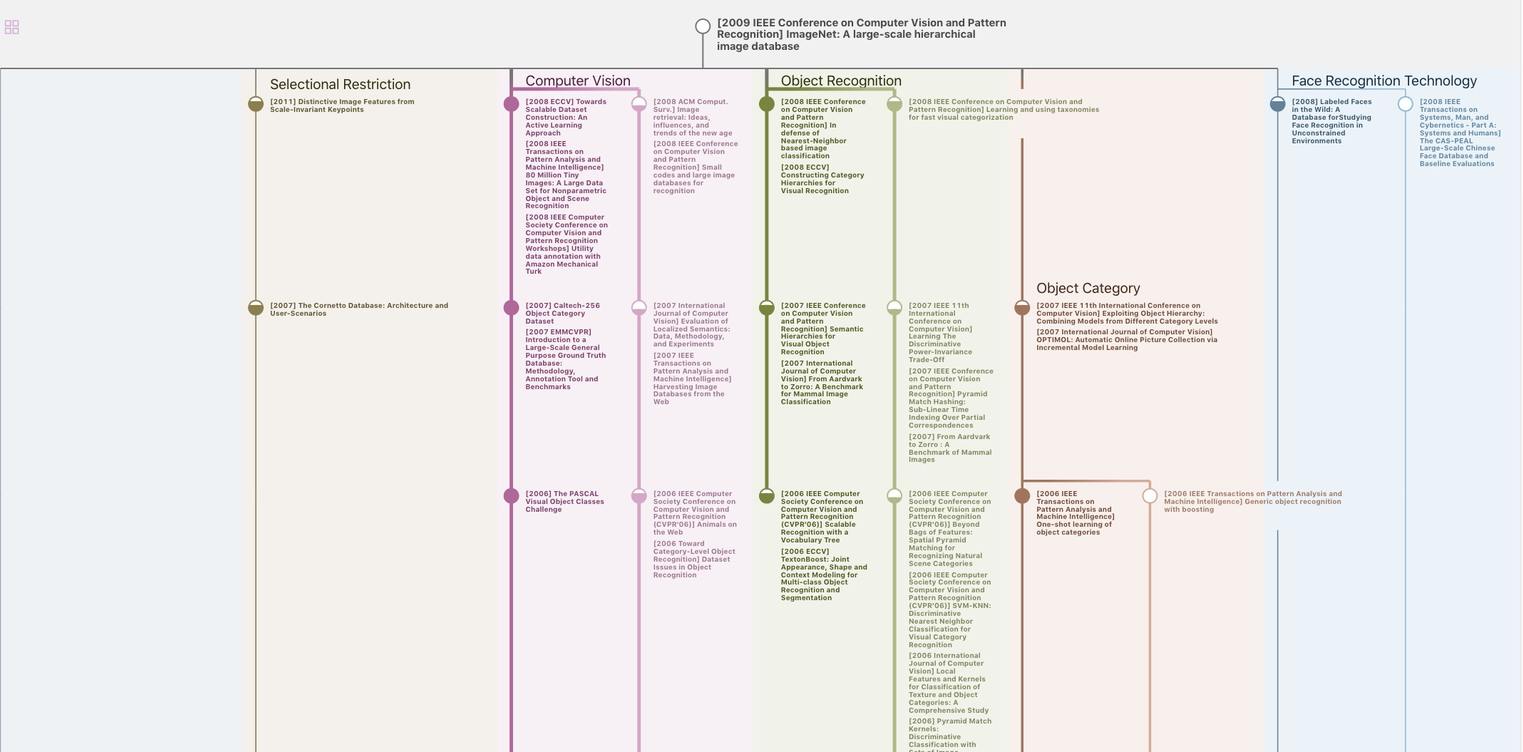
生成溯源树,研究论文发展脉络
Chat Paper
正在生成论文摘要