Dynamic Hybrid Models With Active Sampling and Adaptive Selection of Double-Domain Features for the Tuning of Microwave Cavity Filters
IEEE TRANSACTIONS ON CYBERNETICS(2024)
摘要
Microwave cavity filters are essential electromechanical coupling devices in communication systems. Structural-parameter tuning by experienced operators improves the filter performance but is demanding and time-consuming. The automatic tuning method has received extensive research attentions using data-driven modeling approaches. However, two main issues affect the accuracy and efficiency of the model construction: 1) features of tuning processes, as model inputs, have limited adaptability and extraction accuracy to different resonant states and 2) models require plentiful training data and the training process is time-consuming. Thus, dynamic hybrid models are developed in this study with self-selected inputs, self-organized samples, and a self-learning structure. First, spatial features are extracted to flexibly depict the tuning characteristic, and double-domain (spatial or circuital) features are selected adaptively to accommodate distinct resonance states. Second, a trustworthiness-curiosity-driven active sampling method is exploited to attain fewer and better-training data. Third, an improved broad learning systemBLS is developed using new modules of incremental node calculation and weight pruning, characterized by more lightweight and flexible structures. The proposed method is effective and flexible demonstrated by simulations and experiments, and the tuning task of microwave cavity filters is fulfilled in a more accurate and efficient manner.
更多查看译文
关键词
Active sampling,adaptive feature selection,broad learning systems,incremental learning,intelligent tuning,microwave cavity filters
AI 理解论文
溯源树
样例
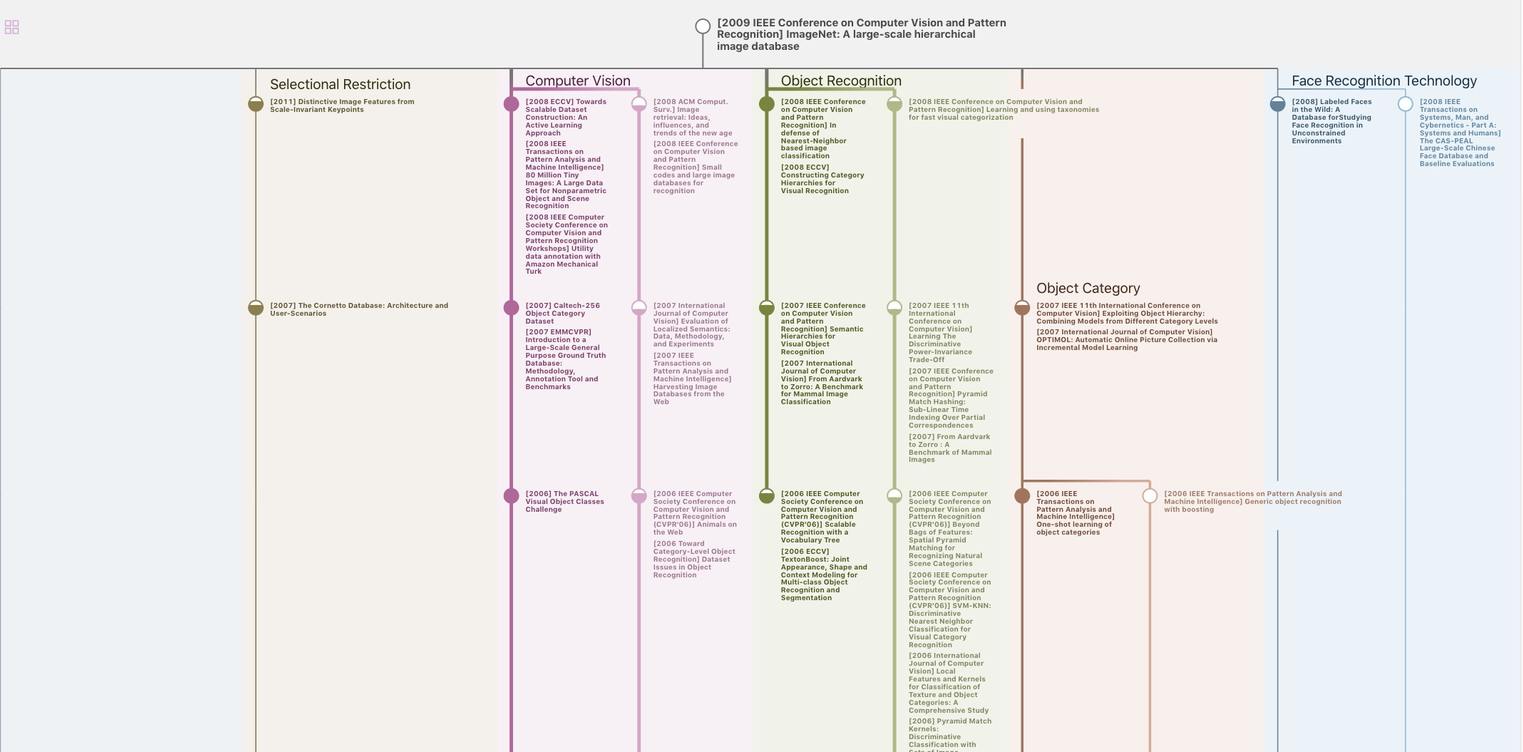
生成溯源树,研究论文发展脉络
Chat Paper
正在生成论文摘要