Distributed Primal-Dual Proximal Algorithms for Convex Optimization Involving Three Composite Functions
IEEE TRANSACTIONS ON CONTROL OF NETWORK SYSTEMS(2024)
摘要
This article develops a novel distributed primal-dua l proximal algorithm (PDPA-Dist) and its corresponding randomized version (Rand-PDPA-Dist) for solving convex optimization problems. The local objective consists of a smooth function and two possibly nonsmooth ones, with one involving a linear mapping. At each iteration, PDPA-Dist allows each agent to perform local computations by communicating with its neighbors, while Rand-PDPA-Dist is executed with a randomly and independently activated subset of agents. The proposed algorithms recover new distributed extensions of known centralized algorithms and existing distributed schemes, depending on certain special setups. Both PDPA-Dist and Rand-PDPA-Dist achieve exact linear convergence rates provided that local objective functions satisfy piecewise linear-quadratic or certain quadratic growth conditions. Finally, the performance and effectiveness of the proposed algorithms are demonstrated by resolving a distributed model predictive control (DMPC) problem.
更多查看译文
关键词
Composite functions,distributed convex optimization,linear convergence,primal-dual proximal algorithms,randomized algorithm
AI 理解论文
溯源树
样例
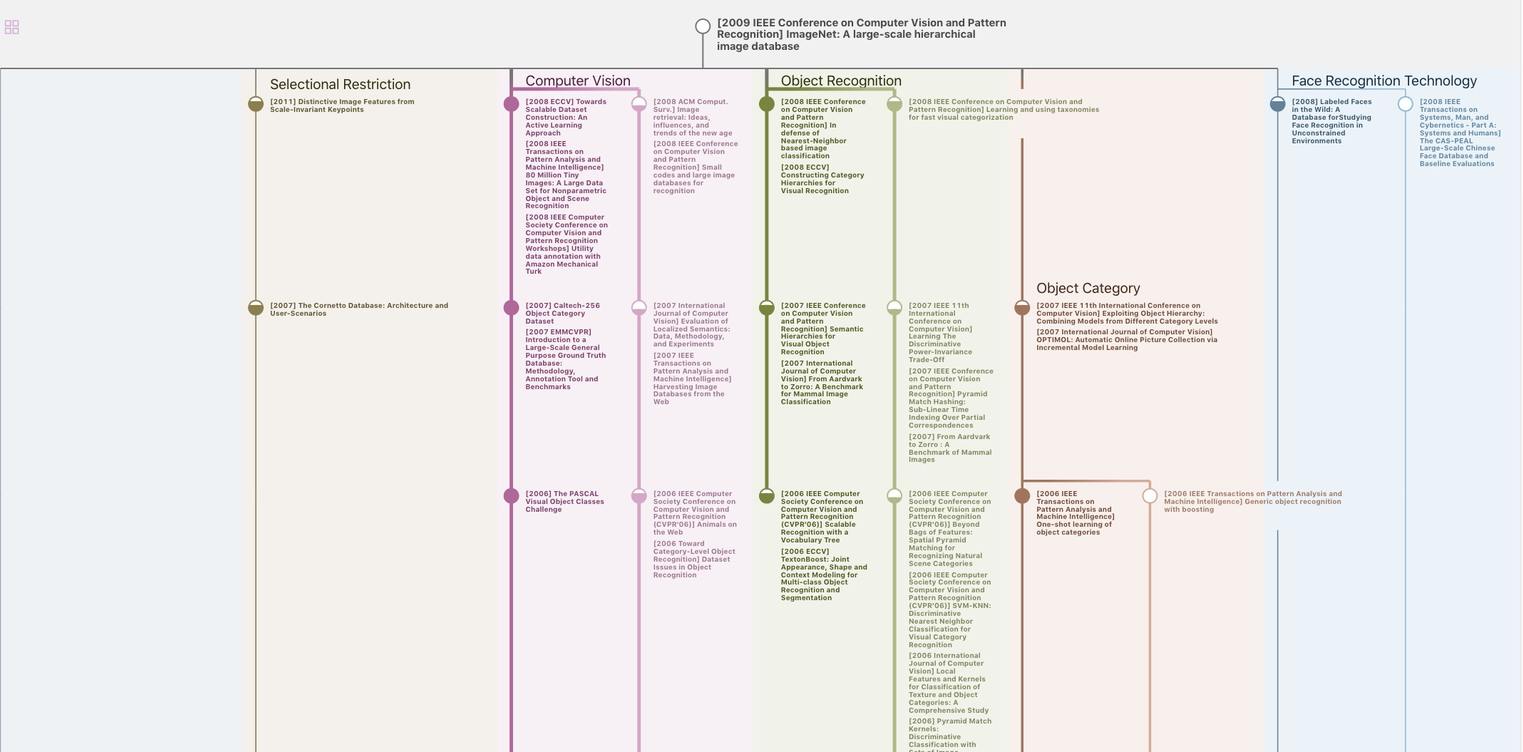
生成溯源树,研究论文发展脉络
Chat Paper
正在生成论文摘要