An End-to-End In Memory Computing System Based On A 40nm eFlash-Based IMC SoC: Circuits, Toolchains, and Systems Co-Design Framework
IEEE Transactions on Computer-Aided Design of Integrated Circuits and Systems(2024)
摘要
Despite its promising potential for Artificial Intelligence (AI) applications, current In-Memory Computing (IMC) technology faces a variety of challenges before mass production. One of the major challenges we face is the absence of efficient toolchains for deploying canonical networks on IMC chips. To address this issue, we propose a co-designed framework that integrates circuit, toolchain, and system elements specifically for IMC. More specifically, our framework consists of several key techniques to improve the key performance including (a) an 8-bit hardware-friendly Quantization-Aware Training (QAT) approach to quantify the deep learning network from floating-point data to fixed-point data, (b) a novel operator optimization technique to increase the computing precision when running the algorithm models on the IMC chips, and (c) an efficient mapping strategy based on the Integer Linear Programming (ILP) approach to improve the computation resource utilization of the IMC array. We assess our method on our 40nm eFlash-based IMC SoC chip with voice recognition, speech noise reduction, and person detection tasks. Our experimental results show an accuracy over 94.60% in a quiet environment and 87.27% in a white noise environment and a false recognition rate below 1 time per 24 hours for voice recognition, a 21.53% improvement for the Perceptual Evaluation of Speech Quality (PESQ) for noise reduction, and a 97.80% accuracy in person detection.
更多查看译文
关键词
In-memory computing,eFlash,toolchains,circuit-toolchain-system co-design framework
AI 理解论文
溯源树
样例
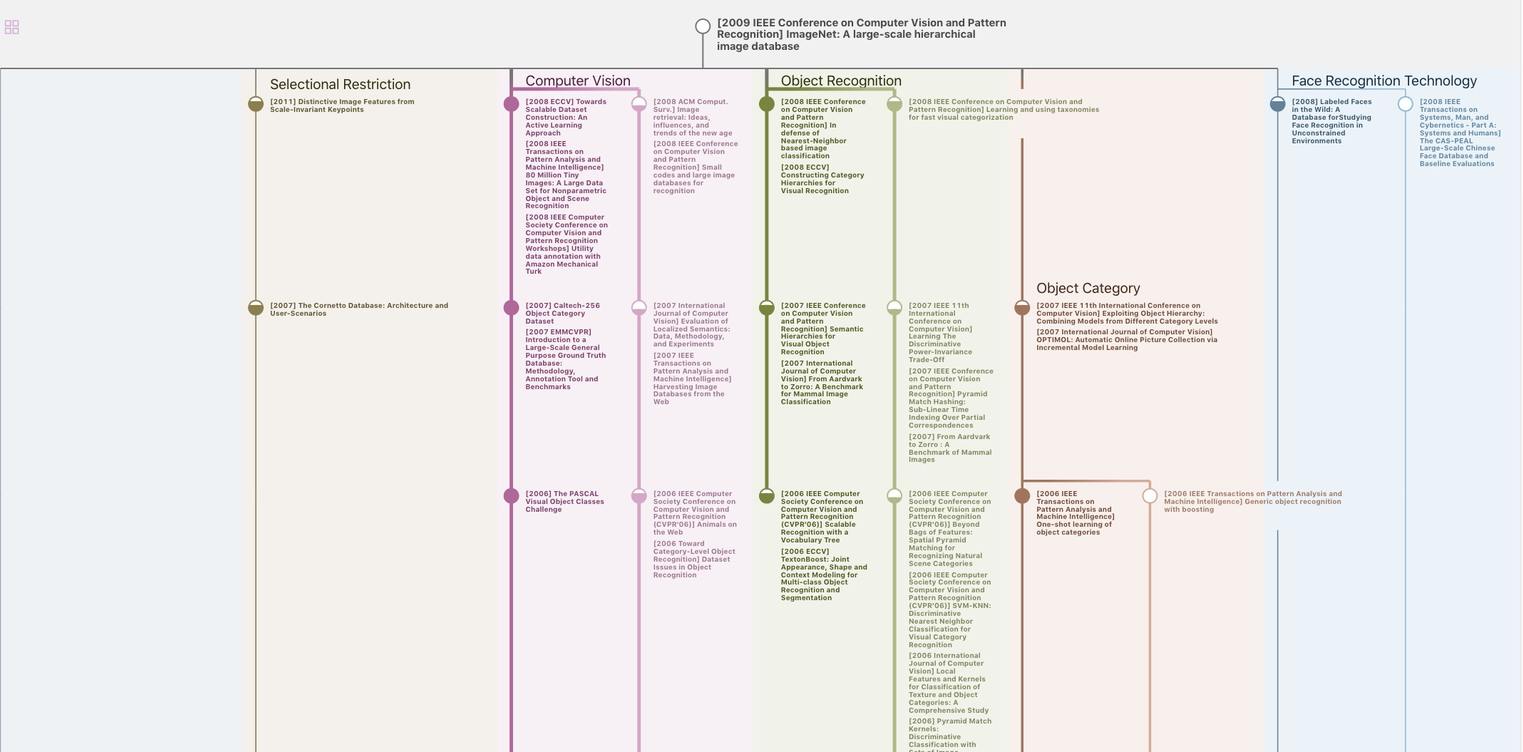
生成溯源树,研究论文发展脉络
Chat Paper
正在生成论文摘要