A Lightweight DRDPG-Based RL DVFS for Video Rendering on CPU-GPU Integrated SoC
IEEE TRANSACTIONS ON CIRCUITS AND SYSTEMS I-REGULAR PAPERS(2024)
摘要
Reinforcement learning (RL) based dynamic voltage and frequency scaling (DVFS) is an effective approach to balance performance and power consumption for video rendering applications. To approximate the "God's eye view" regulation, the CPU-GPU should be fine-grained regulated and the SoC have to be fully observed by a RL-based DVFS governor. Fine-grained regulation with traditional value-based RL governor suffers action space explosion and it is impossible to have a fully observable SoC. To address these two issues, a governor based on deep recurrent deterministic gradient (DRDPG) governor is proposed. The governor is based on Deterministic Policy Gradient (DDPG) algorithm with embedded recurrent neural network (RNN). The DDPG algorithm guarantees fine-grained power regulation without action space explosion and the RNN-FC network topology mitigates the partial observability issue. Evaluated on the Nvidia Jetson NX platform, the proposed DRDPG governor achieves over 19% better regulation efficiency compared with Linux default governors and shows superior regulation efficiency to other RL-based state-of-the-arts. Implemented in a 55-nm CMOS process, the proposed governor draws merely 2.16-mA from a 1.2-V supply at 1-MHz clock occupying a silicon area of 0.075mm(2).
更多查看译文
关键词
SoC,DVFS,RL,video rendering
AI 理解论文
溯源树
样例
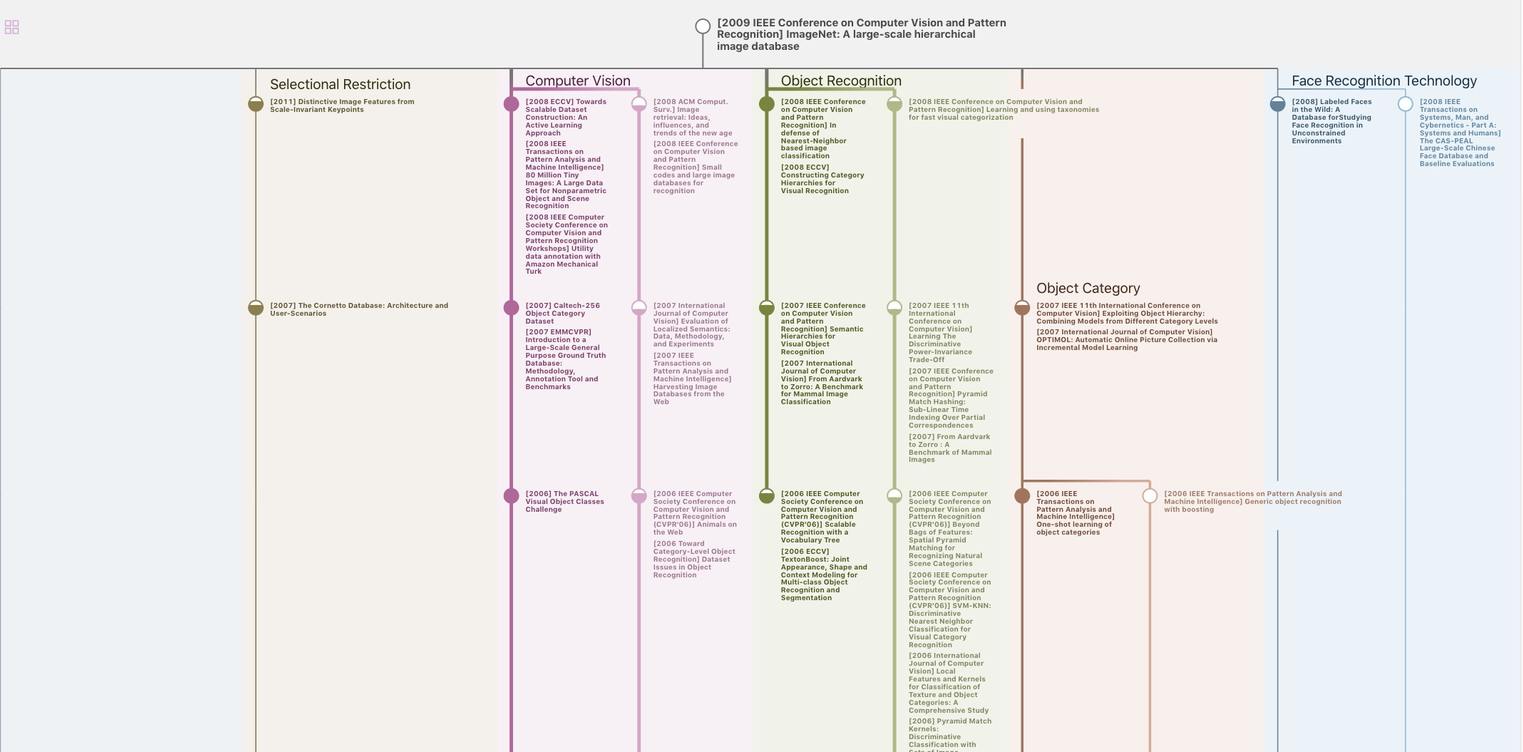
生成溯源树,研究论文发展脉络
Chat Paper
正在生成论文摘要