Fixed-Time Neurodynamic Optimization Algorithms and Application to Circuits Design
IEEE TRANSACTIONS ON CIRCUITS AND SYSTEMS I-REGULAR PAPERS(2024)
摘要
In this article, several fixed-time (FT) neurodynamic algorithms with time-varying coefficients are introduced for composite optimization problems. The remarkable features of neurodynamic algorithms are FT convergence from arbitrary initial conditions with faster convergence rate by choosing different time-varying coefficients. The FT convergence of neurodynamic algorithms can be proved by the Polyak- ${\L}$ ojasiewicz condition, which is beyond strong convexity condition. The upper bounds of the settling time for time-varying neurodynamic algorithms are explicitly given. The robustness of neurodynamic algorithms under bounded noises are further studied. In addition, the proposed neurodynamic algorithms are also utilized for dealing with absolute value equations and sparse signal reconstruction problems. The circuit framework for FT neurodynamic algorithms is subsequently introduced, and an example simulated in Multisim 14.3 is provided to verify the practicability of the proposed analog circuits. Numerical experiments on image recovery and sparse logistic regression are conducted to validate the superiority of the proposed algorithms.
更多查看译文
关键词
Neurodynamic algorithms,fixed-time convergence,composite optimization problems,robustness analysis,circuit implementation
AI 理解论文
溯源树
样例
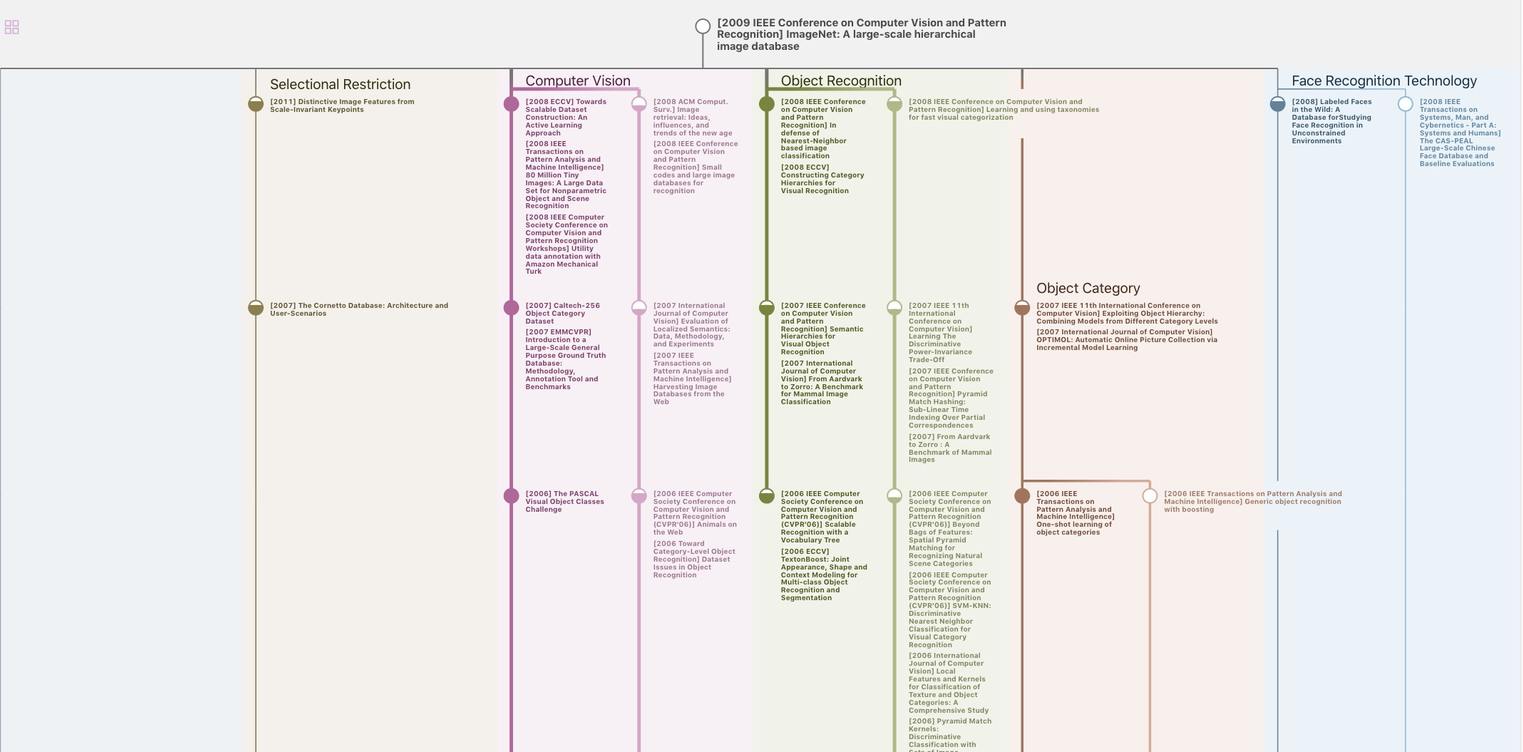
生成溯源树,研究论文发展脉络
Chat Paper
正在生成论文摘要