Dual-GCL: Dual-Graph Contrastive Learning for Unsupervised Person Re-Identification
IEEE Transactions on Cognitive and Developmental Systems(2024)
摘要
Unsupervised person re-identification (re-ID) aims to perform person retrieval in an unsupervised manner across multiple cameras. Most recent works usually adopted contrastive learning with clustering strategy to guide learning process. However, the domain gap between cameras easily causes the high intra-class variance and prohibits an effective contrastive learning. To overcome this problem, we propose dual-graph contrastive learning (Dual-GCL) to fully utilize the structures between the instances as well as the relationship between the instances and the centroids generated by the clustering strategy. Firstly, a dual-graph is constructed by utilizing the affinities of instances in the feature space and the pseudo-label space derived from clustering strategy with the node-to-node contrastive learning. Then, to capture the relations between the dual-graph and the centroids, we perform dual-graph contrastive learning at both the node level and the super-node level. The contrastive learning based on the two levels can encode multi-level structural representations between the instances corresponding to the nodes in dual-graph and align the centroids between different cameras in the shared embedding space. Moreover, a camera-aware augmentation scheme that exploits the semantic relationship is proposed to alleviate the domain discrepancy between cameras. Experimental results demonstrate that our approach outperforms the state-of-the-art methods.
更多查看译文
关键词
Person re-identification,unsupervised learning,contrastive learning
AI 理解论文
溯源树
样例
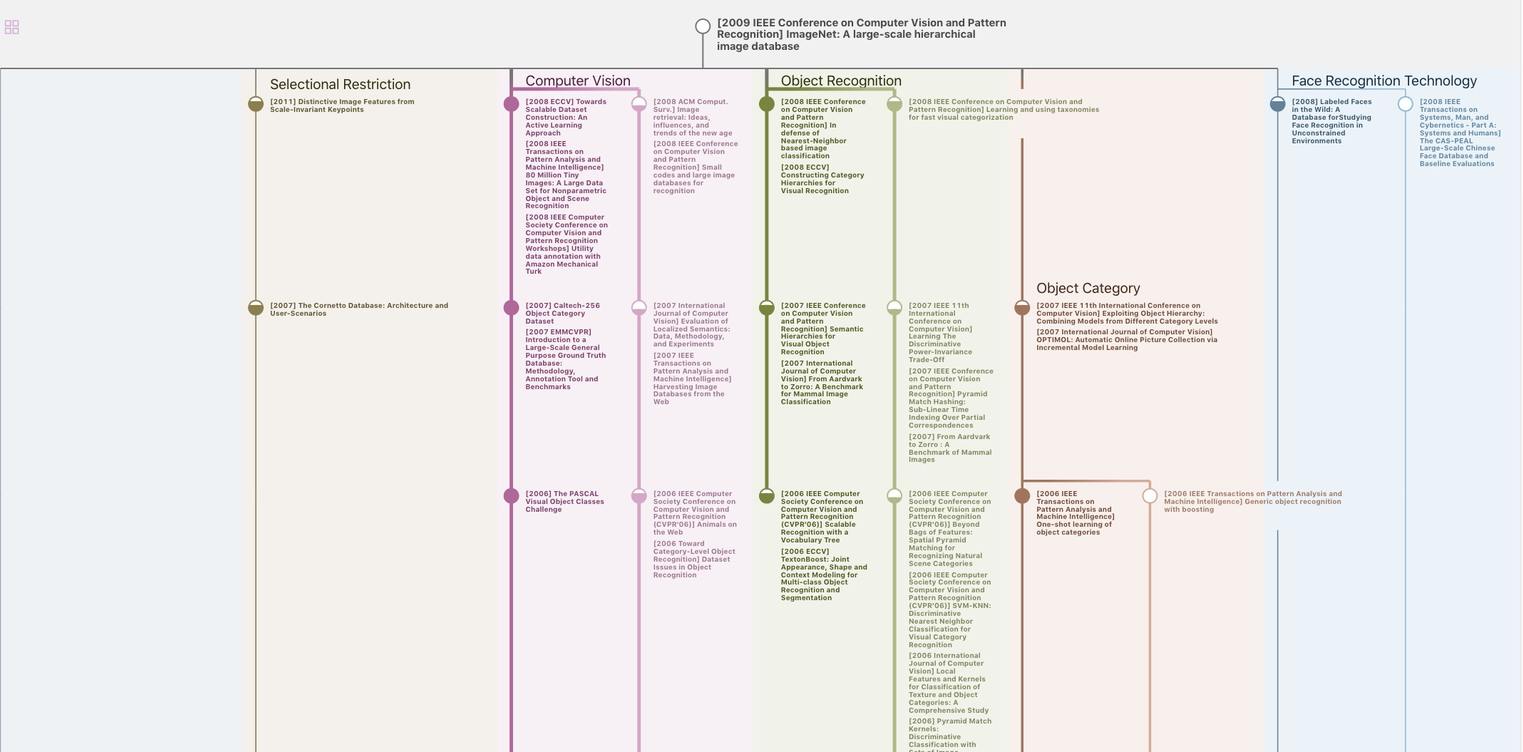
生成溯源树,研究论文发展脉络
Chat Paper
正在生成论文摘要