TR-TransGAN: Temporal Recurrent Transformer Generative Adversarial Network for Longitudinal MRI Dataset Expansion
IEEE Transactions on Cognitive and Developmental Systems(2024)
摘要
Longitudinal MRI datasets have important implications for the study of degenerative diseases because such datasets have data from multiple points in time to track disease progression. However, longitudinal datasets are often incomplete due to unexpected quits of patients. In previous work, we proposed an augmentation method TR-GAN that can complement missing session data of MRI datasets. TR-GAN uses a simple U-Net as a generator, which limits its performance. Transformers have had great success in the research of computer vision and this paper attempts to introduce it into longitudinal dataset completion tasks. The multi-head attention mechanism in Transformer has huge memory requirements, and it is difficult to train 3D MRI data on GPUs with small memory. To build a memory-friendly Transformer-based generator, we introduce a Hilbert transform module (HTM) to convert 3D data to 2D data that preserves locality fairly well. To make up for the insufficiency of CNN-based models that are difficult to establish long-range dependencies, we propose an up/down sampling (STU/STD) module that combines the Swin Transformer module and CNN module to capture global and local information simultaneously. Extensive experiments show that our model can reduce MMSE by at least 7.16% compared to the previous state-of-the-art method.
更多查看译文
关键词
Magnetic Resonance Imaging,Generative Adversarial Network,Transformer,Longitudinal Dasaset
AI 理解论文
溯源树
样例
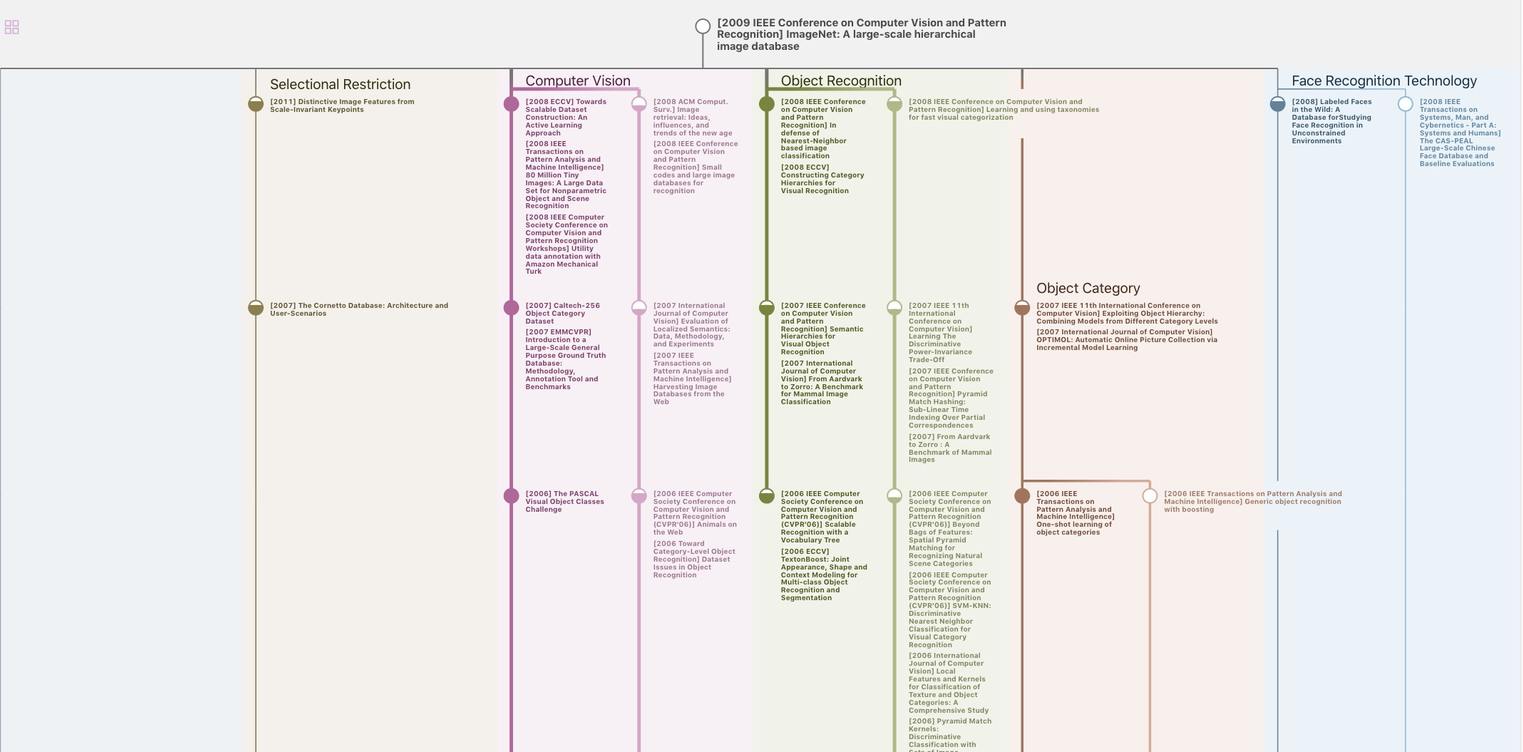
生成溯源树,研究论文发展脉络
Chat Paper
正在生成论文摘要