Web API Recommendation via Combining Adaptive Multi-channel Graph Representation and xDeepFM Quality Prediction
IEEE Transactions on Artificial Intelligence(2023)
摘要
With the increasing number of Web services, how to provide developers with Web APIs that meet their Mashup requirements accurately and efficiently has become a challenge. Even though the existing methods show improvements in service recommendation, the efficiency and accuracy of them still need to be improved due to their limited representation in fusing network topology and node feature of Web service, and the neglected higher-order feature interactions of Web service. To address this problem, this paper proposes a Web APIs recommendation method via combining adaptive multichannel graph representation and xDeepFM (eXtreme Deep Factorization Machine) quality prediction. In this method, firstly, specific embedding and shared embedding in Web API node isomorphic network are extracted from the nodes’ feature space, topology space, and the combination of the two spaces. Then, attention mechanism is used to adaptively learn the importance weight of each embedding. Next, these embeddings are adaptively integrated to generate the multi-channel graph representation of Web APIs for service classification. Finally, aiming at the Web APIs in the service cluster, it utilizes xDeepFM to model and mine the complex feature interactions and predict and rank the scores of Web APIs for Mashup creation. The experimental results on the real datasets of ProgrammableWeb show that compared with DeepFM, WDL, FNN, NFM, and MLR, the method proposed in this paper has an average improvement in AUC of 2.3%, 7.9%, 8.0%, 9.6%, and 13.3%.
更多查看译文
关键词
Web APIs,Mashup,Web API Recommendation,Graph Representation,xDeepFM
AI 理解论文
溯源树
样例
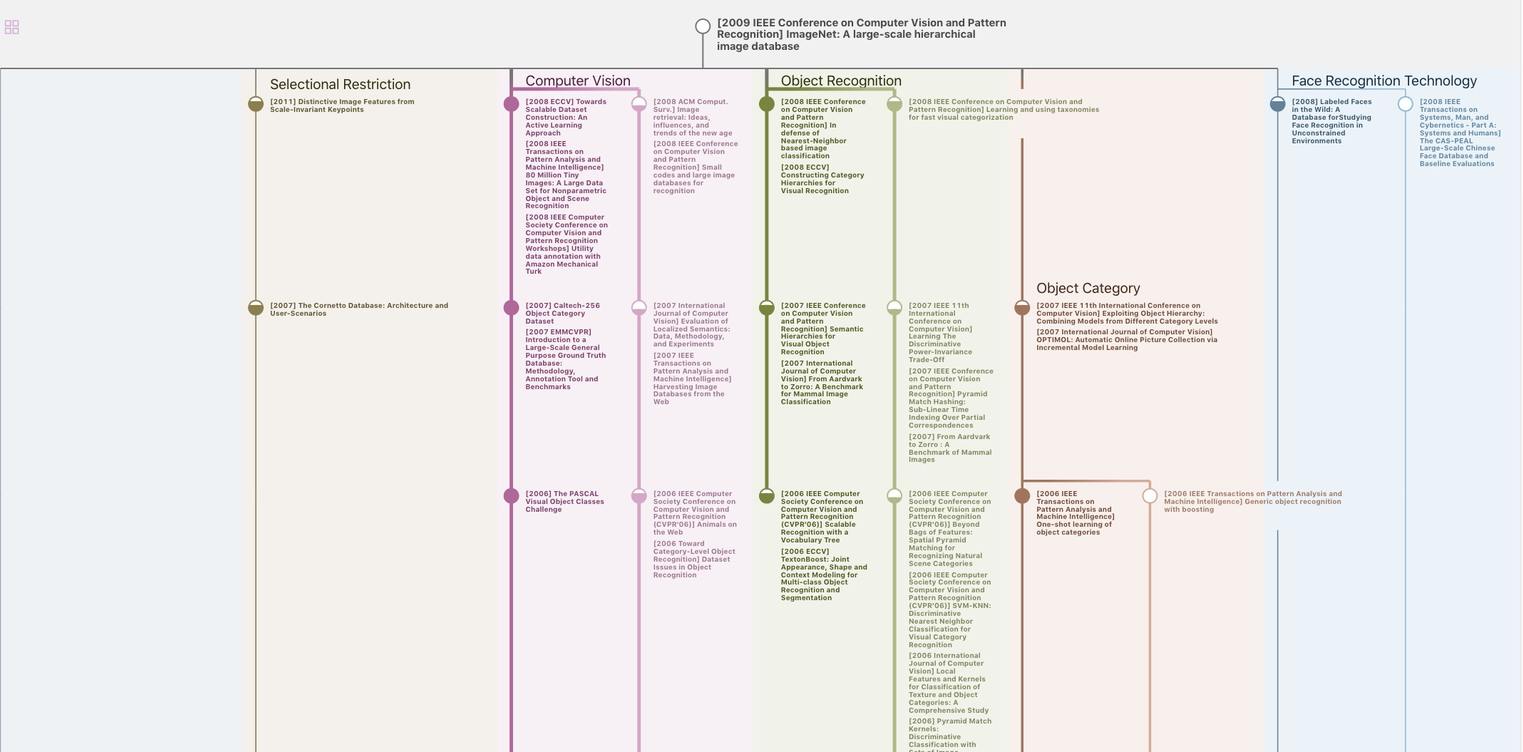
生成溯源树,研究论文发展脉络
Chat Paper
正在生成论文摘要