Additive Noise Model Structure learning Based on Spatial Coordinates
IEEE Transactions on Artificial Intelligence(2024)
摘要
Discovering causal relationships from a large amount of observational data is an important research direction in data mining. To address the challenges of discovering and constructing causal networks on nonlinear and high-dimensional data, this paper proposes a new structural learning algorithm called SCB. The SCB algorithm effectively discovers causal networks from large-scale nonlinear data. In this paper, we make three main contributions. Firstly, based on the Hilbert Schmidt Independence Criterion (HSIC), we propose new independence test coefficients: SC (Spatial Coordinate Coefficient) and CSC (Conditional Spatial Coordinate Coefficient). We also prove that the statistical distribution of the CSC coefficient follows a standard normal distribution. Secondly, using the statistical distribution of the CSC coefficient, we redefine the correlation of variables and combine it with local learning to propose the SCB algorithm. Finally, we demonstrate the effectiveness of the algorithm through experiments on data generated from various nonlinear function dependencies. Compared to existing algorithms, the causal network models constructed using the SCB algorithm exhibit significant improvements in accuracy and time performance. The effectiveness of the proposed method is further validated on real power plant data.
更多查看译文
关键词
Nonlinear,Additive Noise Model,Bayesian Network,Spatial Coordinates
AI 理解论文
溯源树
样例
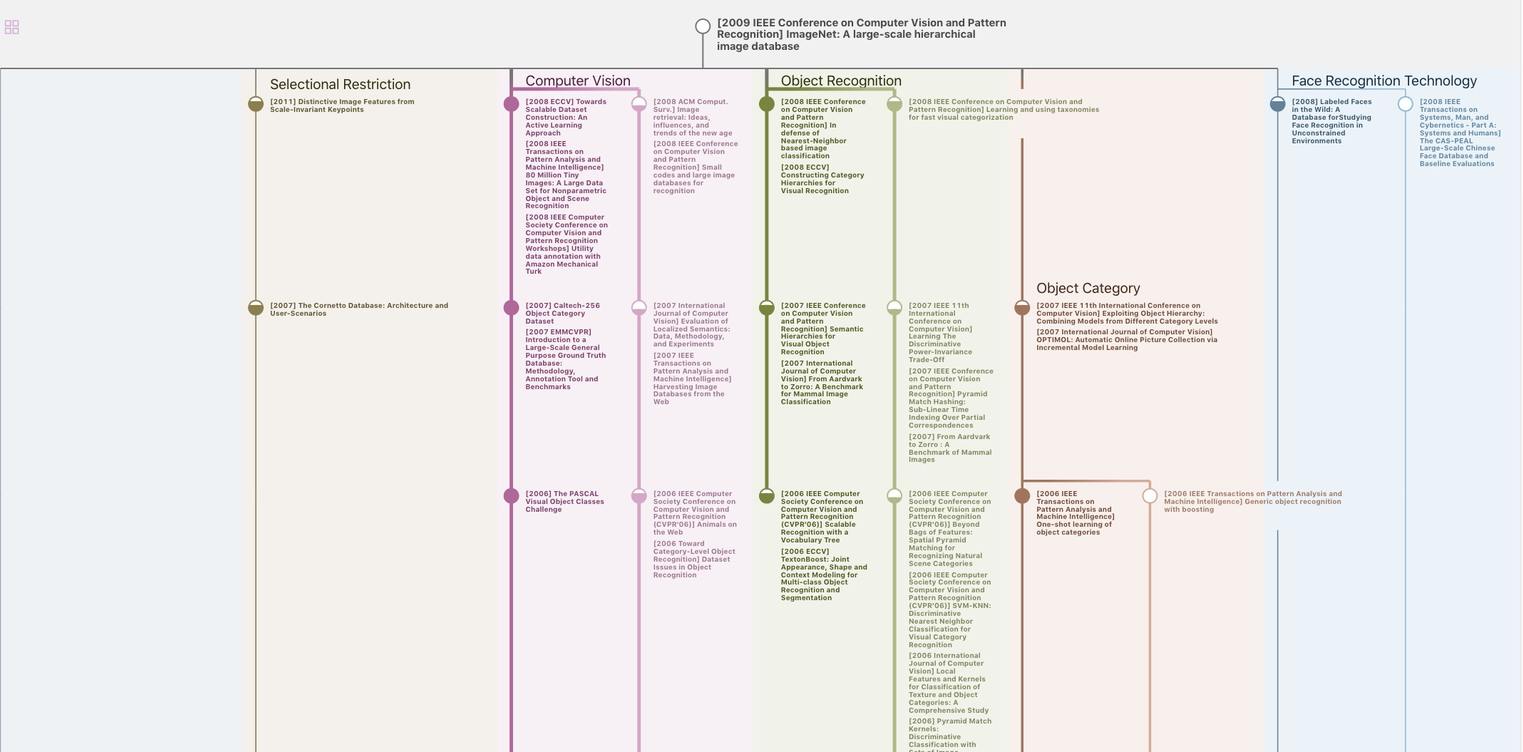
生成溯源树,研究论文发展脉络
Chat Paper
正在生成论文摘要