LGAG-Net: Lesion-Guided Adaptive Graph Network for Bone Abnormality Detection From Musculoskeletal Radiograph
IEEE ACCESS(2024)
摘要
Musculoskeletal abnormality is routinely presented in tissues and organs of the human locomotor system across the life course, and it is essential to detect musculoskeletal abnormality in X-rays. However, it is difficult to diagnose musculoskeletal abnormality from Radiographs due to the following issues: 1) There are other interfering organ tissues in the complicated backgrounds; 2)The MURA dataset contains seven different musculoskeletal radiographs, which makes general convolution neural networks unable to model the weird relationship between them. To address such problems, a Lesion-Guided Adaptive Graph Network (LGAG-Net) is proposed for bone abnormality detection from musculoskeletal radiograph, where the Lesion-guided Recurrent Feature Sampling (LRFS) module is first designed to localize the corresponding musculoskeletal abnormality regions, and then the Adaptive Graphsage Attention (AGA) module is developed to perform bone abnormality detection on the located musculoskeletal abnormality regions. Experiments on MURA dataset show that the proposed LGAG-Net can achieve an accuracy of 87.81% and Cohen Kappa statistic of 0.868, which outperforms the state-of-the-art methods, assisting the radiologists to rapidly estimate the physical development in patients.
更多查看译文
关键词
Surface defect segmentation,multi-view learning,residual attention,Gaussian normalization
AI 理解论文
溯源树
样例
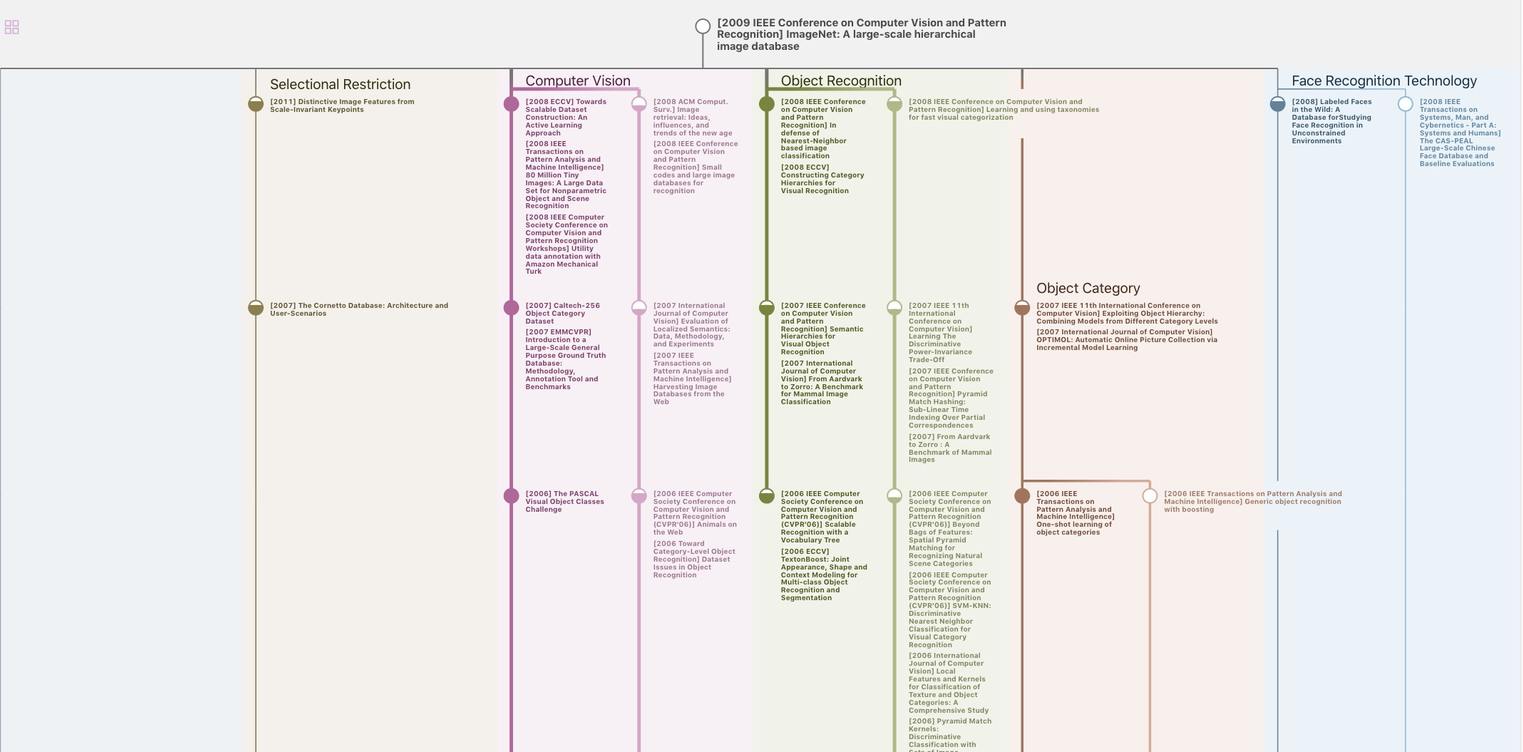
生成溯源树,研究论文发展脉络
Chat Paper
正在生成论文摘要