A Framework for Robust Deep Learning Models Against Adversarial Attacks Based on a Protection Layer Approach
IEEE ACCESS(2024)
摘要
Deep learning (DL) has demonstrated remarkable achievements in various fields. Nevertheless, DL models encounter significant challenges in detecting and defending against adversarial samples (AEs). These AEs are meticulously crafted by adversaries, introducing imperceptible perturbations to clean data to deceive DL models. Consequently, AEs pose potential risks to DL applications. In this paper, we propose an effective framework for enhancing the robustness of DL models against adversarial attacks. The framework leverages convolutional neural networks (CNNs) for feature learning, Deep Neural Networks (DNNs) with softmax for classification, and a defense mechanism to identify and exclude AEs. Evasion attacks are employed to create AEs to evade and mislead the classifier by generating malicious samples during the test phase of DL models i.e., CNN and DNN, using the Fast Gradient Sign Method (FGSM), Basic Iterative Method (BIM), Projected Gradient Descent (PGD), and Square Attack (SA). A protection layer is developed as a detection mechanism placed before the DNN classifier to identify and exclude AEs. The detection mechanism incorporates a machine learning model, which includes one of the following: Fuzzy ARTMAP, Random Forest, K-Nearest Neighbors, XGBoost, or Gradient Boosting Machine. Extensive evaluations are conducted on the MNIST, CIFAR-10, SVHN, and Fashion-MNIST data sets to assess the effectiveness of the proposed framework. The experimental results indicate the framework's ability to effectively and accurately detect AEs generated by four popular attacking methods, highlighting the potential of our developed framework in enhancing its robustness against AEs.
更多查看译文
关键词
Deep learning,Adversarial machine learning,adversarial examples,security,adversarial attacks,adversarial examples detection
AI 理解论文
溯源树
样例
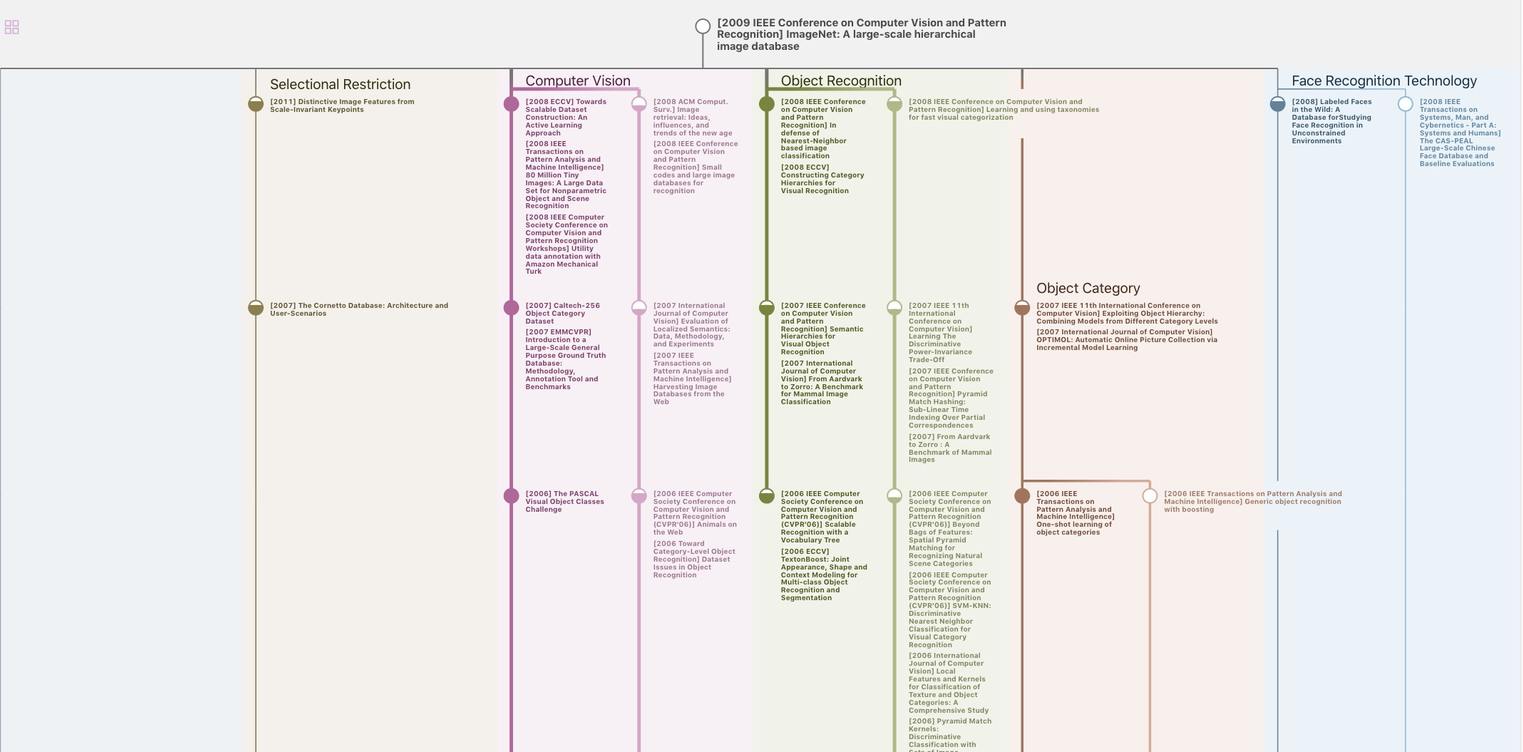
生成溯源树,研究论文发展脉络
Chat Paper
正在生成论文摘要