Enhancing Micro-Video Venue Recognition via Multi-Modal and Multi-Granularity Object Relations
IEEE Transactions on Circuits and Systems for Video Technology(2024)
摘要
Micro-video venue recognition aims to predict the venue category where a micro-video was filmed. Different from traditional long videos which contain rich temporal context, venue prediction for micro-videos is difficult due to its limited duration (generally within 6s). The existing works usually extract features of each modality from a global perspective for prediction, neglecting the semantics carried by local objects. To this end, we propose Multi-Modal and Multi-Granularity Object Relations (M
2
ORE) to address the above issues, which learns multi-granularity interactive semantics between venues and multimodal semantic objects to help understand venues. Specifically, M
2
ORE comprises of two modules: it first extract semantic objects of different modalities, i.e. visual objects in keyframes and keywords in texts, and models the affiliation relationship between semantic objects and venues and the co-occurrence relationship among semantic objects, forming a heterogeneous venue-object relation graph. Then, to achieve the interactive semantics between venues and objects from the relation graph, a novel Parallel-Graph Inference Model (Parallel-GIM) is proposed, which updates the representation of nodes through graph propagation and fuse multi-level features (local-global-multimodal) through the devised hierarchical attention mechanism. Finally, the probability distribution of venues can be obtained through a multi-layer perceptron with the comprehensive features of the venue nodes. Extensive experiments on real-world micro-video dataset demonstrate the superiority of the proposed M
2
ORE.
更多查看译文
关键词
Micro-video venue recognition,Graph neural network,Attention mechanism,Multi-modal fusion,Online social network
AI 理解论文
溯源树
样例
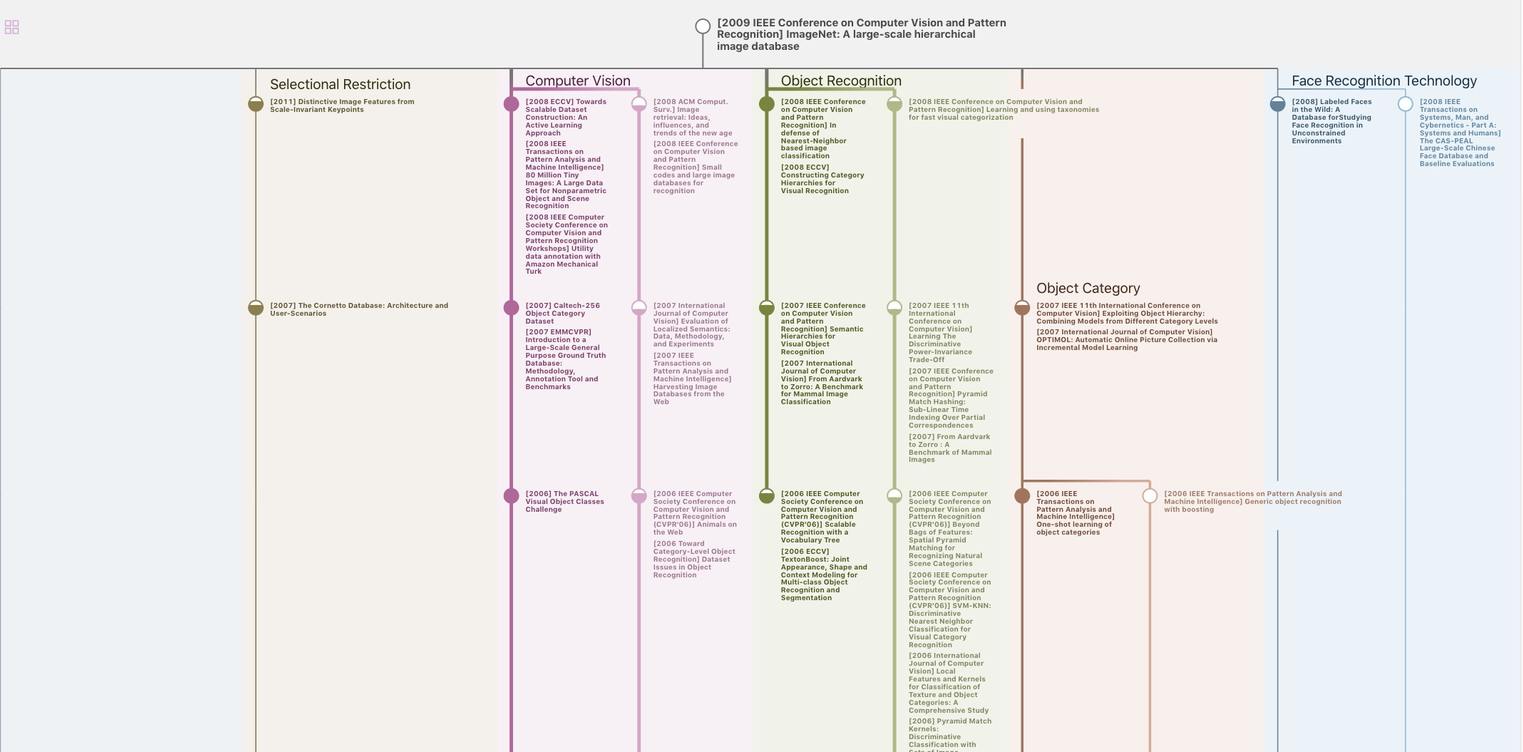
生成溯源树,研究论文发展脉络
Chat Paper
正在生成论文摘要