quantile-Long Short Term Memory: A Robust, Time Series Anomaly Detection Method
IEEE Transactions on Artificial Intelligence(2024)
摘要
Anomalies refer to the departure of systems and devices from their normal behaviour in standard operating conditions. An anomaly in an industrial device can indicate an upcoming failure, often in the temporal direction. In this paper, we make two contributions: 1) we estimate conditional quantiles in the popular Long Short Term Memory networks (LSTM) architecture, propose a novel anomaly detection method, qLSTM, and consider three different ways to define anomalies based on the estimated quantiles. 2) we use a new learnable activation function (AF), Parametric Elliot Function (PEF), in qLSTM architecture to model temporal long-range dependency. Unlike
sigmoid
and
tanh
, the derivative of the PEF depends on the input as well as on the parameter, which help in mitigating the vanishing gradient problem and therefore facilitates in escaping early saturation. The proposed algorithms are compared with other well-known anomaly detection algorithms, such as Isolation Forest (iForest), Elliptic Envelope, Autoencoder, and modern Deep Learning models such as Deep Autoencoding Gaussian Mixture Model (DAGMM), Generative Adversarial Networks (GAN). The algorithms are evaluated using various performance metrics, such as Precision and Recall. The algorithms have been tested on multiple industrial time-series datasets such as Yahoo, AWS, GE, and machine sensors. We have found that the LSTM-based quantile algorithms are very effective and outperformed the existing algorithms in identifying anomalies.
更多查看译文
关键词
Anomaly,LSTM,Quantile,Parametric Activation
AI 理解论文
溯源树
样例
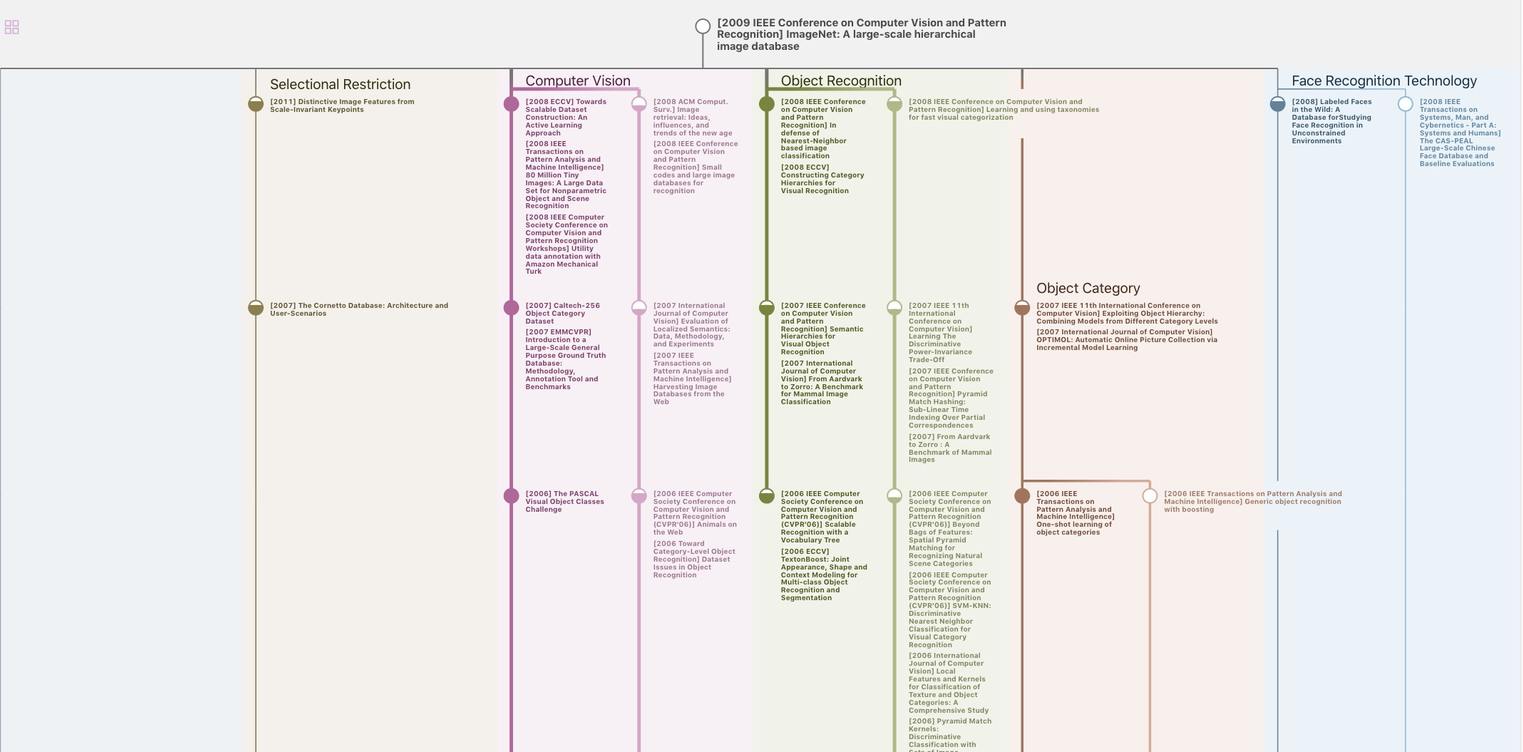
生成溯源树,研究论文发展脉络
Chat Paper
正在生成论文摘要