Learning-Driven Dynamic Multimodal Optimization Algorithm for Real-Time Traceability of Water Pollution
IEEE Transactions on Artificial Intelligence(2024)
摘要
In recent years, water quality safety problems caused by sudden urban drinking water contamination events have attracted the attention of experts in China and abroad. After an occurrence of urban water pollution, it is challenging to locate the pollution source in real time according to the information collected by water quality sensors and then quickly deduce the injection location, injection concentration quality, and other characteristics of the pollution source. In this paper, we propose a learning-driven dynamic multimodal optimization algorithm framework that combines various machine learning algorithms. First, it uses the support vector machine (SVM) model to scale down and perform node probability estimation for a large-scale water supply pipeline network. Second, by predicting the uncertainty parameters of the pipe network when setting the pipe network simulation parameters, the framework can narrow the gap between simulation and real conditions, giving the pollution source characteristics obtained by the algorithm solution a higher confidence level. The experimental results show that the algorithm framework can achieve real-time traceability of water pollution for large-scale, uncertain pipe network environments and can obtain better accuracy and real-time performance than other dynamic algorithms.
更多查看译文
关键词
dynamic multimodal optimization algorithm,learning-driven,real-time water pollution traceability,support vector machine (SVM),urban water pollution
AI 理解论文
溯源树
样例
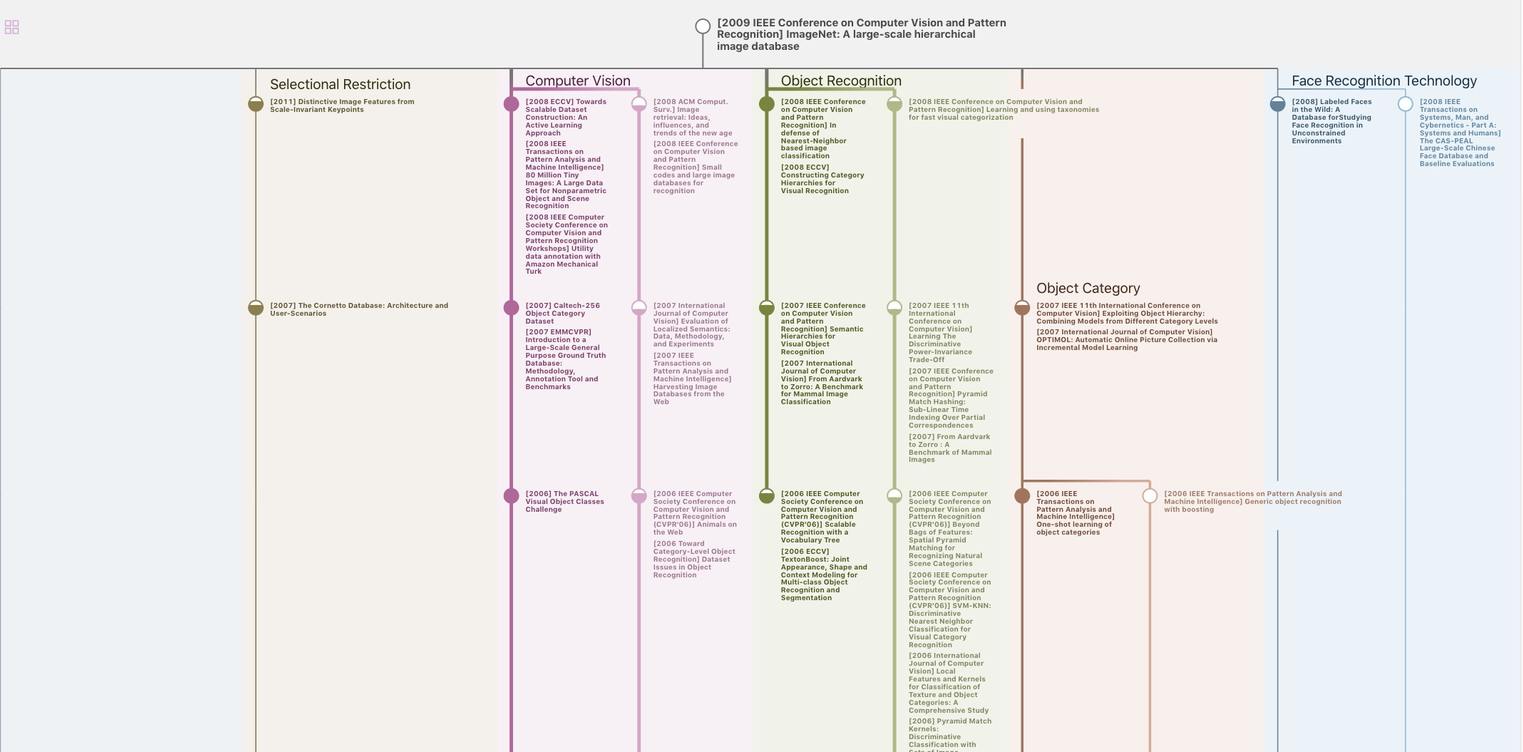
生成溯源树,研究论文发展脉络
Chat Paper
正在生成论文摘要