A general dual-pathway network for EEG denoising
FRONTIERS IN NEUROSCIENCE(2024)
摘要
Introduction Scalp electroencephalogram (EEG) analysis and interpretation are crucial for tracking and analyzing brain activity. The collected scalp EEG signals, however, are weak and frequently tainted with various sorts of artifacts. The models based on deep learning provide comparable performance with that of traditional techniques. However, current deep learning networks applied to scalp EEG noise reduction are large in scale and suffer from overfitting.Methods Here, we propose a dual-pathway autoencoder modeling framework named DPAE for scalp EEG signal denoising and demonstrate the superiority of the model on multi-layer perceptron (MLP), convolutional neural network (CNN) and recurrent neural network (RNN), respectively. We validate the denoising performance on benchmark scalp EEG artifact datasets.Results The experimental results show that our model architecture not only significantly reduces the computational effort but also outperforms existing deep learning denoising algorithms in root relative mean square error (RRMSE)metrics, both in the time and frequency domains.Discussion The DPAE architecture does not require a priori knowledge of the noise distribution nor is it limited by the network layer structure, which is a general network model oriented toward blind source separation.
更多查看译文
关键词
EEG denoising,dual-pathway structure,general network model,light weight autoencoder,blind source separation
AI 理解论文
溯源树
样例
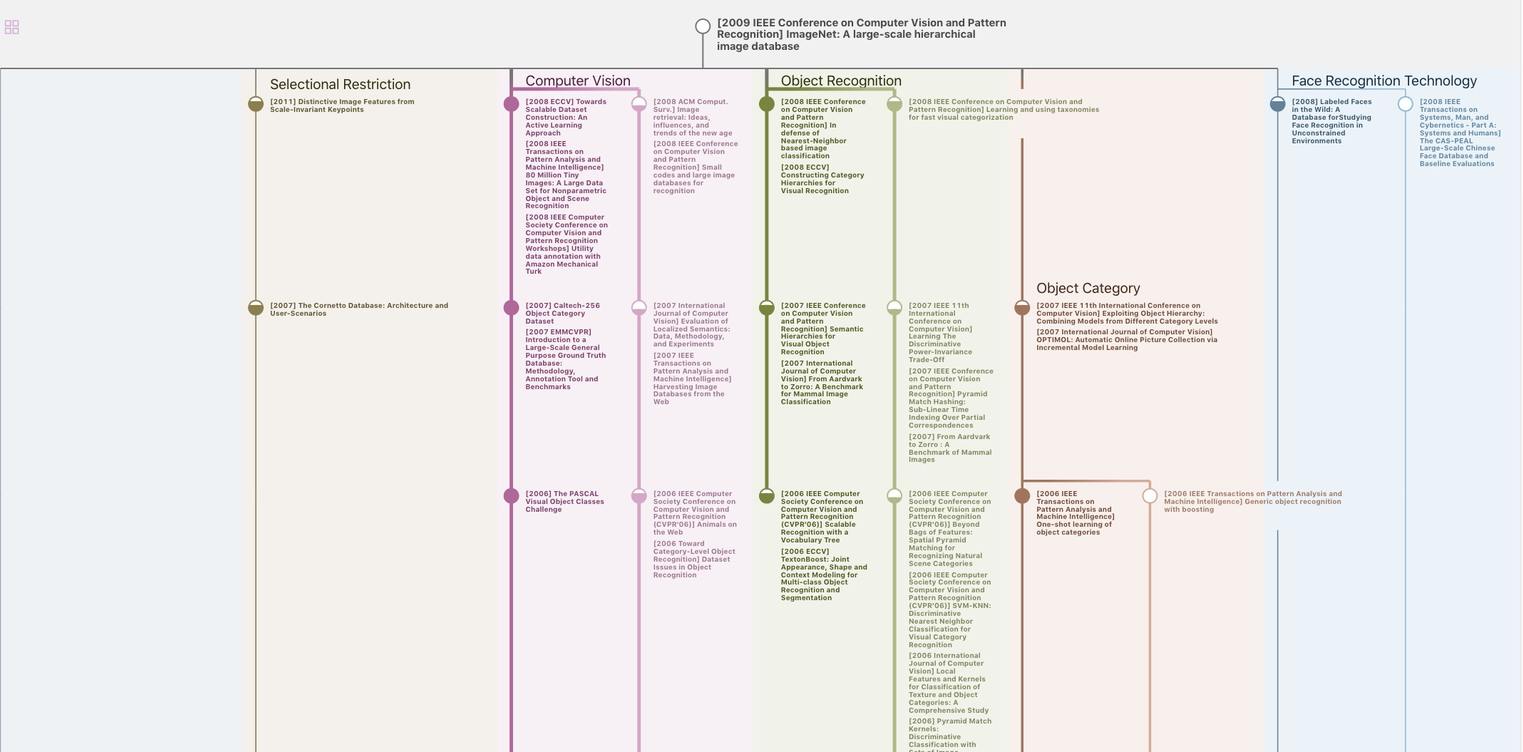
生成溯源树,研究论文发展脉络
Chat Paper
正在生成论文摘要