SiamBRF: Siamese Broad-Spectrum Relevance Fusion Network for Aerial Tracking
IEEE GEOSCIENCE AND REMOTE SENSING LETTERS(2024)
摘要
Tracking in UAV scenarios is a complex and important task. Different from target tracking in general scenarios, due to the unique characteristics of the UAV view, the UAV tracking task is faced with many very challenging scenarios such as viewpoint changes and aerial view. Although the Siamese series of trackers have achieved good performance in general scenes, the existing methods of enhancing local features at certain levels may be effective for general scene tracking, but the lack of broad-spectrum relevance will lead to degradation of accuracy, especially in aerial tracking scenarios. In this letter, we propose a broad-spectrum relevance fusion model (SiamBRF) in the Siamese-based tracking framework to efficiently obtain relevance at broad-spectrum levels to enhance the feature representation, thereby enabling our tracker to be more suitable for solving UAV-tracking tasks. Specifically, a coordinate contextual encoder (CCE) is designed to update the saliency representations, and then the transformer cascaded fusion (TCF) structure is used to realize the interbranch information exchange and integration, which could precisely distinguish the target and background in the UAV-tracking scenarios. Finally, we design a spatiotemporal dependency fusion (SDF) network for deep features to better balance the semantic and spatial information. Several experiments demonstrate that our SiamBRF achieves around 3% performance improvement in precision and success rate over the mainstream Siamese-based trackers.
更多查看译文
关键词
Target tracking,Task analysis,Transformers,Semantics,Remote sensing,Spatiotemporal phenomena,Optimization,Aerial tracking,broad-spectrum relevance,feature fusion,remote sensing
AI 理解论文
溯源树
样例
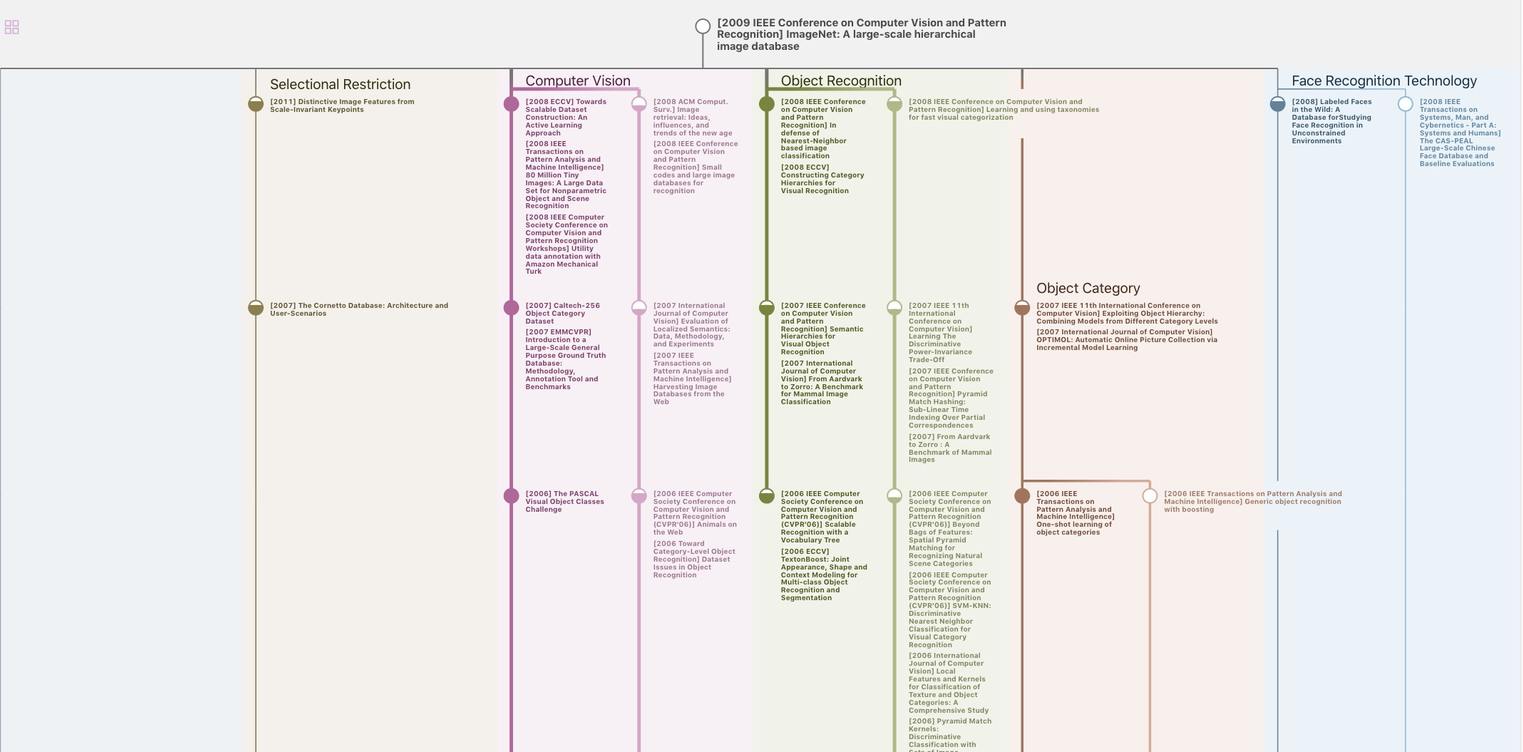
生成溯源树,研究论文发展脉络
Chat Paper
正在生成论文摘要