Adjacent Self-Similarity 3-D Convolution for Multimodal Image Registration
IEEE GEOSCIENCE AND REMOTE SENSING LETTERS(2024)
摘要
Significant challenges exist in the registration of multimodal images (MMIs) due to nonlinear radiation differences, variations in lighting, and interference from image noise. These issues often lead to unreliable similarity measurements and low accuracy in point matching during multimodal registration. To address these challenges, this letter introduces a novel MMI registration method based on adjacent self-similarity 3-D convolution (ASTC). The proposed method consists of three main steps: feature point extraction, where key points are uniformly extracted via the block-FAST method; ASTC salient feature construction, where a local adjacent self-similarity (ASS) model is employed to create multidimensional features; and feature structure enhancement, where a 3-D convolution is used for feature enhancement and finishing the process of image feature description. This letter evaluates the ASTC method against six sets of representative MMIs and compares it with six other algorithms. The results demonstrate that: 1) the ASTC algorithm effectively overcomes radiation distortion, intensity differences, and lighting differences in MMIs, leading to improved accuracy in point matching; and 2) the ASTC algorithm achieves higher matching efficiency and reduces time consumption, making it a practical choice for various data types. In summary, the proposed ASTC algorithm offers a robust solution for reliable registration of MMIs, addressing common challenges related to image differences and improving the overall accuracy of the process. The experimental data and code link used in this letter can be found at https://github.com/yangwill81/ASTC.
更多查看译文
关键词
Resistance,3-D convolution,adjacent self-similarity (ASS) features,multimodal images (MMIs),similarity measures,template matching
AI 理解论文
溯源树
样例
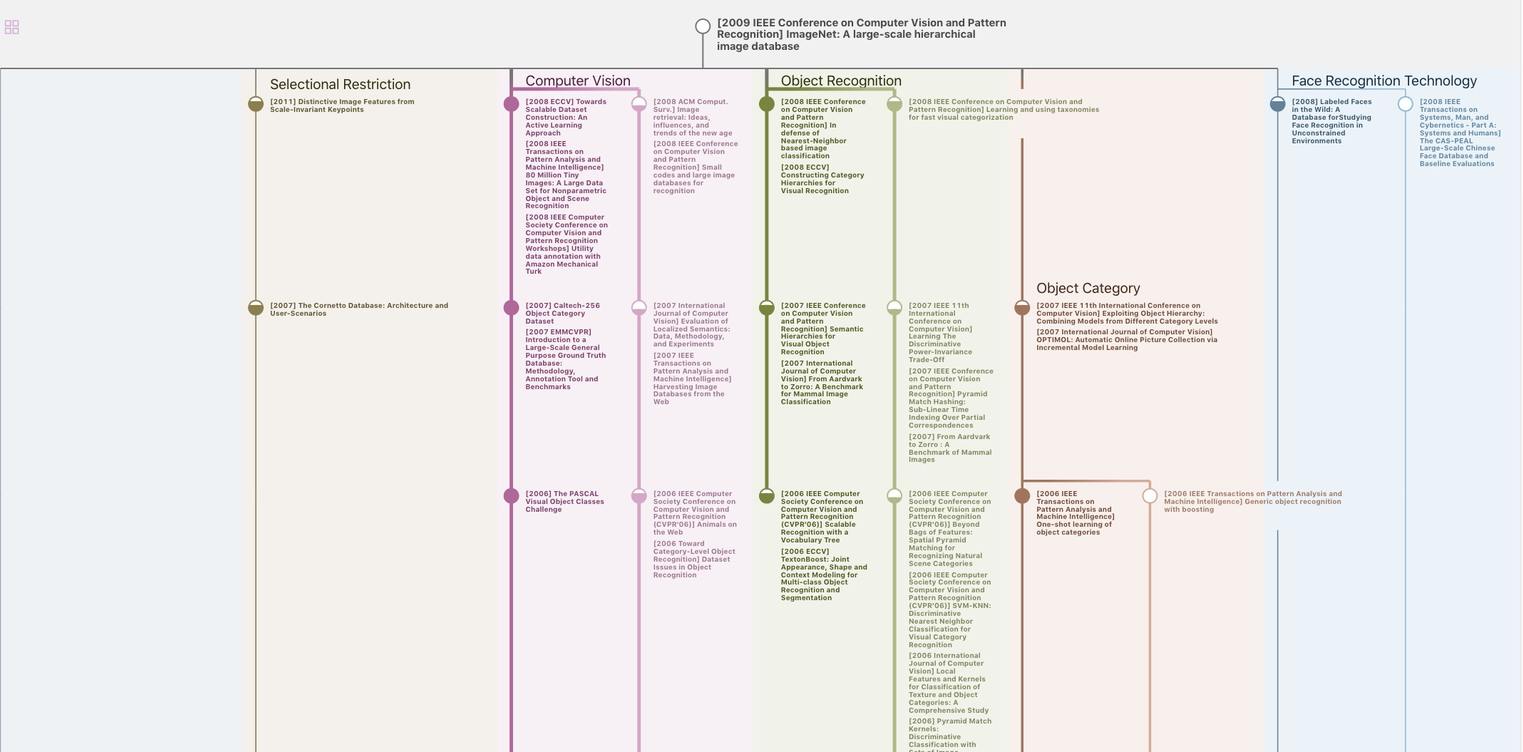
生成溯源树,研究论文发展脉络
Chat Paper
正在生成论文摘要