Machine Learning-Optimized Compact Frequency Reconfigurable Antenna With RSSI Enhancement for Long-Range Applications
IEEE ACCESS(2024)
摘要
This study presents an innovative and compact monopole antenna with dual-band frequency reconfigurability for LoRa applications. It operates within the 915 MHz and 868 MHz frequencies, aligning with the designated bands for use in America, Asia and Europe. No existing compact reconfigurable antenna with these features for LoRa applications within ISM bands below 1 GHz is known. Employing an economical FR-4 substrate in its design, the antenna attains a compact size of 40 x 42 mm(2) (0.12 lambda(0) x 0.12 lambda(0)), where lambda(0) denotes the wavelength in free space corresponding to 868 MHz. A single RF PIN diode enables seamless switching between 868 MHz and 915 MHz bands. Design, simulation, and optimization employed CST MWS (R) software. Supervised regression Machine Learning (ML) models predicted resonance frequencies, with Gaussian Process Regression emerging as optimal, achieving R-squared and variance scores of 92.87% and 93.77%, respectively. A maximum gain of 2 dBi at 915 MHz and 70% efficiency, boasting good radiation patterns and matching was demonstrated by the antenna. Experimental validation in a football field at Universiti Teknologi PETRONAS, Malaysia, assessed the proposed antenna's performance on a LoRa transceiver system based on LoRa SX1276. The Received Signal Strength Indicator (RSSI) of the proposed antenna consistently exceeded the conventional commercially available monopole antenna by an average of -12 dBm at every point up to 300 m, showcasing enhanced signal reception. The antenna proves promising for wireless sensor nodes in long-range applications.
更多查看译文
关键词
Dual-band,LoRa,PIN-diode,reconfigurable,RSSI
AI 理解论文
溯源树
样例
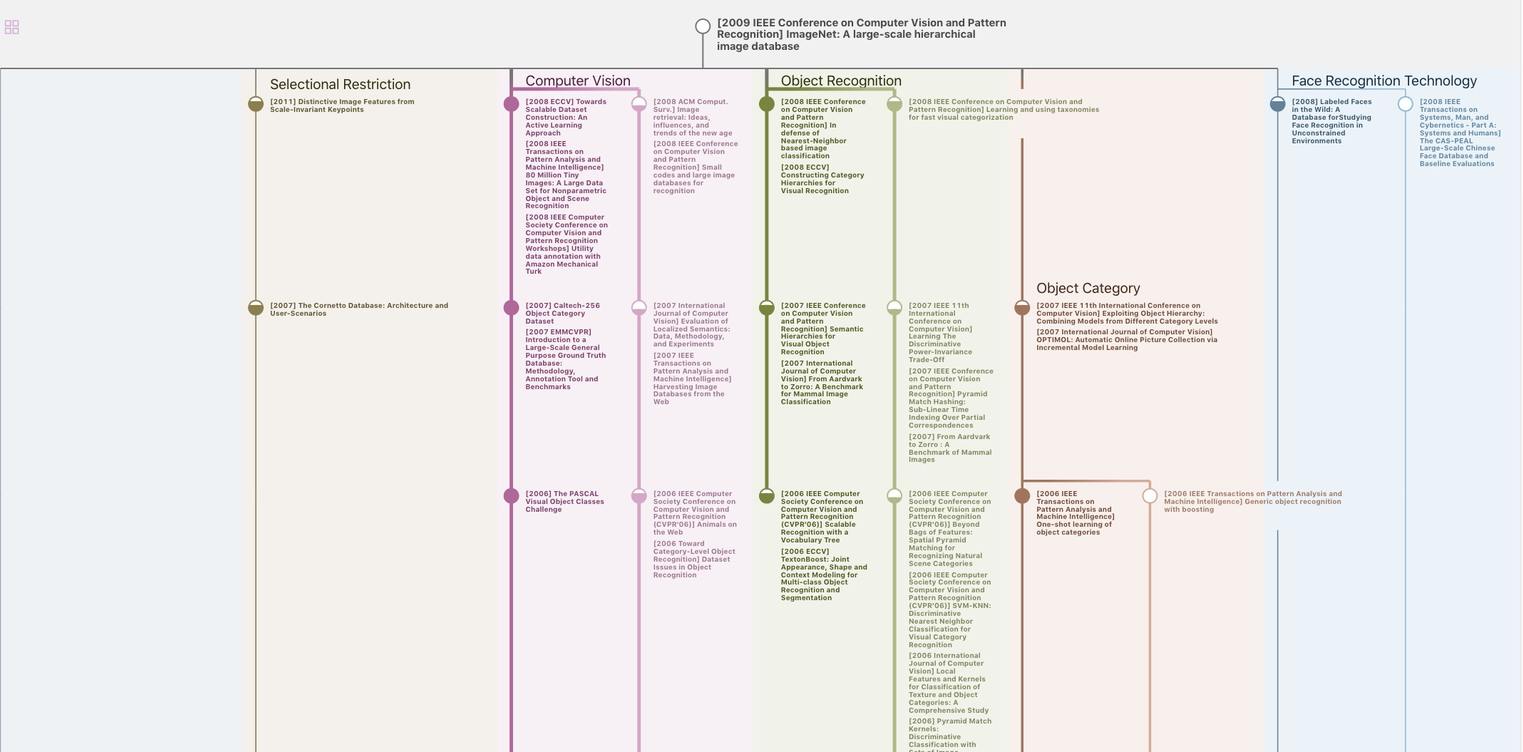
生成溯源树,研究论文发展脉络
Chat Paper
正在生成论文摘要