Binary structured physics-informed neural networks for solving equations with rapidly changing solutions
CoRR(2024)
摘要
Physics-informed neural networks (PINNs), rooted in deep learning, have emerged as a promising approach for solving partial differential equations (PDEs). By embedding the physical information described by PDEs into feedforward neural networks, PINNs are trained as surrogate models to approximate solutions without the need for label data. Nevertheless, even though PINNs have shown remarkable performance, they can face difficulties, especially when dealing with equations featuring rapidly changing solutions. These difficulties encompass slow convergence, susceptibility to becoming trapped in local minima, and reduced solution accuracy. To address these issues, we propose a binary structured physics-informed neural network (BsPINN) framework, which employs binary structured neural network (BsNN) as the neural network component. By leveraging a binary structure that reduces inter-neuron connections compared to fully connected neural networks, BsPINNs excel in capturing the local features of solutions more effectively and efficiently. These features are particularly crucial for learning the rapidly changing in the nature of solutions. In a series of numerical experiments solving Burgers equation, Euler equation, Helmholtz equation, and high-dimension Poisson equation, BsPINNs exhibit superior convergence speed and heightened accuracy compared to PINNs. From these experiments, we discover that BsPINNs resolve the issues caused by increased hidden layers in PINNs resulting in over-smoothing, and prevent the decline in accuracy due to non-smoothness of PDEs solutions.
更多查看译文
AI 理解论文
溯源树
样例
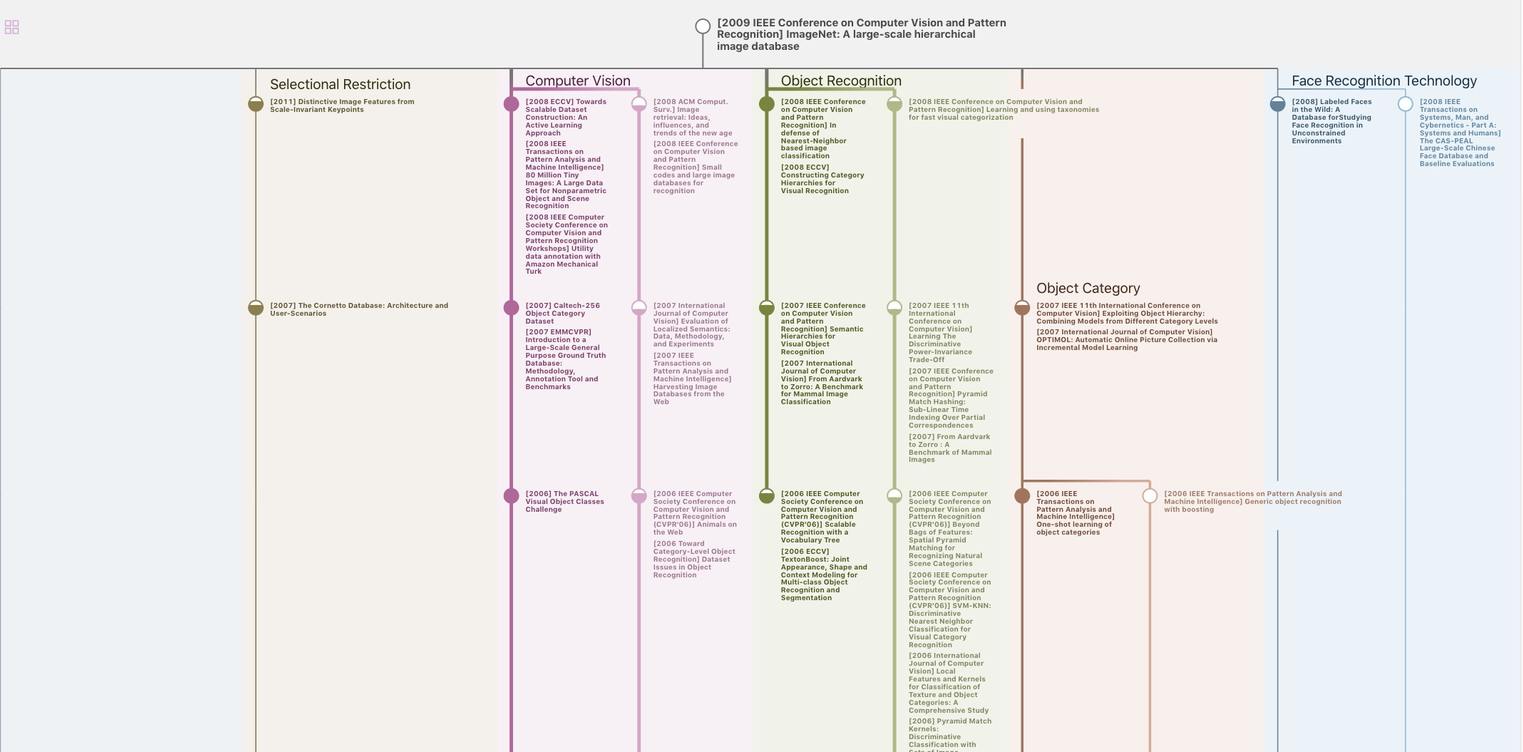
生成溯源树,研究论文发展脉络
Chat Paper
正在生成论文摘要