Multi-Sources Information Fusion Learning for Multi-Points NLOS Localization
arxiv(2024)
摘要
Accurate localization of mobile terminals is a pivotal aspect of integrated
sensing and communication systems. Traditional fingerprint localization
methods, which infer coordinates from channel information within pre-defined
rectangular areas, often face challenges due to the heterogeneous distribution
of fingerprints inherent in non-line-of-sight (NLOS) scenarios. To overcome
this limitation, we have developed a novel multi-source information fusion
learning framework referred to as the Autosync Multi-Domain NLOS Localization
(AMDNLoc). Specifically, AMDNLoc employs a two-stage matched filter fused with
a target tracking algorithm and iterative centroid-based clustering to
automatically and irregularly segment NLOS regions, ensuring uniform
fingerprint distribution within channel state information across frequency,
power, and time-delay domains. Additionally, the framework utilizes a
segment-specific linear classifier array, coupled with deep residual
network-based feature extraction and fusion, to establish the correlation
function between fingerprint features and coordinates within these regions.
Simulation results demonstrate that AMDNLoc achieves an impressive accuracy of
1.46 meters on typical wireless artificial intelligence research datasets and
offers interpretability, adaptability, and scalability in various scenarios.
更多查看译文
AI 理解论文
溯源树
样例
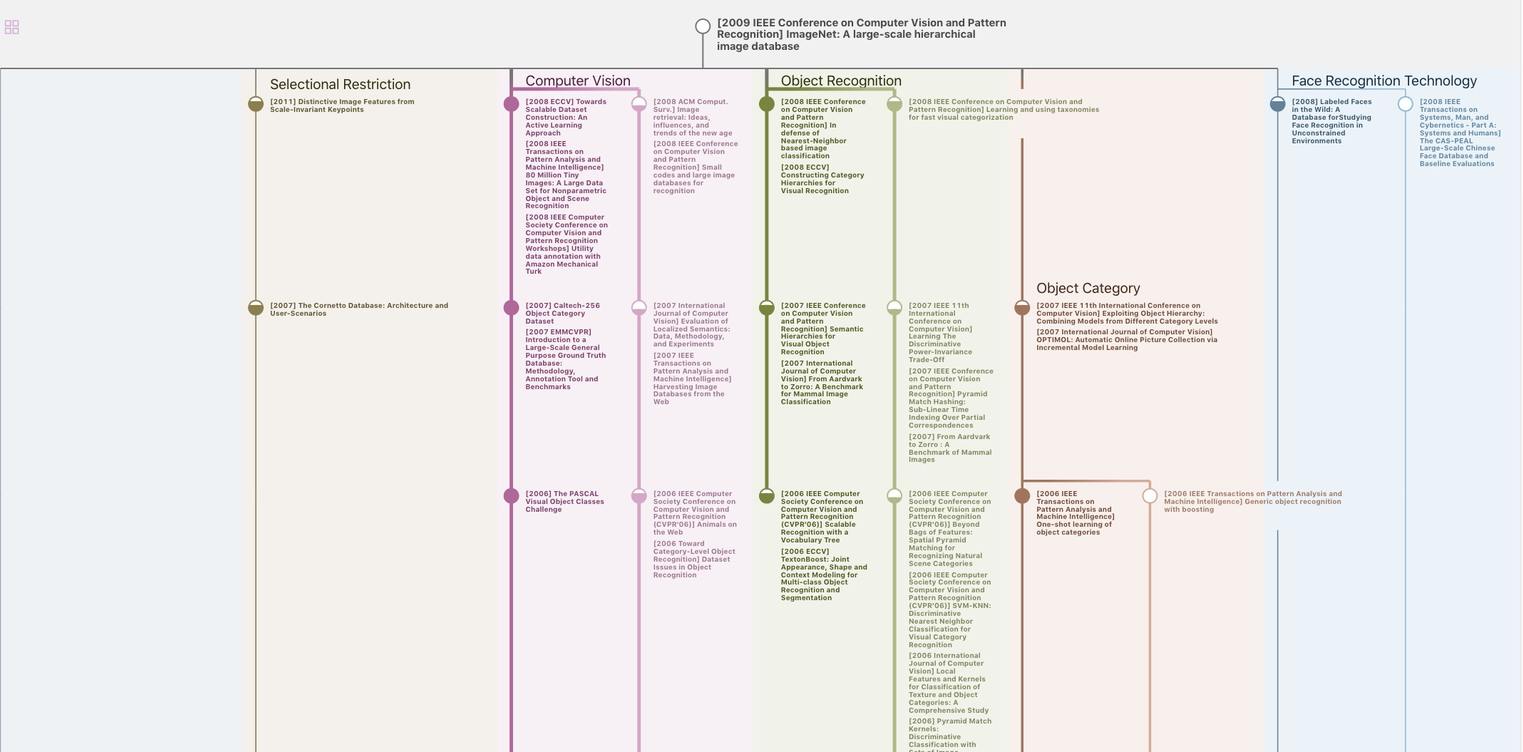
生成溯源树,研究论文发展脉络
Chat Paper
正在生成论文摘要